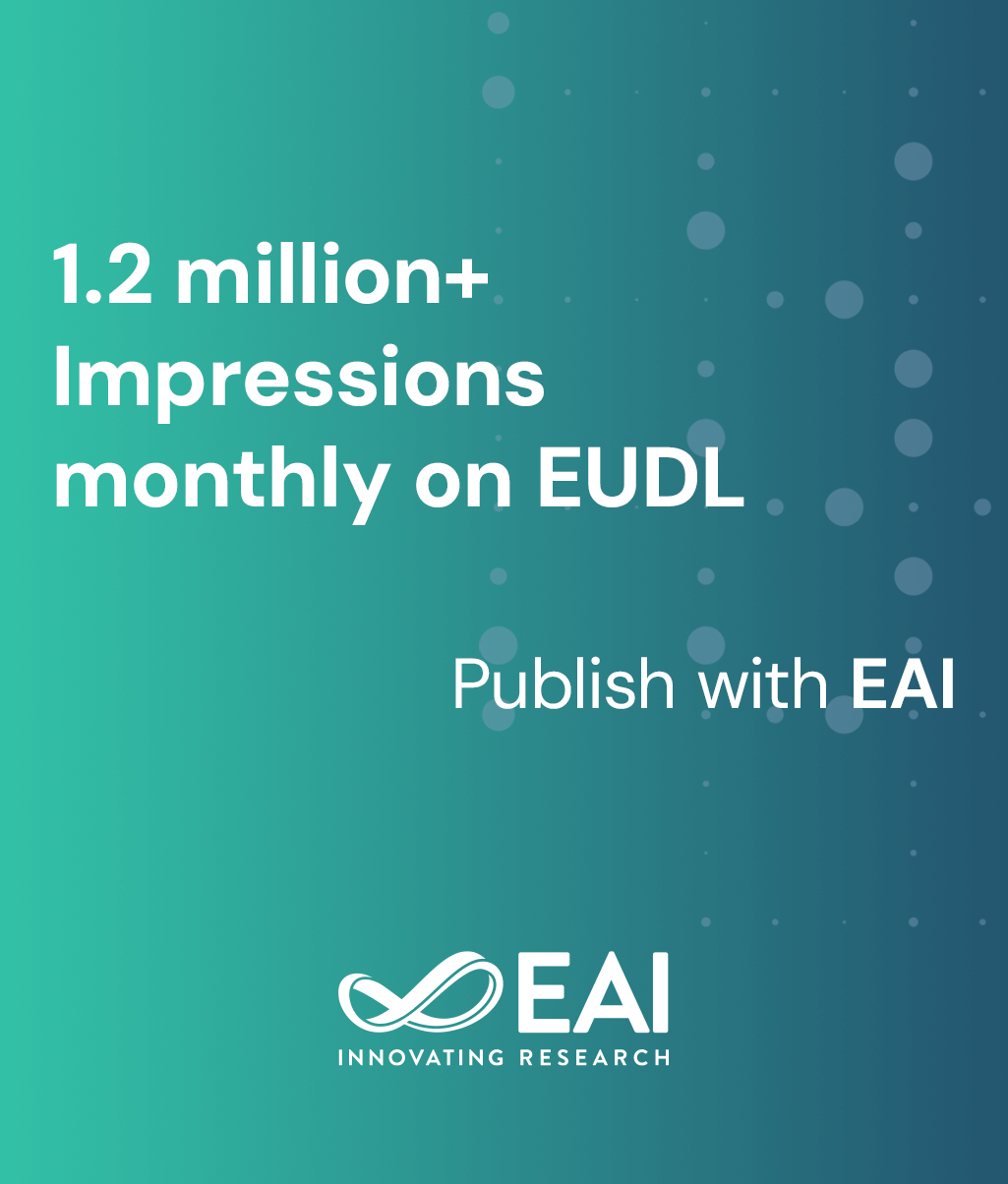
Research Article
Context-Based Classifier Grids Learning for Object Detection in Surveillance Systems
@INPROCEEDINGS{10.1007/978-3-319-29236-6_6, author={Dang Nguyen}, title={Context-Based Classifier Grids Learning for Object Detection in Surveillance Systems}, proceedings={Context-Aware Systems and Applications. 4th International Conference, ICCASA 2015, Vung Tau, Vietnam, November 26-27, 2015, Revised Selected Papers}, proceedings_a={ICCASA}, year={2016}, month={4}, keywords={Context-based learning Classifier grids Object detection Online learning}, doi={10.1007/978-3-319-29236-6_6} }
- Dang Nguyen
Year: 2016
Context-Based Classifier Grids Learning for Object Detection in Surveillance Systems
ICCASA
Springer
DOI: 10.1007/978-3-319-29236-6_6
Abstract
We propose a new method to adaptive object detector is to incorporate the scene specific information without human intervention to reach the goal of fully autonomous surveillance where the focus is on developing adaptive approaches for object detection from single and multiple stationary cameras that are able to incorporate unlabeled information using different types of context in order to collect scene specific samples from both, the background and the object class over time. The main contributions of this paper tackle the question of how to incorporate prior knowledge or scene specific information in an unsupervised manner. Thus, the goal of this work is to increase the recall of scene-specific classifiers while preserving their accuracy and speed. In particular, we introduce a co-training strategy for classifier grids using a robust on-line learner. The system runs at 24 h per day and 7 days per week with 24 frames per second on consumer hardware. Our evaluation show high accuracy on both synthetic and real test sets. We achieve state of the art in our comparisons with related work and in the experimental results these benefits are demonstrated on different publicly available surveillance benchmark data sets.