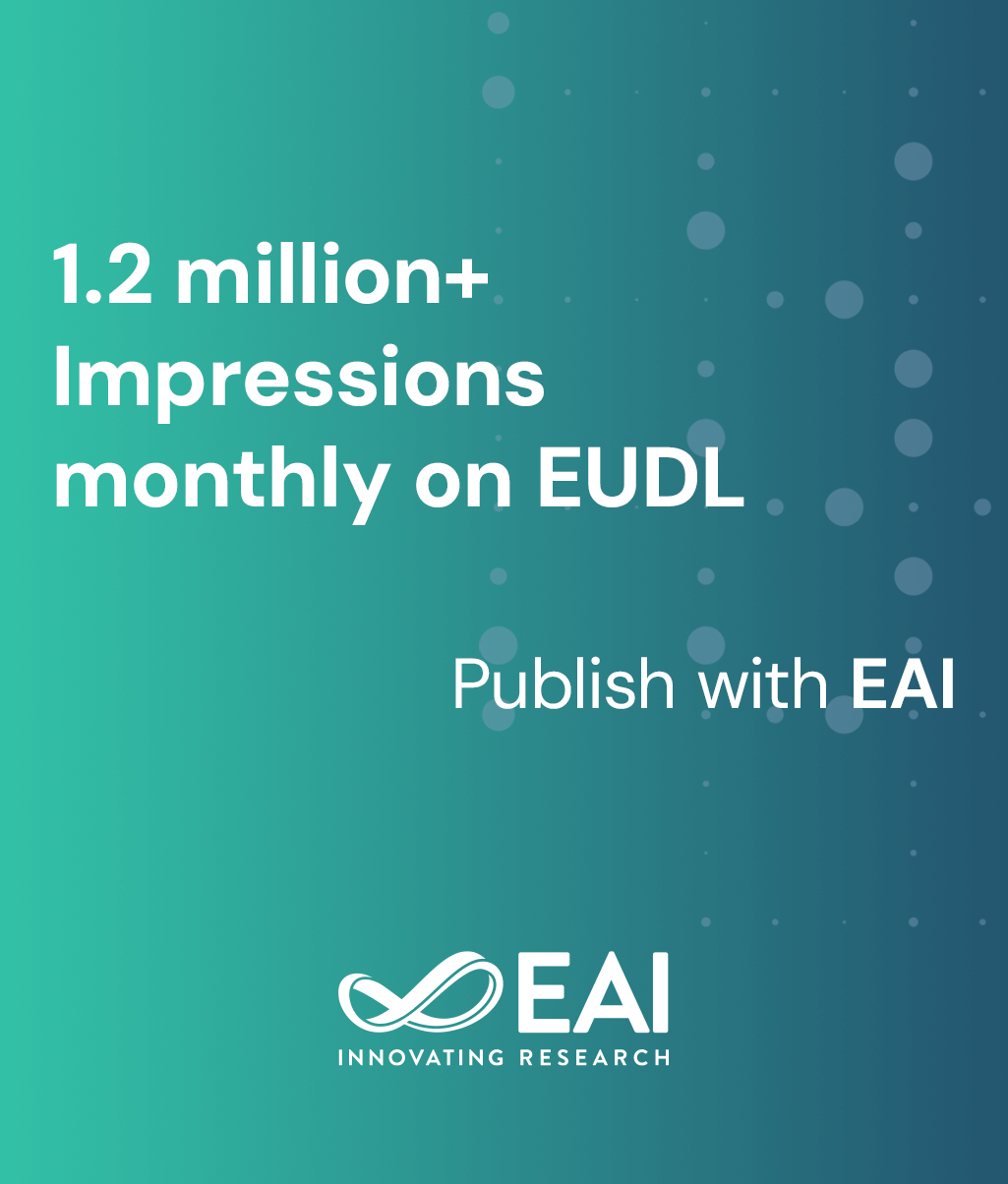
Research Article
Inertial Sensor Based Modelling of Human Activity Classes: Feature Extraction and Multi-sensor Data Fusion Using Machine Learning Algorithms
@INPROCEEDINGS{10.1007/978-3-319-49655-9_38, author={Tahmina Zebin and Patricia Scully and Krikor Ozanyan}, title={Inertial Sensor Based Modelling of Human Activity Classes: Feature Extraction and Multi-sensor Data Fusion Using Machine Learning Algorithms}, proceedings={eHealth 360°. International Summit on eHealth, Budapest, Hungary, June 14-16, 2016, Revised Selected Papers}, proceedings_a={EHEALTH360}, year={2017}, month={1}, keywords={Inertial measurement unit Accelerometer data Feature extraction Data-fusion Machine learning algorithms Human Activity Recognition}, doi={10.1007/978-3-319-49655-9_38} }
- Tahmina Zebin
Patricia Scully
Krikor Ozanyan
Year: 2017
Inertial Sensor Based Modelling of Human Activity Classes: Feature Extraction and Multi-sensor Data Fusion Using Machine Learning Algorithms
EHEALTH360
Springer
DOI: 10.1007/978-3-319-49655-9_38
Abstract
Wearable inertial sensors are currently receiving pronounced interest due to applications in unconstrained daily life settings, ambulatory monitoring and pervasive computing systems. This research focuses on human activity recognition problem, in which inputs are multichannel time series signals acquired from a set of body-worn inertial sensors and outputs are automatically classified human activities. A general-purpose framework has been presented for designing and evaluating activity recognition system with six different activities using machine learning algorithms such as support vector machine (SVM) and artificial neural networks (ANN). Several feature selection methods were explored to make the recognition process faster by experimenting on the features extracted from the accelerometer and gyroscope time series data collected from a number of volunteers. In addition, a detailed discussion is presented to explore how different design parameters, for example, the number of features and data fusion from multiple sensor locations - impact on overall recognition performance.