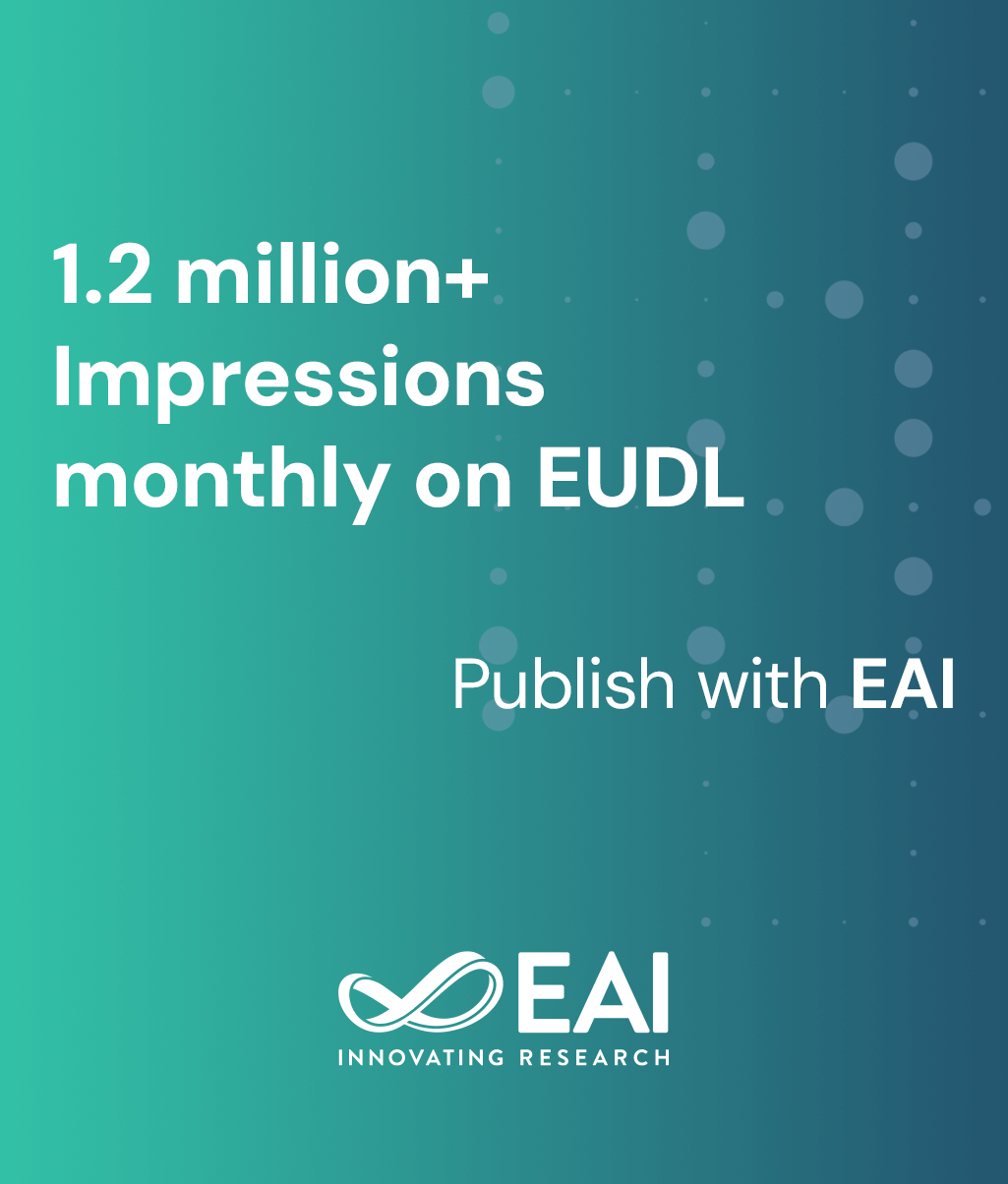
Research Article
Un-normlized and Random Walk Hypergraph Laplacian Un-supervised Learning
@INPROCEEDINGS{10.1007/978-3-319-15392-6_25, author={Loc Tran and Linh Tran and Hoang Trang}, title={Un-normlized and Random Walk Hypergraph Laplacian Un-supervised Learning}, proceedings={Nature of Computation and Communication. International Conference, ICTCC 2014, Ho Chi Minh City, Vietnam, November 24-25, 2014, Revised Selected Papers}, proceedings_a={ICTCC}, year={2015}, month={2}, keywords={Hypergraph Laplacian Clustering Un-supervised learning}, doi={10.1007/978-3-319-15392-6_25} }
- Loc Tran
Linh Tran
Hoang Trang
Year: 2015
Un-normlized and Random Walk Hypergraph Laplacian Un-supervised Learning
ICTCC
ICST
DOI: 10.1007/978-3-319-15392-6_25
Abstract
Most network-based clustering methods are based on the assumption that the labels of two adjacent vertices in the network are likely to be the same. However, assuming the pairwise relationship between vertices is not complete. The information a group of vertices that show very similar patterns and tend to have similar labels is missed. The natural way overcoming the information loss of the above assumption is to represent the given data as the hypergraph. Thus, in this paper, the two un-normalized and random walk hypergraph Laplacian based un-supervised learning methods are introduced. Experiment results show that the accuracy performance measures of these two hypergraph Laplacian based un-supervised learning methods are greater than the accuracy performance measure of symmetric normalized graph Laplacian based un-supervised learning method (i.e. the baseline method of this paper) applied to simple graph created from the incident matrix of hypergraph.