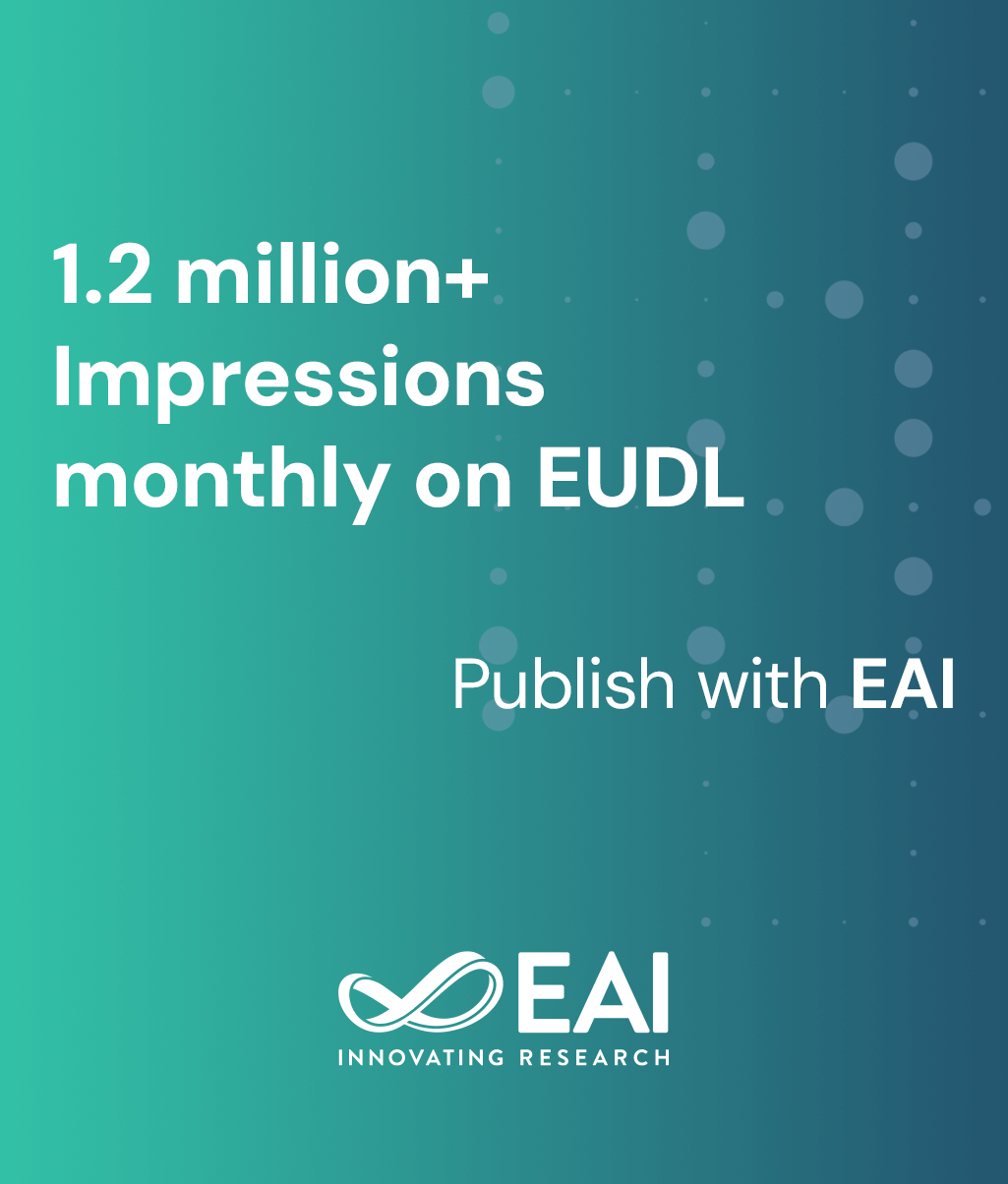
Research Article
Semi Supervised Learning Based Text Classification Model for Multi Label Paradigm
@INPROCEEDINGS{10.1007/978-3-319-11629-7_26, author={Shweta Dharmadhikari and Maya Ingle and Parag Kulkarni}, title={Semi Supervised Learning Based Text Classification Model for Multi Label Paradigm}, proceedings={Signal Processing and Information Technology. Second International Joint Conference, SPIT 2012, Dubai, UAE, September 20-21, 2012, Revised Selected Papers}, proceedings_a={SPIT}, year={2014}, month={11}, keywords={Automatic text categorization Multi-label text classification graph based framework semi supervised learning}, doi={10.1007/978-3-319-11629-7_26} }
- Shweta Dharmadhikari
Maya Ingle
Parag Kulkarni
Year: 2014
Semi Supervised Learning Based Text Classification Model for Multi Label Paradigm
SPIT
Springer
DOI: 10.1007/978-3-319-11629-7_26
Abstract
Automatic text categorization (ATC) is a prominent research area within Information retrieval. Through this paper a classification model for ATC in multi-label domain is discussed. We are proposing a new multi label text classification model for assigning more relevant set of categories to every input text document. Our model is greatly influenced by graph based framework and Semi supervised learning. We demonstrate the effectiveness of our model using Enron, Slashdot, Bibtex and RCV1 datasets. We also compare performance of our model with few popular existing supervised techniques. Our experimental results indicate that the use of Semi Supervised Learning in multi label text classification greatly improves the decision making capability of classifier.