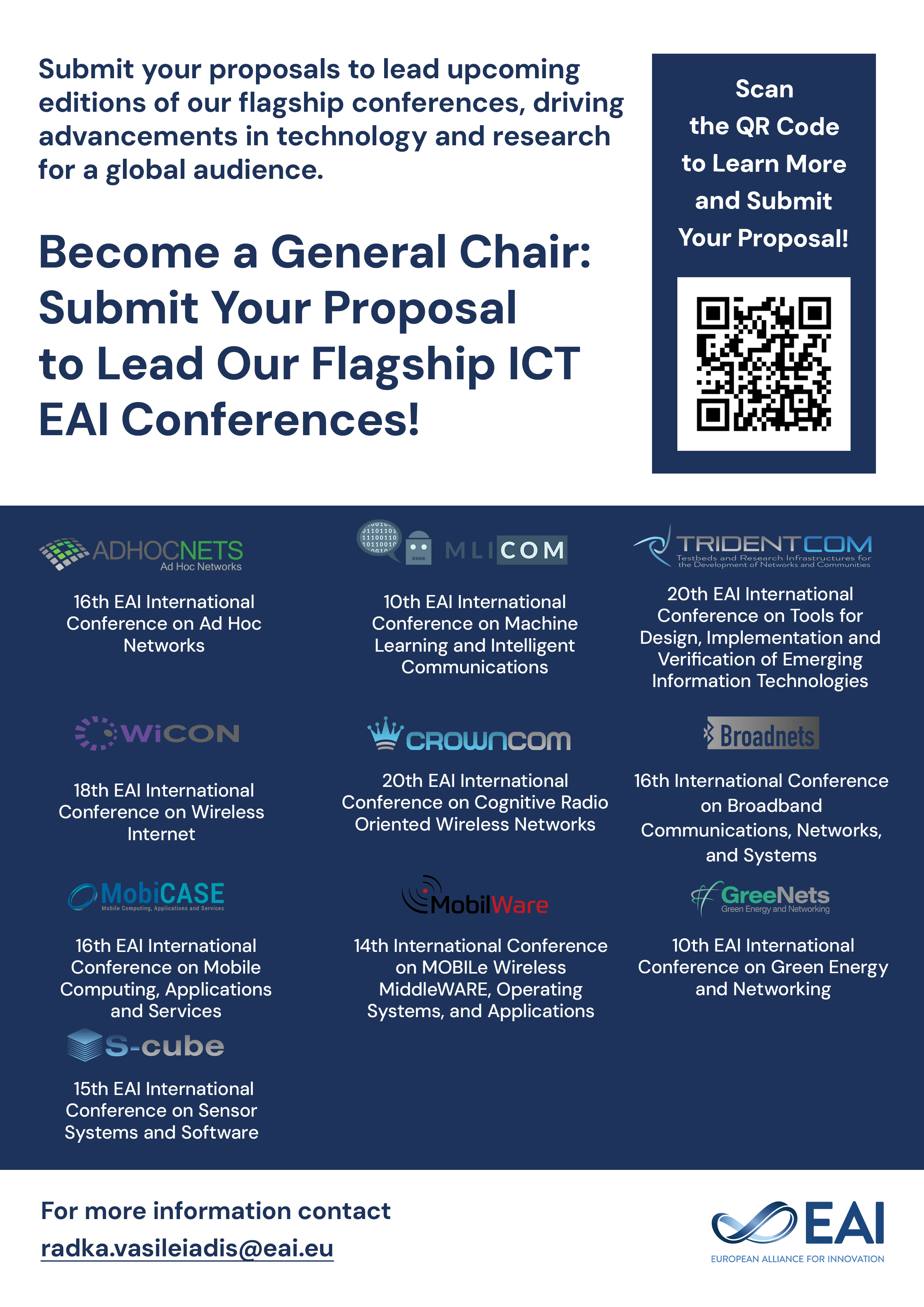
Research Article
Towards Stress Detection in Real-Life Scenarios Using Wearable Sensors: Normalization Factor to Reduce Variability in Stress Physiology
@INPROCEEDINGS{10.1007/978-3-319-49655-9_34, author={Bishal Lamichhane and Ulf Gro\`{a}ekath\o{}fer and Giuseppina Schiavone and Pierluigi Casale}, title={Towards Stress Detection in Real-Life Scenarios Using Wearable Sensors: Normalization Factor to Reduce Variability in Stress Physiology}, proceedings={eHealth 360°. International Summit on eHealth, Budapest, Hungary, June 14-16, 2016, Revised Selected Papers}, proceedings_a={EHEALTH360}, year={2017}, month={1}, keywords={Stress detection Wearable sensors Physiology normalization Machine learning}, doi={10.1007/978-3-319-49655-9_34} }
- Bishal Lamichhane
Ulf Großekathöfer
Giuseppina Schiavone
Pierluigi Casale
Year: 2017
Towards Stress Detection in Real-Life Scenarios Using Wearable Sensors: Normalization Factor to Reduce Variability in Stress Physiology
EHEALTH360
Springer
DOI: 10.1007/978-3-319-49655-9_34
Abstract
Wearable physiological sensors offer possibilities for the development of continuous stress detection models. Such models need to address the inter-individual and intra-individual differences in stress physiology. In this paper we propose and evaluate a normalization factor, , to address such differences. is computed using physiological features and the corresponding stress level at a reference point. The proposed normalization factor is evaluated in a dataset obtained from a free-living study with 10 participants, where each participant was monitored for 5 days during their working hours using different physiological sensors. We obtain an average reduction of mean squared error by up to 32% in models with compared to the models without .