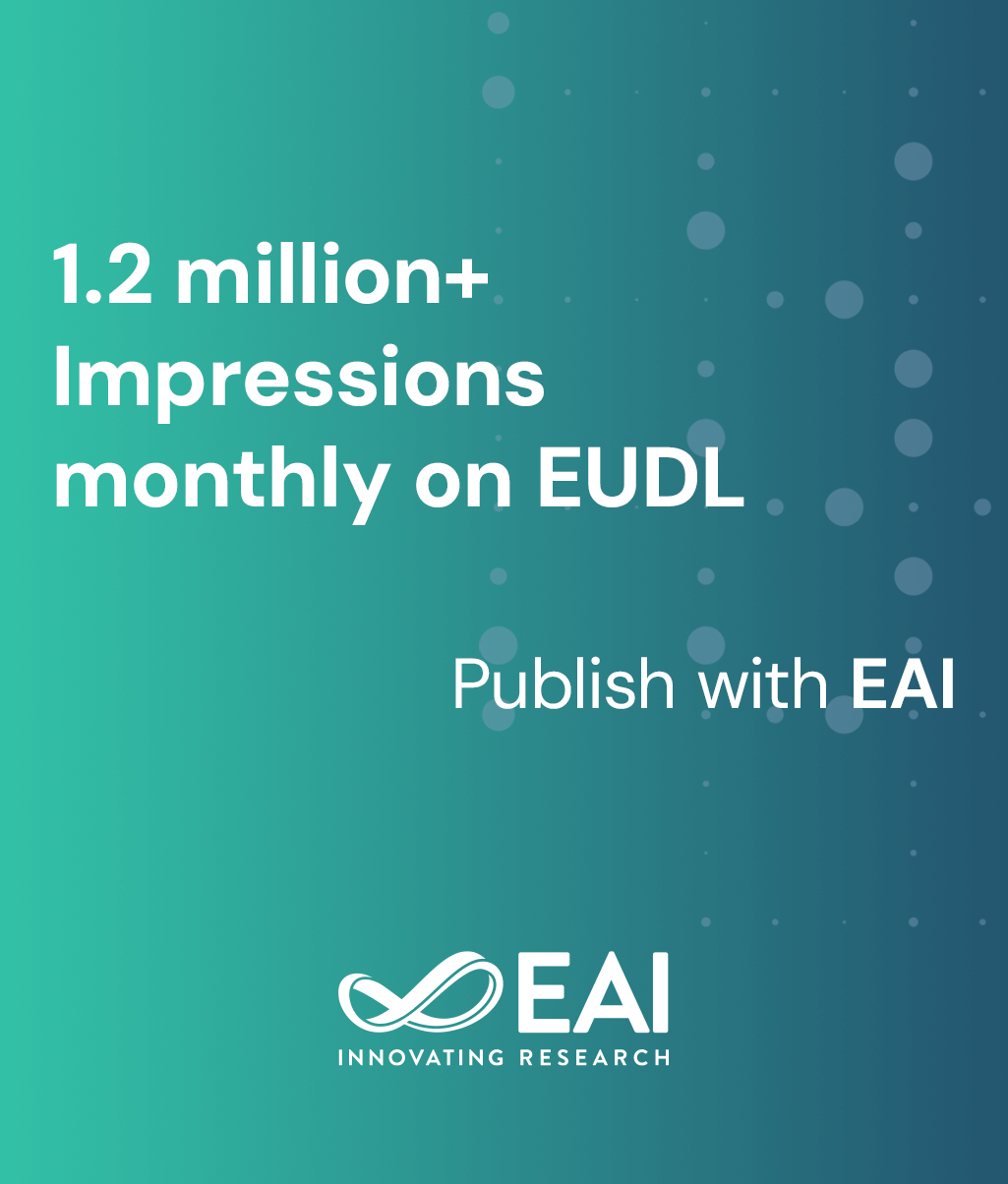
Research Article
Detecting Malicious Sessions Through Traffic Fingerprinting Using Hidden Markov Models
@INPROCEEDINGS{10.1007/978-3-319-23829-6_47, author={Sami Zhioua and Adnene Jabeur and Mahjoub Langar and Wael Ilahi}, title={Detecting Malicious Sessions Through Traffic Fingerprinting Using Hidden Markov Models}, proceedings={International Conference on Security and Privacy in Communication Networks. 10th International ICST Conference, SecureComm 2014, Beijing, China, September 24-26, 2014, Revised Selected Papers, Part I}, proceedings_a={SECURECOMM}, year={2015}, month={11}, keywords={Malware detection Hidden Markov Model (HMM) Malicious sessions Traffic analysis}, doi={10.1007/978-3-319-23829-6_47} }
- Sami Zhioua
Adnene Jabeur
Mahjoub Langar
Wael Ilahi
Year: 2015
Detecting Malicious Sessions Through Traffic Fingerprinting Using Hidden Markov Models
SECURECOMM
Springer
DOI: 10.1007/978-3-319-23829-6_47
Abstract
Almost any malware attack involves data communication between the infected host and the attacker host/server allowing the latter to remotely control the infected host. The remote control is achieved through opening different types of sessions such as remote desktop, webcam video streaming, file transfer, etc. In this paper, we present a traffic analysis based malware detection technique using Hidden Markov Model (HMM). The main contribution is that the proposed system does not only detect malware infections but also identifies with precision the type of malicious session opened by the attacker. The empirical analysis shows that the proposed detection system has a stable identification precision of 90 % and that it allows to identify between 40 % and 75 % of all malicious sessions in typical network traffic.