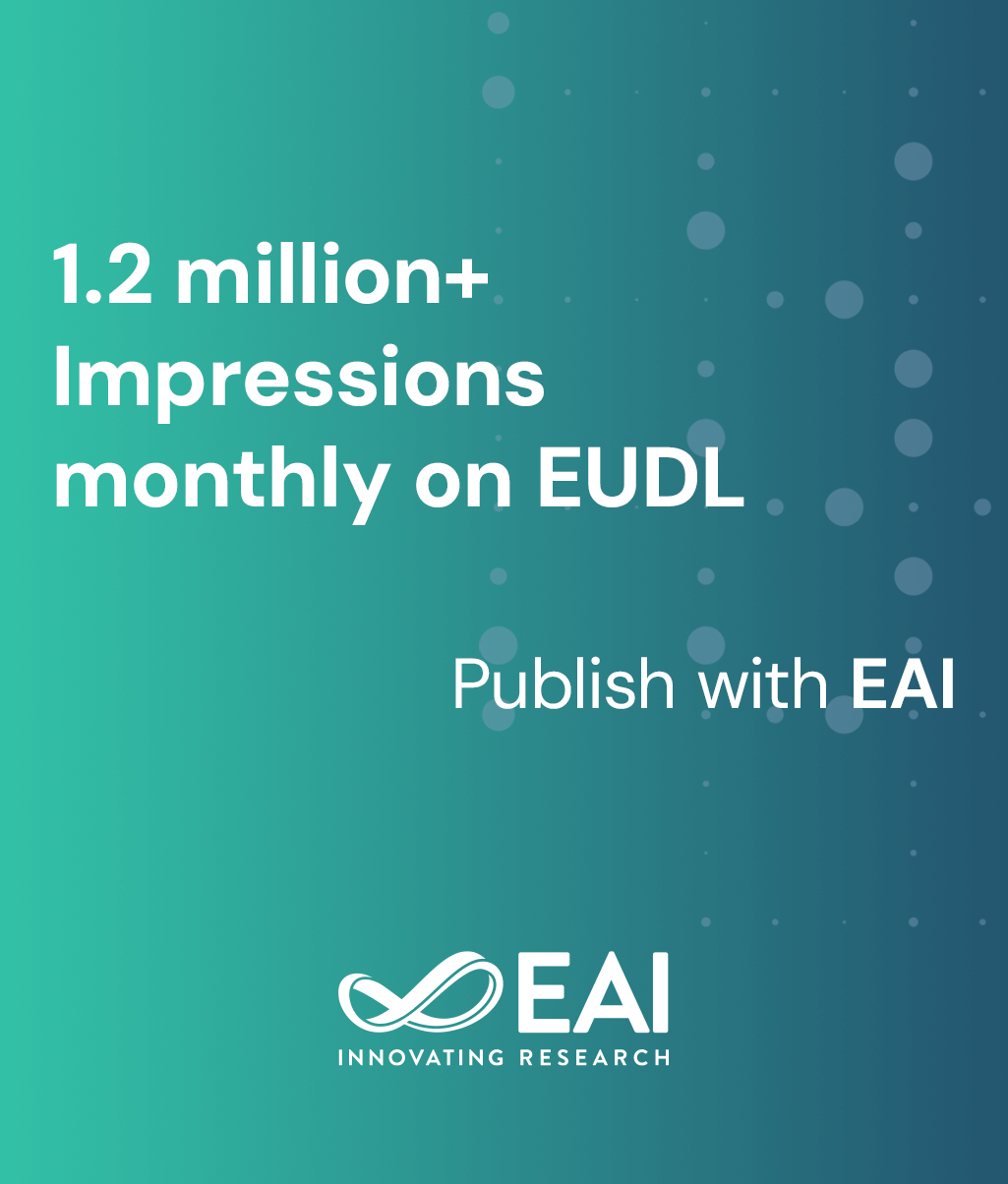
Research Article
Sensing Based Semi-deterministic Inter-Cell Interference Map in Heterogeneous Networks
@INPROCEEDINGS{10.1007/978-3-319-40352-6_15, author={Fatima Kaddour and Dimitri Kt\^{e}nas and Beno\"{\i}t Denis}, title={Sensing Based Semi-deterministic Inter-Cell Interference Map in Heterogeneous Networks}, proceedings={Cognitive Radio Oriented Wireless Networks. 11th International Conference, CROWNCOM 2016, Grenoble, France, May 30 - June 1, 2016, Proceedings}, proceedings_a={CROWNCOM}, year={2016}, month={6}, keywords={Inter-Cell Interference (ICI) Interference Map (IM) Self-learning Spatial interpolation Kriging Long Term Evolution}, doi={10.1007/978-3-319-40352-6_15} }
- Fatima Kaddour
Dimitri Kténas
Benoît Denis
Year: 2016
Sensing Based Semi-deterministic Inter-Cell Interference Map in Heterogeneous Networks
CROWNCOM
Springer
DOI: 10.1007/978-3-319-40352-6_15
Abstract
This paper describes a low computational complexity semi-deterministic Inter-Cell Interference (ICI) map construction procedure. The built Interference Map (IM) gives the ICI level at each pixel of a two-dimensional area, based on an initialization map and ICI levels measured by collaborative User Equipments (UEs). In a first step, the initialization map is obtained with an analytical location-dependent ICI prediction model based on the Poisson Point Process (PPP) framework, where a priori deterministic information about the indoor/outdoor UE status can be injected. The analytical interference map is then updated following a self-learning approach, after spatially interpolating the gap sensed by the UEs with respect to analytical predictions in their visited positions. Two conventional spatial interpolation techniques are thus considered under regular and irregular sensing grids: Inverse Distance Weighting (IDW) and kriging, where exponential and Von Kàrmàn variograms are used. In order to show the benefits of the IM initialization, the performance is compared to that of traditional approaches (i.e., direct spatial interpolation of the ICI measured values), while varying the density of sensing positions.