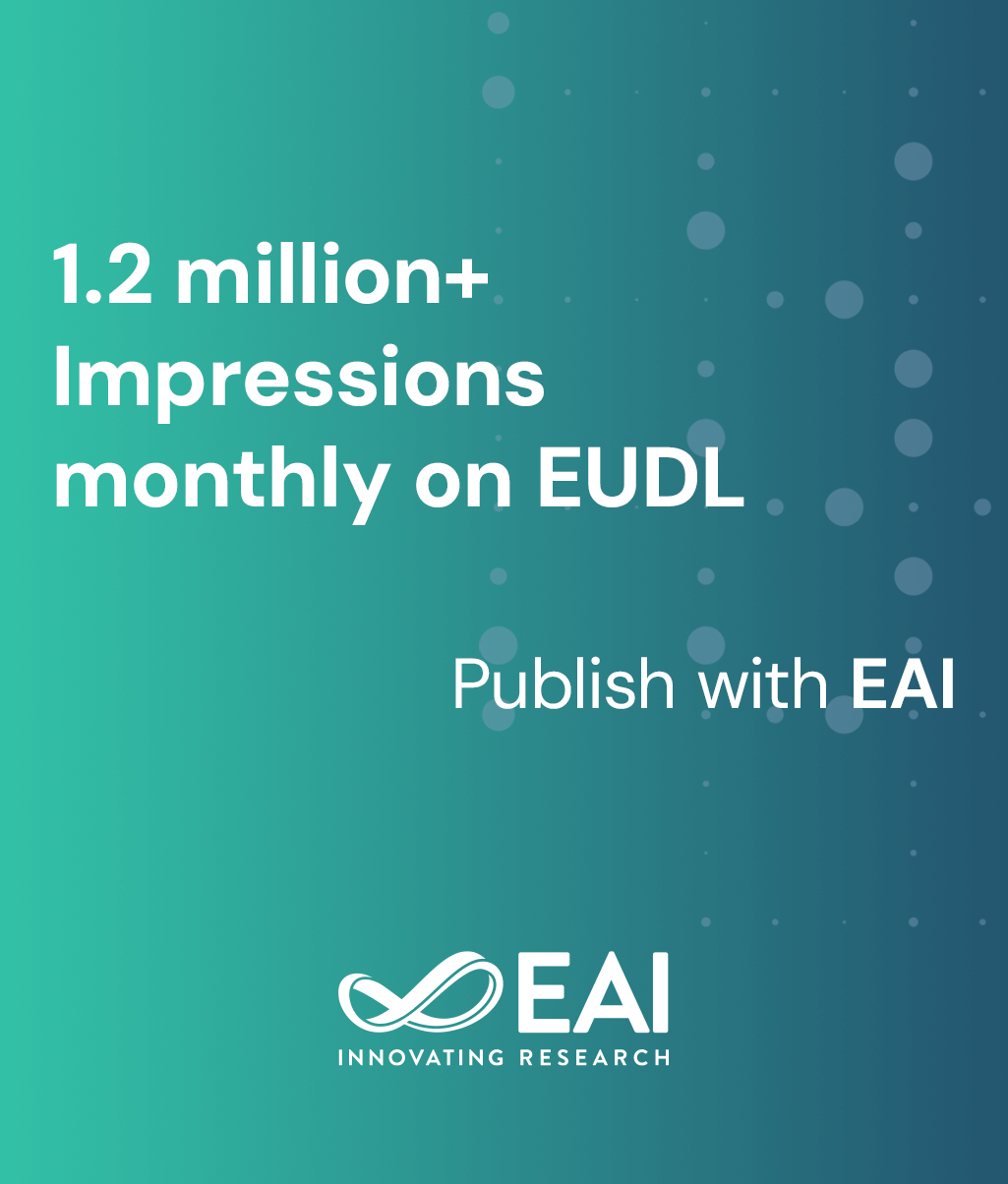
Research Article
TADOOP: Mining Network Traffic Anomalies with Hadoop
554 downloads
@INPROCEEDINGS{10.1007/978-3-319-28865-9_10, author={Geng Tian and Zhiliang Wang and Xia Yin and Zimu Li and Xingang Shi and Ziyi Lu and Chao Zhou and Yang Yu and Dan Wu}, title={TADOOP: Mining Network Traffic Anomalies with Hadoop}, proceedings={Security and Privacy in Communication Networks. 11th International Conference, SecureComm 2015, Dallas, TX, USA, October 26-29, 2015, Revised Selected Papers}, proceedings_a={SECURECOMM}, year={2016}, month={2}, keywords={Tsallis entropy Traffic anomaly detection Hadoop Big data MapReduce}, doi={10.1007/978-3-319-28865-9_10} }
- Geng Tian
Zhiliang Wang
Xia Yin
Zimu Li
Xingang Shi
Ziyi Lu
Chao Zhou
Yang Yu
Dan Wu
Year: 2016
TADOOP: Mining Network Traffic Anomalies with Hadoop
SECURECOMM
Springer
DOI: 10.1007/978-3-319-28865-9_10
Abstract
Today, various anomalies and large number of flows in a network make traffic anomaly detection a big challenge. In this paper, we propose - (ual sallis ntropy for flow eature with roperties), a more efficient method for traffic anomaly detection. To handle huge amount of traffic, based on Hadoop, we implement a network traffic anomaly detection system named TADOOP, which supports semi-automatic training and both offline and online traffic anomaly detection. TADOOP with a cluster of five servers has been deployed in Tsinghua University Campus Network. Furthermore, we compare DTE-FP with Tsallis entropy, and the experimental results show that DTE-FP has much better detection capability than Tsallis entropy.
Copyright © 2015–2025 ICST