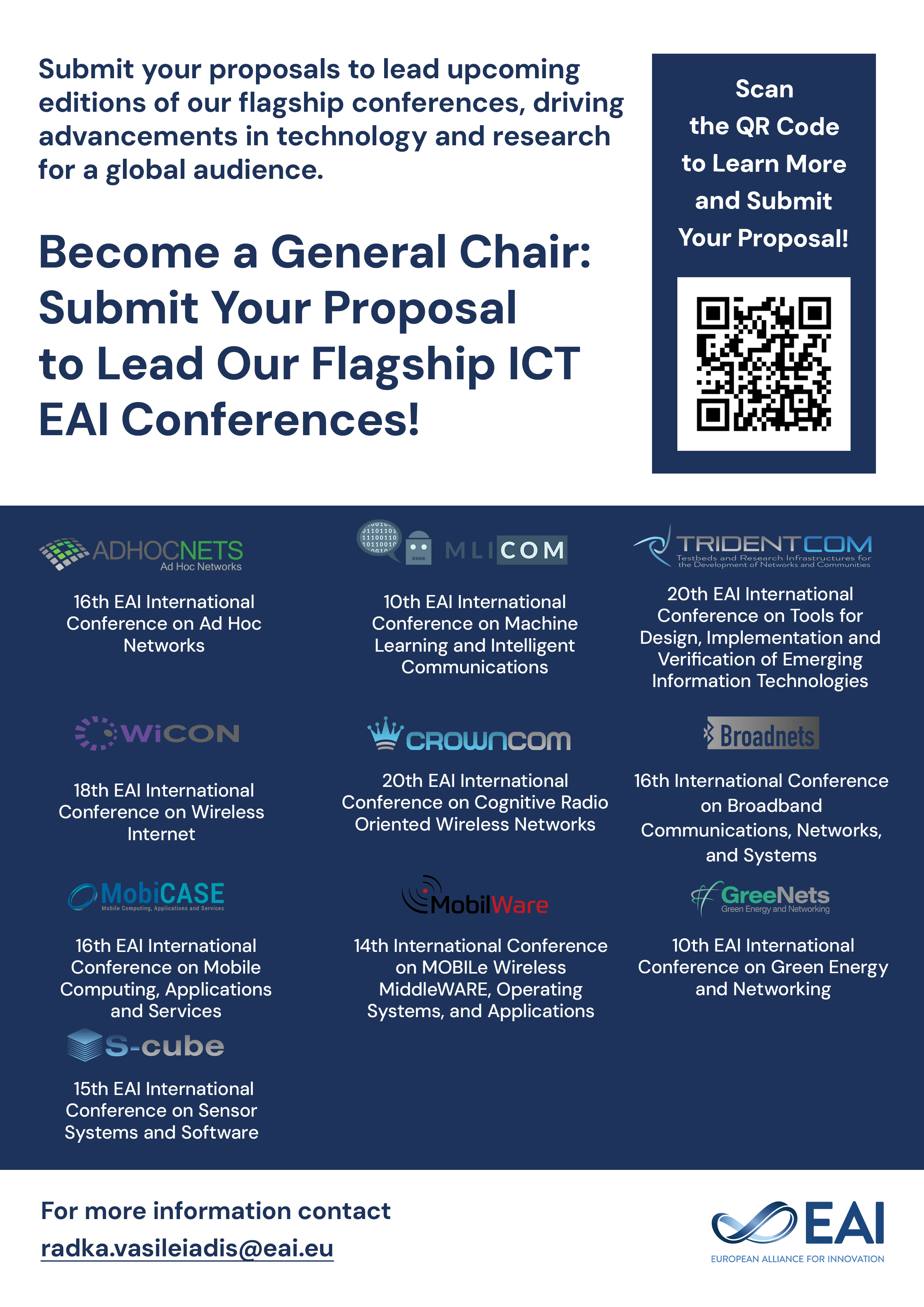
Research Article
Leveraging IoT Device Data for Emotional Health
@INPROCEEDINGS{10.1007/978-3-319-47063-4_53, author={Hariprasad Anumala and Shiva Busetty and Vishal Bharti}, title={Leveraging IoT Device Data for Emotional Health}, proceedings={Internet of Things. IoT Infrastructures. Second International Summit, IoT 360° 2015, Rome, Italy, October 27-29, 2015. Revised Selected Papers, Part I}, proceedings_a={IOT360}, year={2017}, month={1}, keywords={IoT Healthcare Depression Emotional health}, doi={10.1007/978-3-319-47063-4_53} }
- Hariprasad Anumala
Shiva Busetty
Vishal Bharti
Year: 2017
Leveraging IoT Device Data for Emotional Health
IOT360
Springer
DOI: 10.1007/978-3-319-47063-4_53
Abstract
Recent evolution of wearable devices is primarily focused on physical health and fitness but ignore emotional health aspects of an individual. Current health services help user define goals “Reduce weight” but do not provide interfaces for users to define goals as “Stay Happy”. Lot of existing research has focused on sensing user mood classification based on device data but there is limited research that has focused to diagnose and heal depression. A conventional method of doctors detecting depression is based on Hamilton scale of depression with a set of questions and is an intrusive method to probe depression patients. IoT devices are slowly gaining popularity and huge data that is generated from these devices can be leveraged to determine user emotional health. Proposed method attempts to analyze IoT device data and calculate user depression scale and recommends relevant social communication with user social contacts (Friends, Family Members). Identifying precise social contacts and recommending actions and content to recover from early stages of depression is one of the goals of the proposed system. Method recommends relevant social contacts based on current depression score. Proposed system tries to monitor user’s emotional state and more tries to act as preventive health assistant to correct emotional states in early stages and avoids user moving to advanced stages of depression.