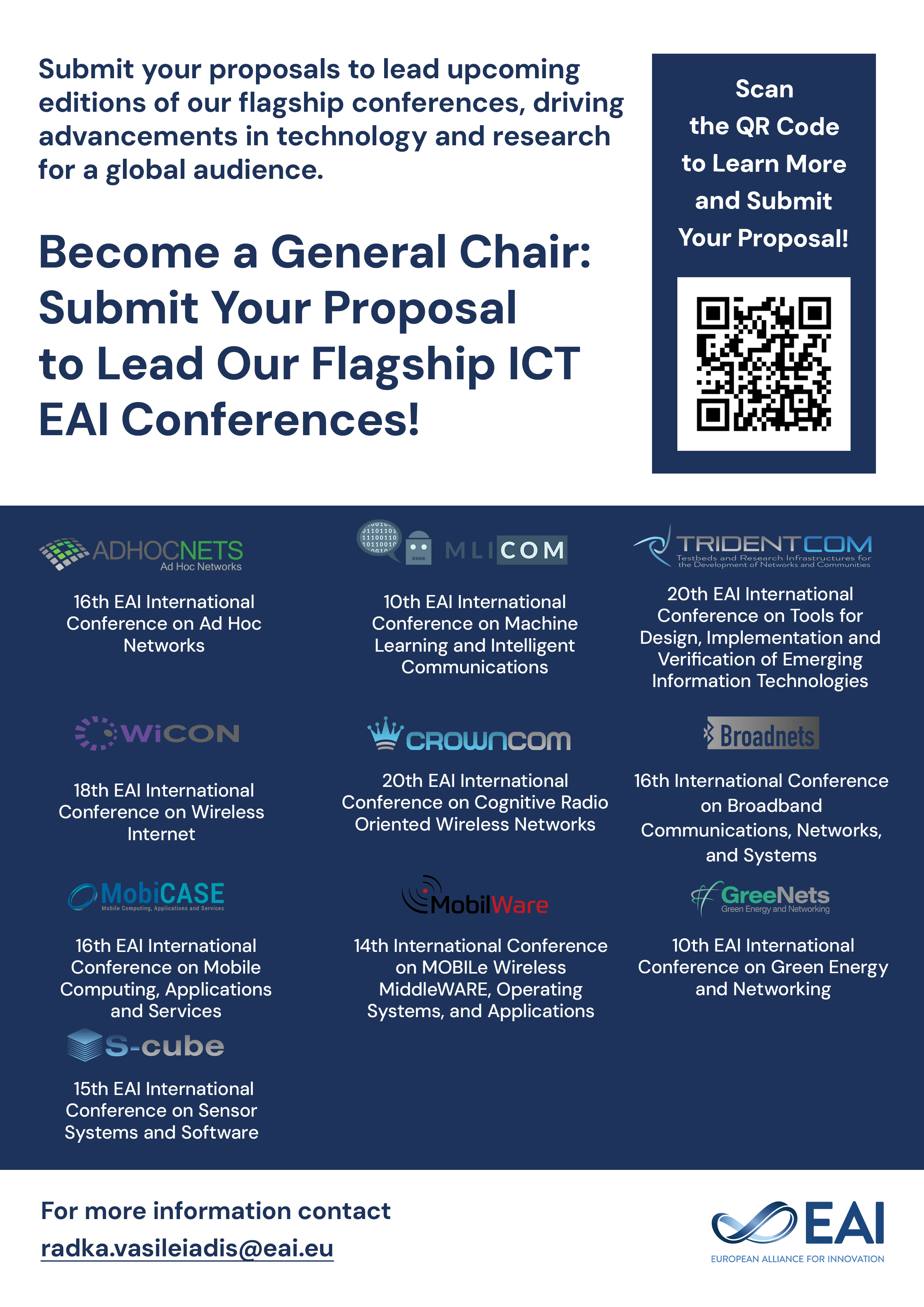
Research Article
Novel Iterative Min-Max Clustering to Minimize Information Loss in Statistical Disclosure Control
@INPROCEEDINGS{10.1007/978-3-319-23802-9_14, author={Abdun Mahmood and Md Kabir and Abdul Mustafa}, title={Novel Iterative Min-Max Clustering to Minimize Information Loss in Statistical Disclosure Control}, proceedings={International Conference on Security and Privacy in Communication Networks. 10th International ICST Conference, SecureComm 2014, Beijing, China, September 24-26, 2014, Revised Selected Papers, Part II}, proceedings_a={SECURECOMM}, year={2015}, month={12}, keywords={Privacy Microaggregation Microdata protection -anonymity Disclosure control}, doi={10.1007/978-3-319-23802-9_14} }
- Abdun Mahmood
Md Kabir
Abdul Mustafa
Year: 2015
Novel Iterative Min-Max Clustering to Minimize Information Loss in Statistical Disclosure Control
SECURECOMM
Springer
DOI: 10.1007/978-3-319-23802-9_14
Abstract
In recent years, there has been an alarming increase of online identity theft and attacks using personally identifiable information. The goal of privacy preservation is to de-associate individuals from sensitive or microdata information. Microaggregation techniques seeks to protect microdata in such a way that can be published and mined without providing any private information that can be linked to specific individuals. Microaggregation works by partitioning the microdata into groups of at least records and then replacing the records in each group with the centroid of the group. An optimal microaggregation method must minimize the information loss resulting from this replacement process. The challenge is how to minimize the information loss during the microaggregation process. This paper presents a new microaggregation technique for Statistical Disclosure Control (SDC). It consists of two stages. In the first stage, the algorithm sorts all the records in the data set in a particular way to ensure that during microaggregation very dissimilar observations are never entered into the same cluster. In the second stage an optimal microaggregation method is used to create -anonymous clusters while minimizing the information loss. It works by taking the sorted data and simultaneously creating two distant clusters using the two extreme sorted values as seeds for the clusters. The performance of the proposed technique is compared against the most recent microaggregation methods. Experimental results using benchmark datasets show that the proposed algorithm has the lowest information loss compared with a basket of techniques in the literature.