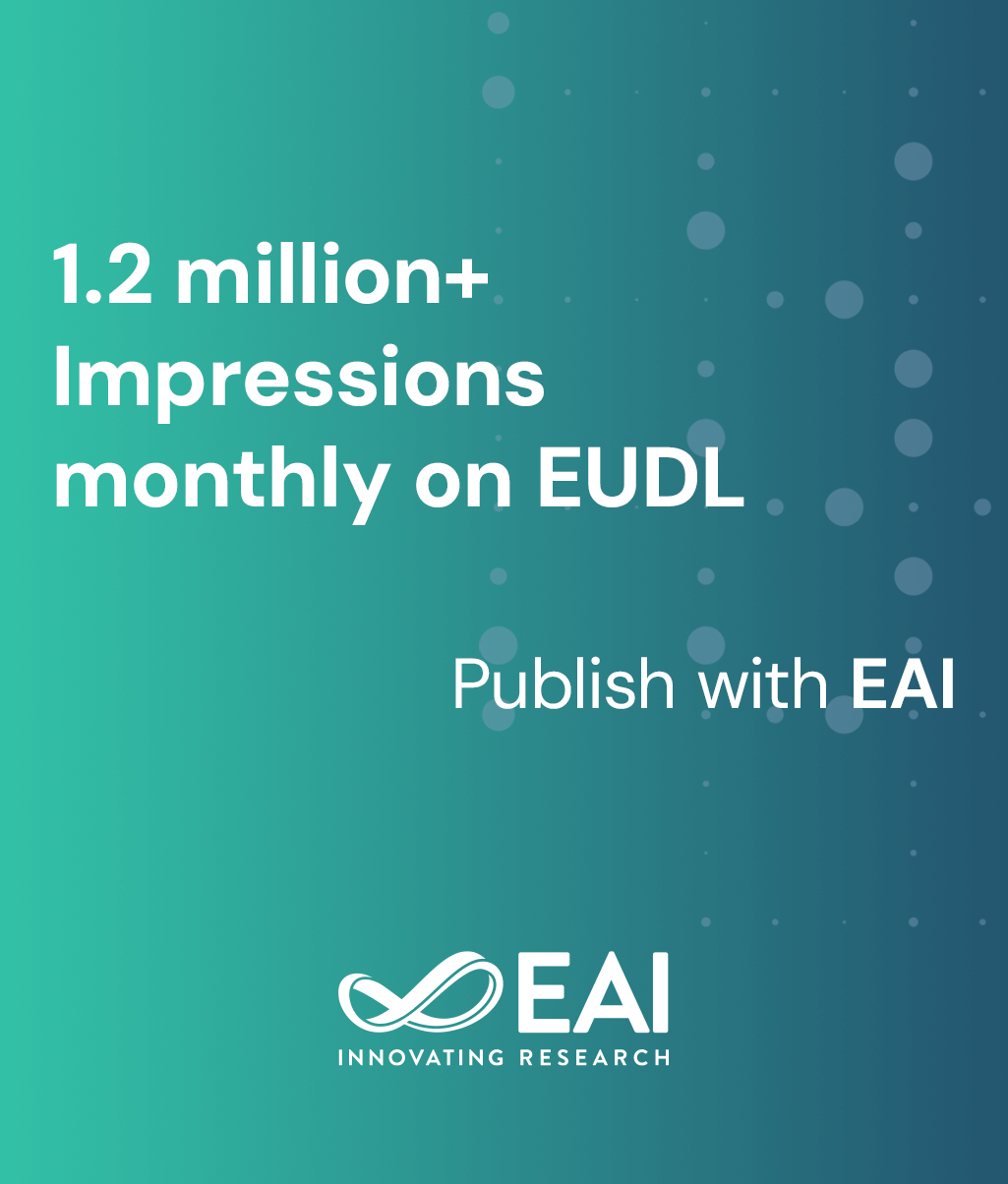
Research Article
Personalized QoS Prediction of Cloud Services via Learning Neighborhood-Based Model
@INPROCEEDINGS{10.1007/978-3-319-28910-6_10, author={Hao Wu and Jun He and Bo Li and Yijian Pei}, title={Personalized QoS Prediction of Cloud Services via Learning Neighborhood-Based Model}, proceedings={Collaborative Computing: Networking, Applications, and Worksharing. 11th International Conference, CollaborateCom 2015, Wuhan, November 10-11, 2015, China. Proceedings}, proceedings_a={COLLABORATECOM}, year={2016}, month={2}, keywords={Cloud services QoS prediction Neighborhood model Parameter learning}, doi={10.1007/978-3-319-28910-6_10} }
- Hao Wu
Jun He
Bo Li
Yijian Pei
Year: 2016
Personalized QoS Prediction of Cloud Services via Learning Neighborhood-Based Model
COLLABORATECOM
Springer
DOI: 10.1007/978-3-319-28910-6_10
Abstract
This paper proposes neighborhood-based approach for QoS-prediction of cloud services by taking advantages of collaborative intelligence. Different from heuristic collaborative filtering and matrix-factorization, we set a formal neighborhood-based prediction framework which allows an efficient global optimization scheme, and then exploits different baseline estimate components to improve predictive performance. To validate our methods, a large-scale QoS-specific dataset which consists of invocation records from 339 service users on 5,825 web services on a world-scale distributed network is used. Experimental results show that the learned neighborhood-based models can overcome existing difficulties of heuristic collaborative filtering methods and achieve superior performance than state-of-the-art prediction methods.