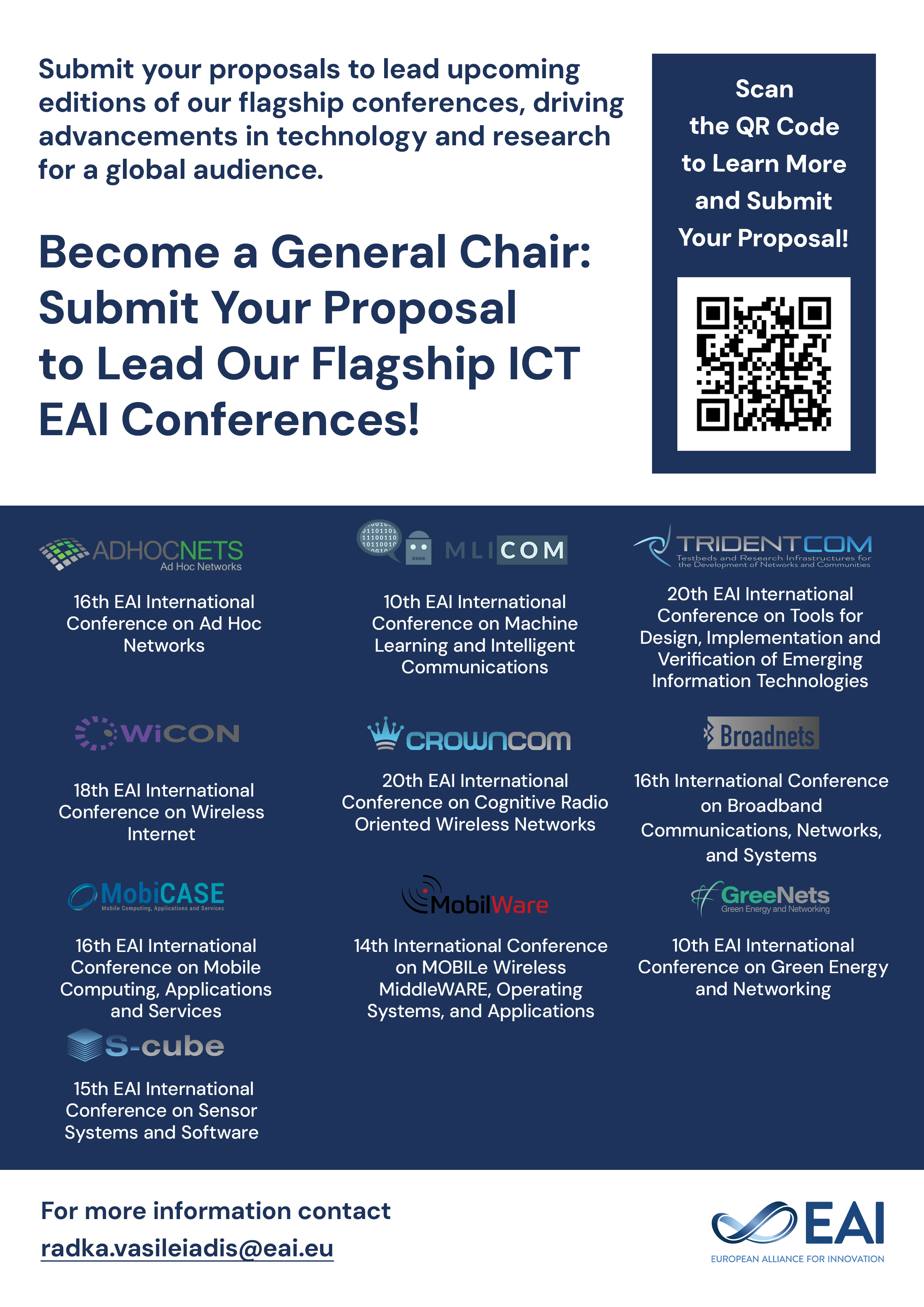
Research Article
Cognitive Load Detection on Commercial EEG Devices: An Optimized Signal Processing Chain
@INPROCEEDINGS{10.1007/978-3-319-19656-5_14, author={Arijit Sinharay and Debatri Chatterjee and Arpan Pal}, title={Cognitive Load Detection on Commercial EEG Devices: An Optimized Signal Processing Chain}, proceedings={Internet of Things. User-Centric IoT. First International Summit, IoT360 2014, Rome, Italy, October 27-28, 2014, Revised Selected Papers, Part I}, proceedings_a={IOT360}, year={2015}, month={7}, keywords={Cognitive load Mental workload EEG signal processing Emotiv}, doi={10.1007/978-3-319-19656-5_14} }
- Arijit Sinharay
Debatri Chatterjee
Arpan Pal
Year: 2015
Cognitive Load Detection on Commercial EEG Devices: An Optimized Signal Processing Chain
IOT360
Springer
DOI: 10.1007/978-3-319-19656-5_14
Abstract
Use of Electroencephalography (EEG) to detect cognitive load is a well-practiced technique. Cognitive load reflects the mental load imparted on a person providing a crucial parameter for applications like personalized education and usability testing. There are several approaches to process the EEG signals and thus choosing an optimal signal processing chain is not a straight forward job. The scenario becomes even more interesting while using commercial low-cost, low resolution EEG devices connected to cloud through Internet of Things (IoT) platform. This paper proposes an optimized signal processing chain offering maximum classification accuracy and minimum computational complexity for measuring the cognitive load using low resolution EEG devices.