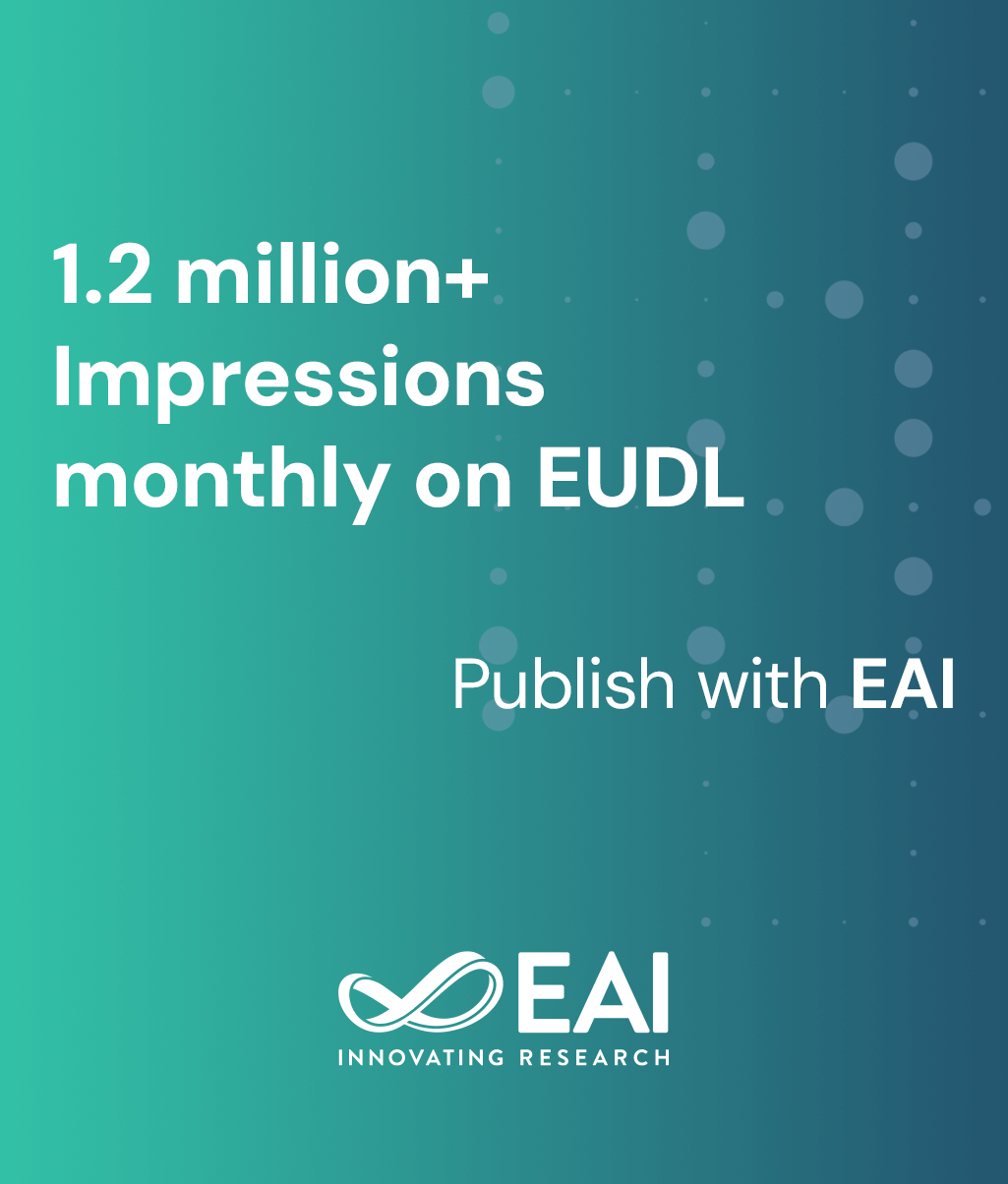
Research Article
FRFE: Fast Recursive Feature Elimination for Credit Scoring
@INPROCEEDINGS{10.1007/978-3-319-46909-6_13, author={Van-Sang Ha and Ha-Nam Nguyen}, title={FRFE: Fast Recursive Feature Elimination for Credit Scoring}, proceedings={Nature of Computation and Communication. Second International Conference, ICTCC 2016, Rach Gia, Vietnam, March 17-18, 2016, Revised Selected Papers}, proceedings_a={ICTCC}, year={2017}, month={1}, keywords={Credit risk Credit scoring Feature selection Random forests RFE Machine learning}, doi={10.1007/978-3-319-46909-6_13} }
- Van-Sang Ha
Ha-Nam Nguyen
Year: 2017
FRFE: Fast Recursive Feature Elimination for Credit Scoring
ICTCC
Springer
DOI: 10.1007/978-3-319-46909-6_13
Abstract
Credit scoring is one of the most important issues in financial decision-making. The use of data mining techniques to build models for credit scoring has been a hot topic in recent years. Classification problems often have a large number of features, but not all of them are useful for classification. Irrelevant and redundant features in credit data may even reduce the classification accuracy. Feature selection is a process of selecting a subset of relevant features, which can decrease the dimensionality, reduce the running time, and improve the accuracy of classifiers. Random forest (RF) is a powerful classification tool which is currently an active research area and successfully solves classification problems in many domains. In this study, we constructed a fast credit scoring model based on parallel Random forests and Recursive Feature Elimination (FRFE) . Two public UCI data sets, Australia and German credit have been used to test our method. The experimental results of the real world data showed that the proposed method results in a higher prediction rate than a baseline method for some certain datasets and also shows comparable and sometimes better performance than the feature selection methods widely used in credit scoring.