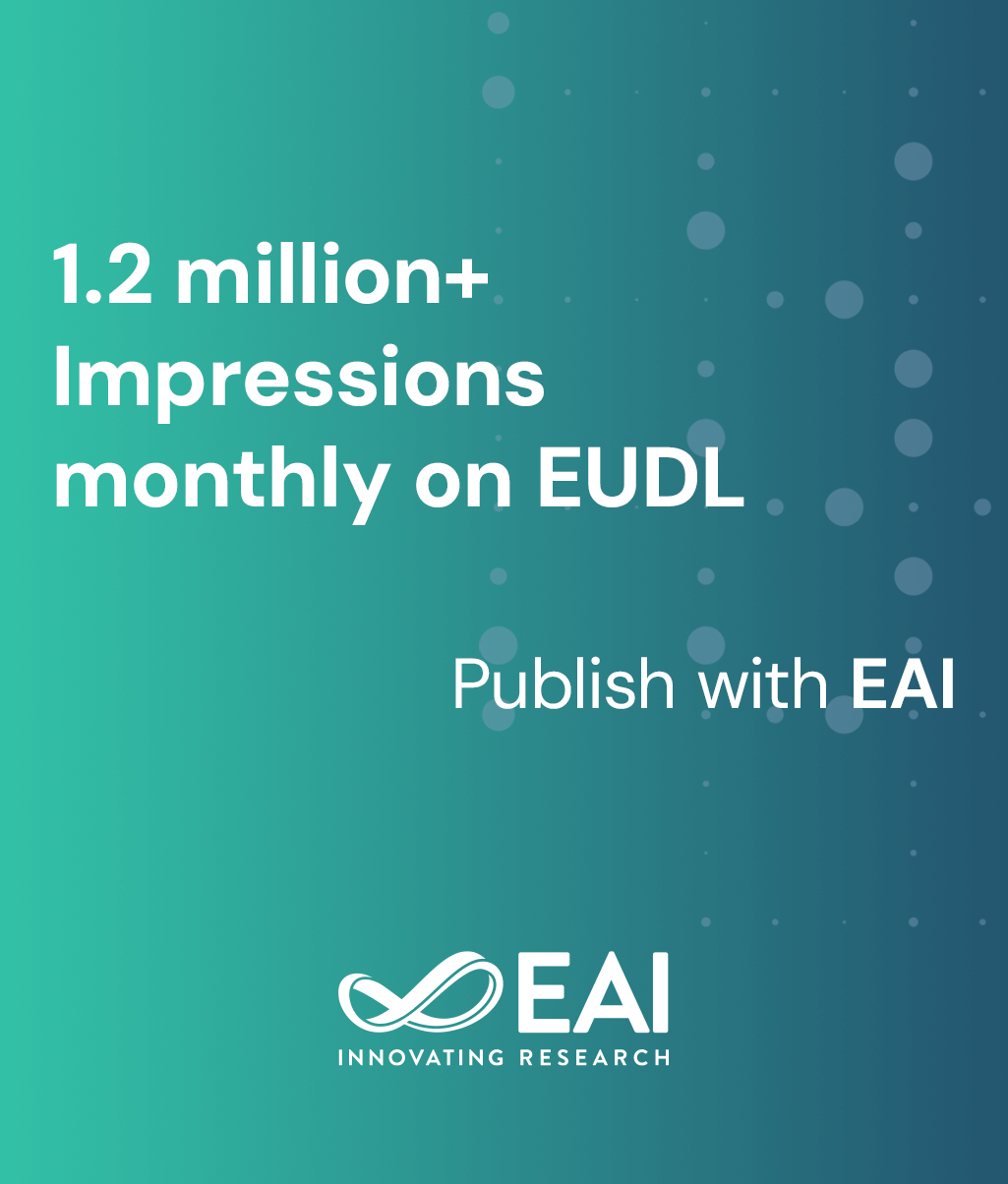
Research Article
A Naive Bayesian Classification Model for Determining Peak Energy Demand in Ontario
@INPROCEEDINGS{10.1007/978-3-319-33681-7_43, author={Bon Ryu and Tokunbo Makanju and Agnieszka Lasek and Xiangdong An and Nick Cercone}, title={A Naive Bayesian Classification Model for Determining Peak Energy Demand in Ontario}, proceedings={Smart City 360°. First EAI International Summit, Smart City 360°, Bratislava, Slovakia and Toronto, Canada, October 13-16, 2015. Revised Selected Papers}, proceedings_a={SMARTCITY360}, year={2016}, month={6}, keywords={Global adjustment Energy Demand Peak Prediction Naive Bayesian Classification}, doi={10.1007/978-3-319-33681-7_43} }
- Bon Ryu
Tokunbo Makanju
Agnieszka Lasek
Xiangdong An
Nick Cercone
Year: 2016
A Naive Bayesian Classification Model for Determining Peak Energy Demand in Ontario
SMARTCITY360
Springer
DOI: 10.1007/978-3-319-33681-7_43
Abstract
In the Canadian Province of Ontario, electricity consumers pay a surcharge for electricity called the Global Adjustment (GA). For large consumers, having the ability to predict the top 5 daily energy demand hours of the year, called 5 Coincident Peaks (5CPs), can save millions of dollars in GA costs, and help decrease peak energy usage. This paper presents a Naive Bayesian classification model for predicting the 5CPs. The model classifies hourly energy demand as being a 5CP hour or not. The model was tested using hourly energy demand for the province of Ontario over a 21 year period (1995–2015). Classifying a day as a 5CP hour containing day yielded a mean precision and recall of 0.49 (0.18) and 0.88 (0.23) (Standard deviation is in brackets), respectively. Targeting the 5CP hours to within three candidate hours of potential 5CP containing days yielded a mean precision and recall of 0.47 (0.19) and 0.83 (0.22), respectively.