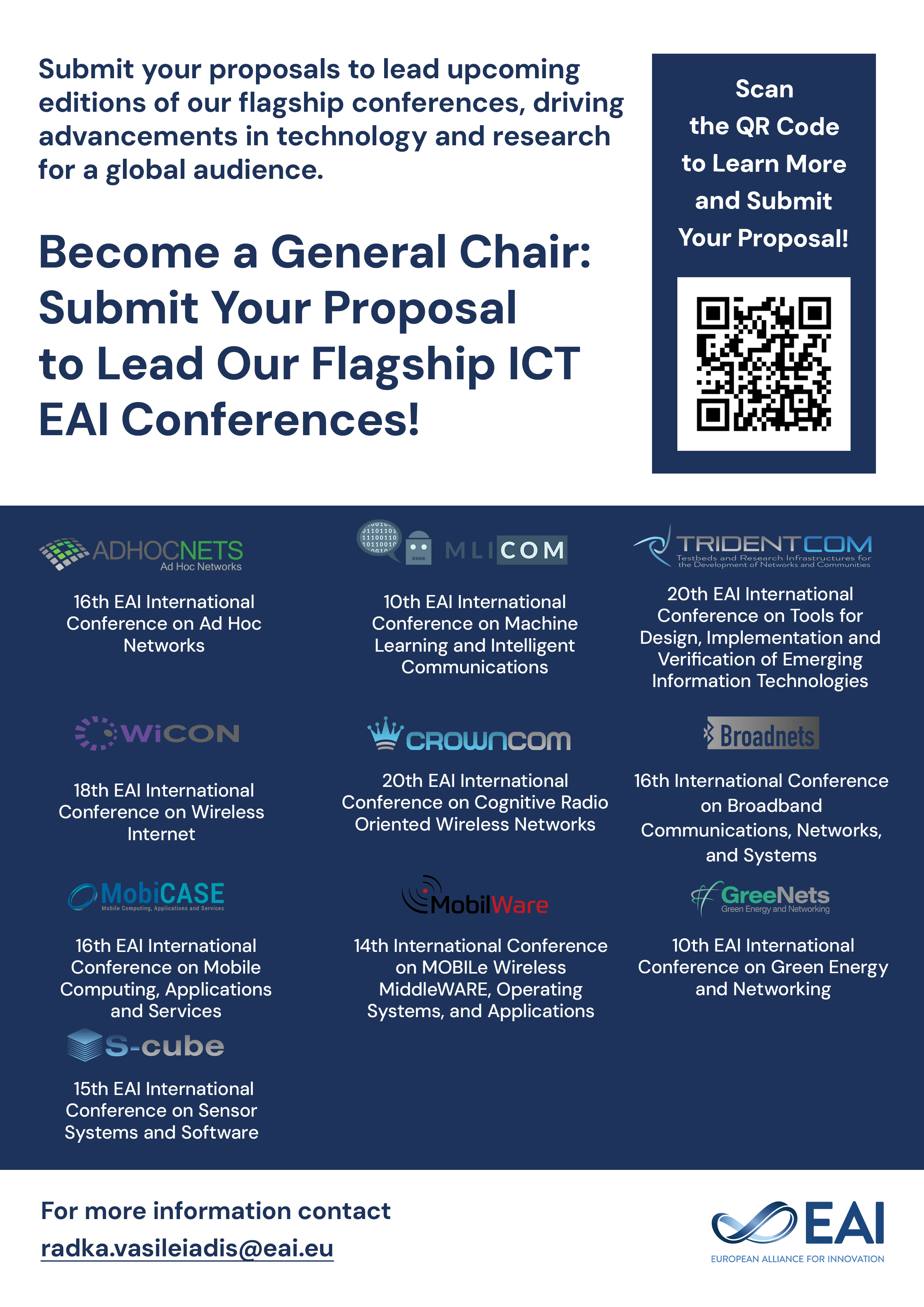
Research Article
Self-similar Traffic Prediction Scheme Based on Wavelet Transform for Satellite Internet Services
@INPROCEEDINGS{10.1007/978-3-319-52730-7_19, author={Yu Han and Dezhi Li and Qing Guo and Zhenyong Wang and Deyang Kong}, title={Self-similar Traffic Prediction Scheme Based on Wavelet Transform for Satellite Internet Services}, proceedings={Machine Learning and Intelligent Communications. First International Conference, MLICOM 2016, Shanghai, China, August 27-28, 2016, Revised Selected Papers}, proceedings_a={MLICOM}, year={2017}, month={2}, keywords={Satellite internet Self-similar traffic prediction Wavelet transform ARIMA model}, doi={10.1007/978-3-319-52730-7_19} }
- Yu Han
Dezhi Li
Qing Guo
Zhenyong Wang
Deyang Kong
Year: 2017
Self-similar Traffic Prediction Scheme Based on Wavelet Transform for Satellite Internet Services
MLICOM
Springer
DOI: 10.1007/978-3-319-52730-7_19
Abstract
With service types and requirements of broadband satellite internet continuously increasing, improving QoS (Quality of service) of satellite internet has attracted extensive attention. To reduce the impact of self-similarity caused by various of service traffic sources converge on satellite communication system, we propose a novel model from the perspective of self-similar traffic prediction. Combinating wavelet transform and ARIMA (Autoregressive Integrated Moving Average) model to predict self-similar traffic of satellite internet is proposed. The optimal model to the problem is presented. The number selection of prediction samples and the impact of prediction steps on the accuracy of the prediction system are discussed, and the parameters are addressed. Simulation results show ARIMA model can achieve a better prediction effect with a combination of wavelet transform than that of the traditional autoregressive model not utilizing wavelet technology.