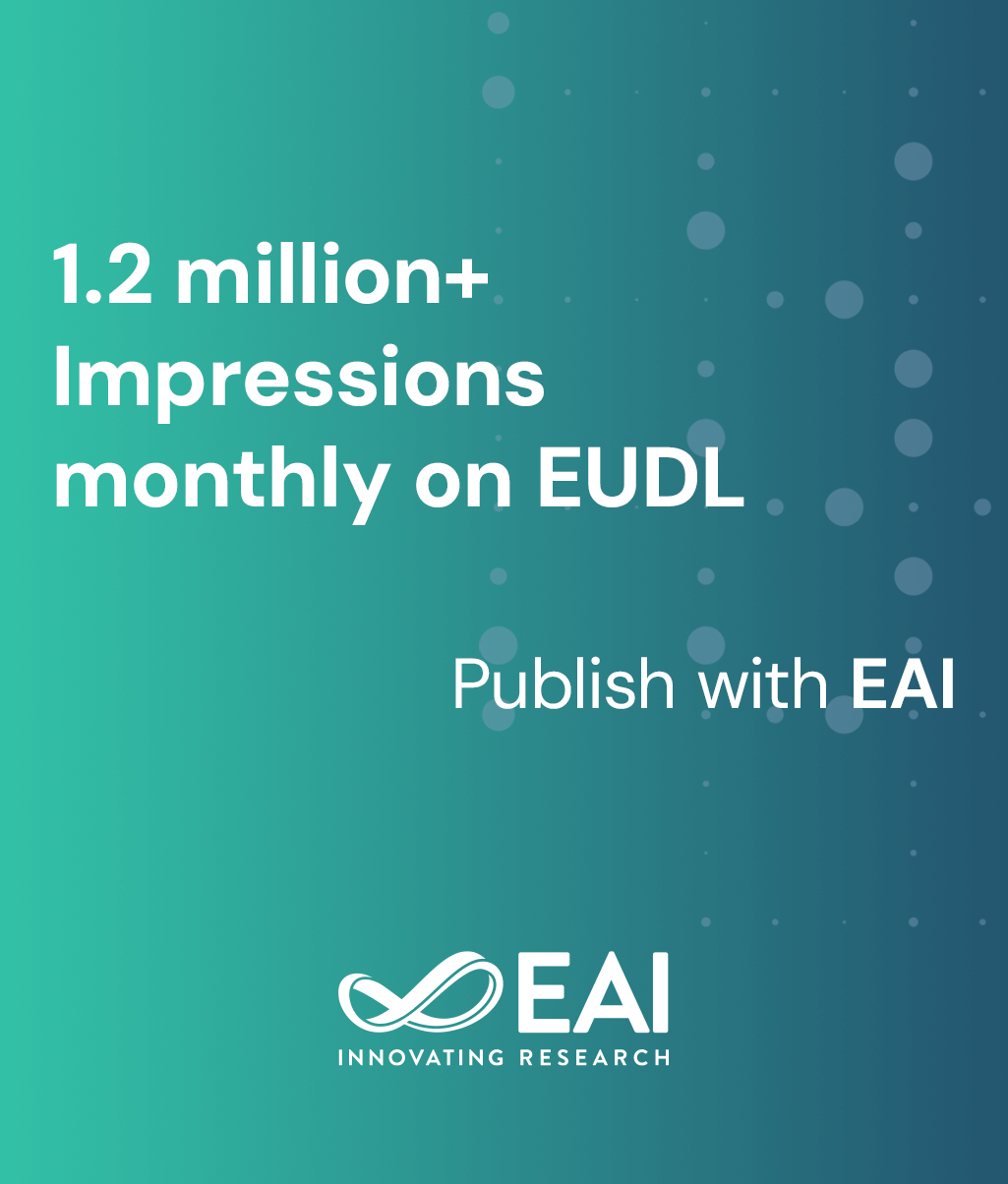
Research Article
Understanding the Impact of Data Sparsity and Duration for Location Prediction Applications
@INPROCEEDINGS{10.1007/978-3-319-19743-2_29, author={Alasdair Thomason and Matthew Leeke and Nathan Griffiths}, title={Understanding the Impact of Data Sparsity and Duration for Location Prediction Applications}, proceedings={Internet of Things. IoT Infrastructures. First International Summit, IoT360 2014, Rome, Italy, October 27-28, 2014, Revised Selected Papers, Part II}, proceedings_a={IOT360}, year={2015}, month={7}, keywords={Collection Data Duration Location prediction Sparsity}, doi={10.1007/978-3-319-19743-2_29} }
- Alasdair Thomason
Matthew Leeke
Nathan Griffiths
Year: 2015
Understanding the Impact of Data Sparsity and Duration for Location Prediction Applications
IOT360
Springer
DOI: 10.1007/978-3-319-19743-2_29
Abstract
As mobile devices capable of sensing location have become pervasive, the collection and transmission of location data has become commonplace, enabling the creation of models of behaviour that support location prediction. With such devices often heavily resource-constrained, the nature of data used in location prediction must be understood in order to optimise storage and processing requirements. This paper specifically explores data sparsity and collection duration. The results presented provide insight which suggest: (i) a relationship of diminishing returns in predictive accuracy when collecting user location data at increased rates over a fixed period, and (ii) the duration over which a fixed size sample of location data is collected has a greater impact on predicative accuracy than data sparsity.