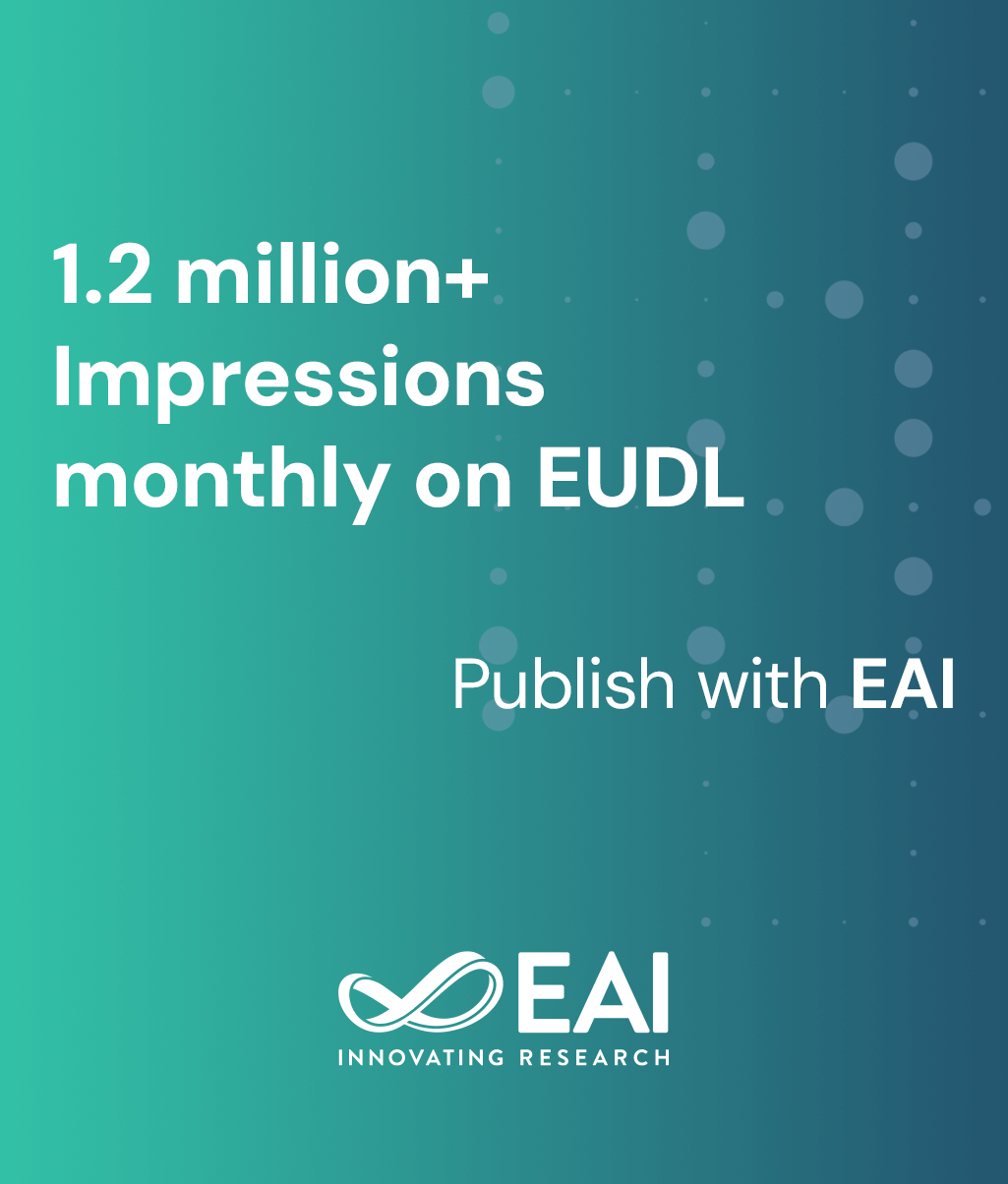
Research Article
Segmentation by Data Point Classification Applied to Forearm Surface EMG
474 downloads
@INPROCEEDINGS{10.1007/978-3-319-33681-7_13, author={Jonathan Lin and Ali-Akbar Samadani and Dana Kulić}, title={Segmentation by Data Point Classification Applied to Forearm Surface EMG}, proceedings={Smart City 360°. First EAI International Summit, Smart City 360°, Bratislava, Slovakia and Toronto, Canada, October 13-16, 2015. Revised Selected Papers}, proceedings_a={SMARTCITY360}, year={2016}, month={6}, keywords={Motion segmentation Surface electromyography Classifiers Pattern recognition}, doi={10.1007/978-3-319-33681-7_13} }
- Jonathan Lin
Ali-Akbar Samadani
Dana Kulić
Year: 2016
Segmentation by Data Point Classification Applied to Forearm Surface EMG
SMARTCITY360
Springer
DOI: 10.1007/978-3-319-33681-7_13
Abstract
Recent advances in wearable technologies have led to the development of new modalities for human-machine interaction such as gesture-based interaction via surface electromyograph (EMG). An important challenge when performing EMG gesture recognition is to temporally segment the individual gestures from continuously recorded time-series data. This paper proposes an approach for EMG data segmentation, by formulating the segmentation problem as a classification task, where a classifier is used to label each data point as either a segment point or a non-segment point. The proposed EMG segmentation approach is used to recognize 9 hand gestures from forearm EMG data of 10 participants and a balanced accuracy of 83 % is achieved.
Copyright © 2015–2025 ICST