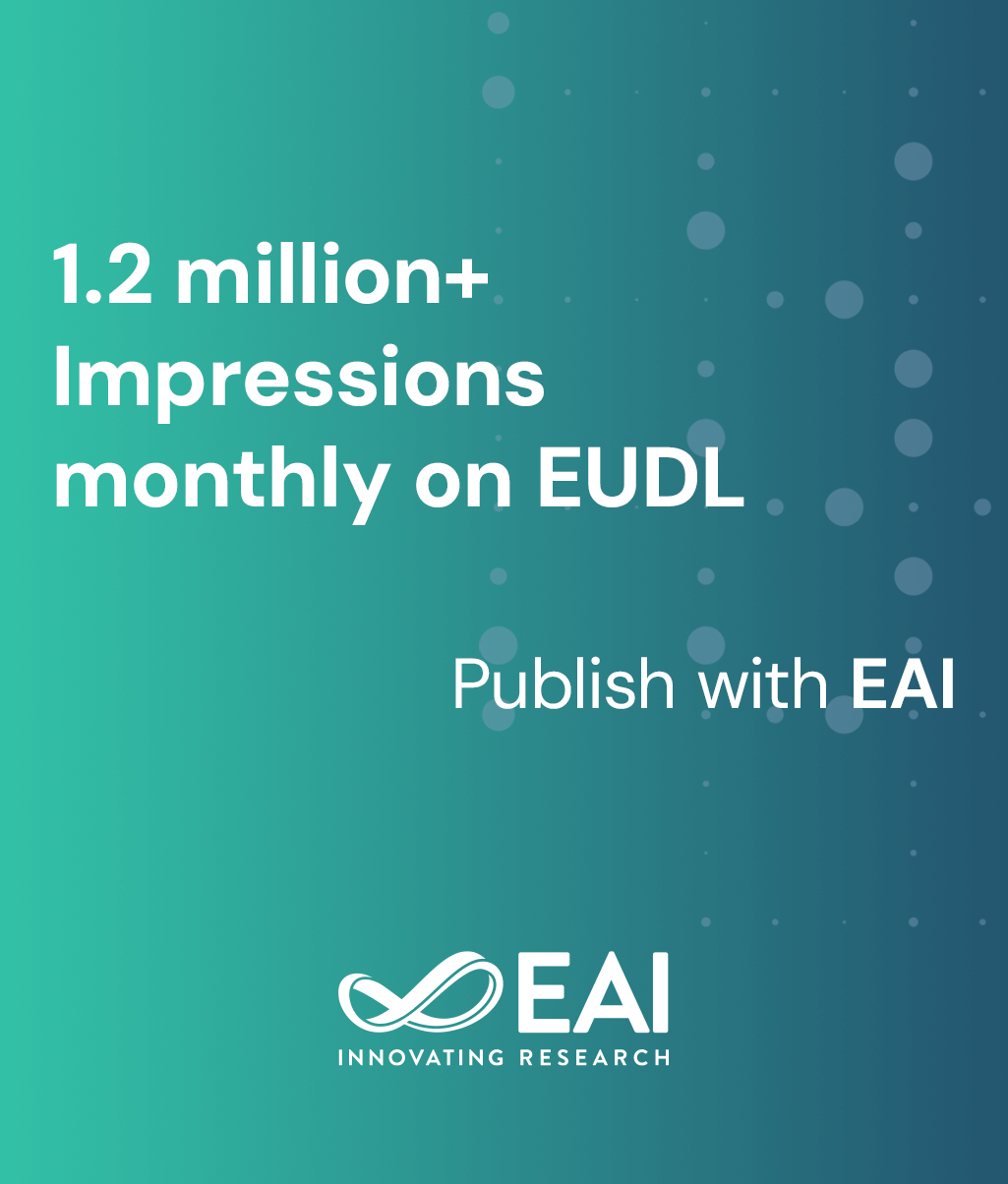
Research Article
Community-Based Collaborative Intrusion Detection
@INPROCEEDINGS{10.1007/978-3-319-28865-9_44, author={Carlos Cordero and Emmanouil Vasilomanolakis and Max M\'{y}hlh\aa{}user and Mathias Fischer}, title={Community-Based Collaborative Intrusion Detection}, proceedings={Security and Privacy in Communication Networks. 11th International Conference, SecureComm 2015, Dallas, TX, USA, October 26-29, 2015, Revised Selected Papers}, proceedings_a={SECURECOMM}, year={2016}, month={2}, keywords={}, doi={10.1007/978-3-319-28865-9_44} }
- Carlos Cordero
Emmanouil Vasilomanolakis
Max Mühlhäuser
Mathias Fischer
Year: 2016
Community-Based Collaborative Intrusion Detection
SECURECOMM
Springer
DOI: 10.1007/978-3-319-28865-9_44
Abstract
The IT infrastructure of today needs to be ready to defend against massive cyber-attacks which often originate from distributed attackers such as Botnets. Most Intrusion Detection Systems (IDSs), nonetheless, are still working in isolation and cannot effectively detect distributed attacks. Collaborative IDSs (CIDSs) have been proposed as a collaborative defense against the ever more sophisticated distributed attacks. However, collaboration by exchanging suspicious alarms among all interconnected sensors in CIDSs does not scale with the size of the IT infrastructure; hence, detection performance and communication overhead, required for collaboration, must be traded off. We propose to partition the set of considered sensors into subsets, or , as a lever for this trade off. The novelty of our approach is the application of ensemble based learning, a machine learning paradigm suitable for distributed intrusion detection. In our approach, community members exchange data features used to train models of normality, not bare alarms, thereby further reducing the communication overhead of our approach. Our experiments show that we can achieve detection rates close to those based on global information exchange with smaller subsets of collaborating sensors.