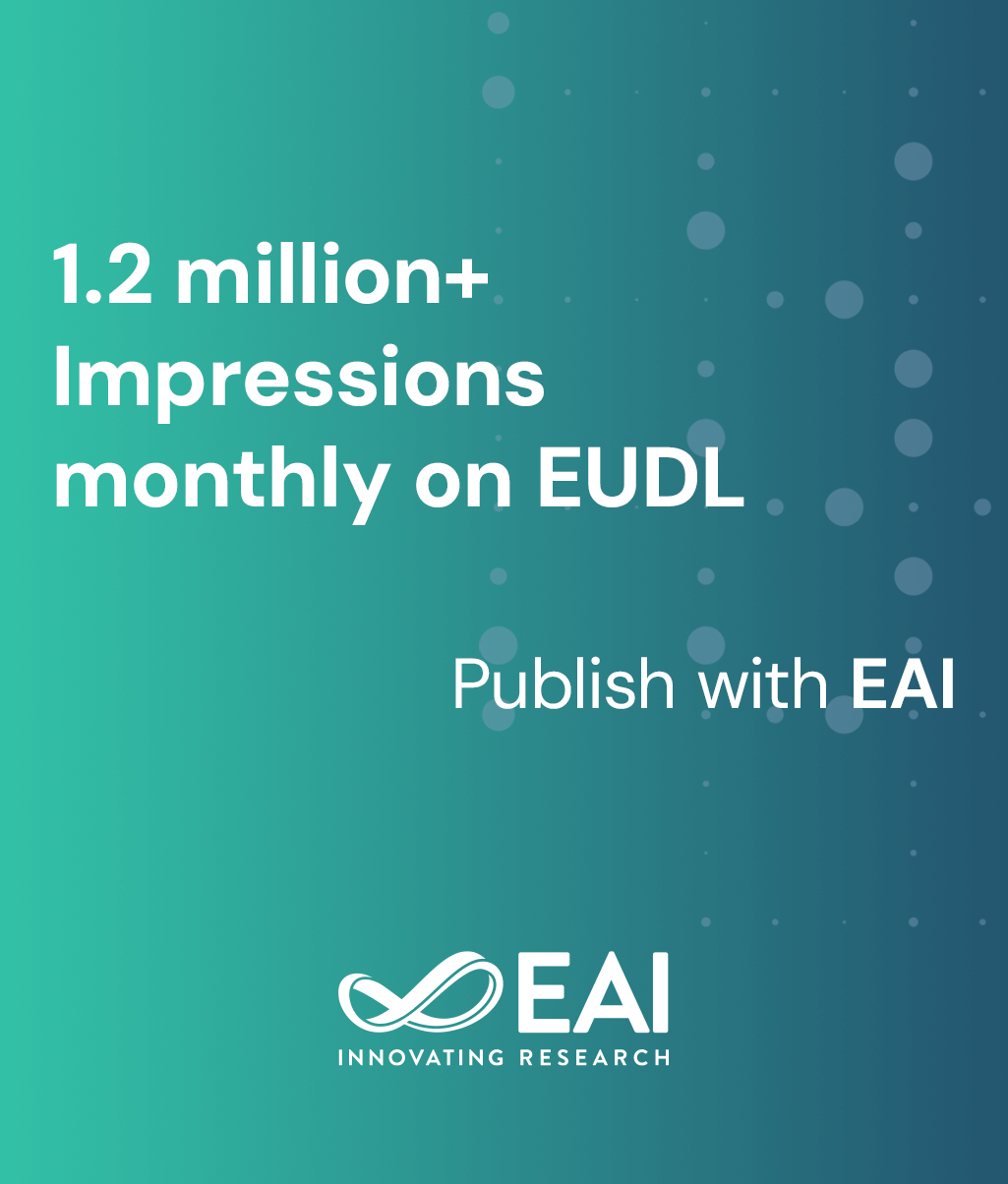
Research Article
Appliance Water Disaggregation via Non-intrusive Load Monitoring (NILM)
@INPROCEEDINGS{10.1007/978-3-319-33681-7_38, author={Bradley Ellert and Stephen Makonin and Fred Popowich}, title={Appliance Water Disaggregation via Non-intrusive Load Monitoring (NILM)}, proceedings={Smart City 360°. First EAI International Summit, Smart City 360°, Bratislava, Slovakia and Toronto, Canada, October 13-16, 2015. Revised Selected Papers}, proceedings_a={SMARTCITY360}, year={2016}, month={6}, keywords={Water disaggregation Water conservation Non-intrusive load monitoring NILM Smart homes Sustainability}, doi={10.1007/978-3-319-33681-7_38} }
- Bradley Ellert
Stephen Makonin
Fred Popowich
Year: 2016
Appliance Water Disaggregation via Non-intrusive Load Monitoring (NILM)
SMARTCITY360
Springer
DOI: 10.1007/978-3-319-33681-7_38
Abstract
The world’s fresh water supply is rapidly dwindling. Informing homeowners of their water use patterns can help them reduce consumption. Today’s smart meters only show a whole house’s water consumption over time. People need to be able to see where they are using water most to be able to change their habits. We are the first to present work where appliance water consumption is non-intrusively disaggregated using the results from a non-intrusive load monitoring algorithm. Unlike previous works that require the installation of water sub-meters or water sensors, our method does not. Further, our method uses low-frequency data from standardized meters and does not rely on labelled data. We modify the Viterbi Algorithm to apply a supervised method to an unsupervised disaggregation problem. We are able to achieve very high accuracy results having mean squared errors of under 0.02 L/min.