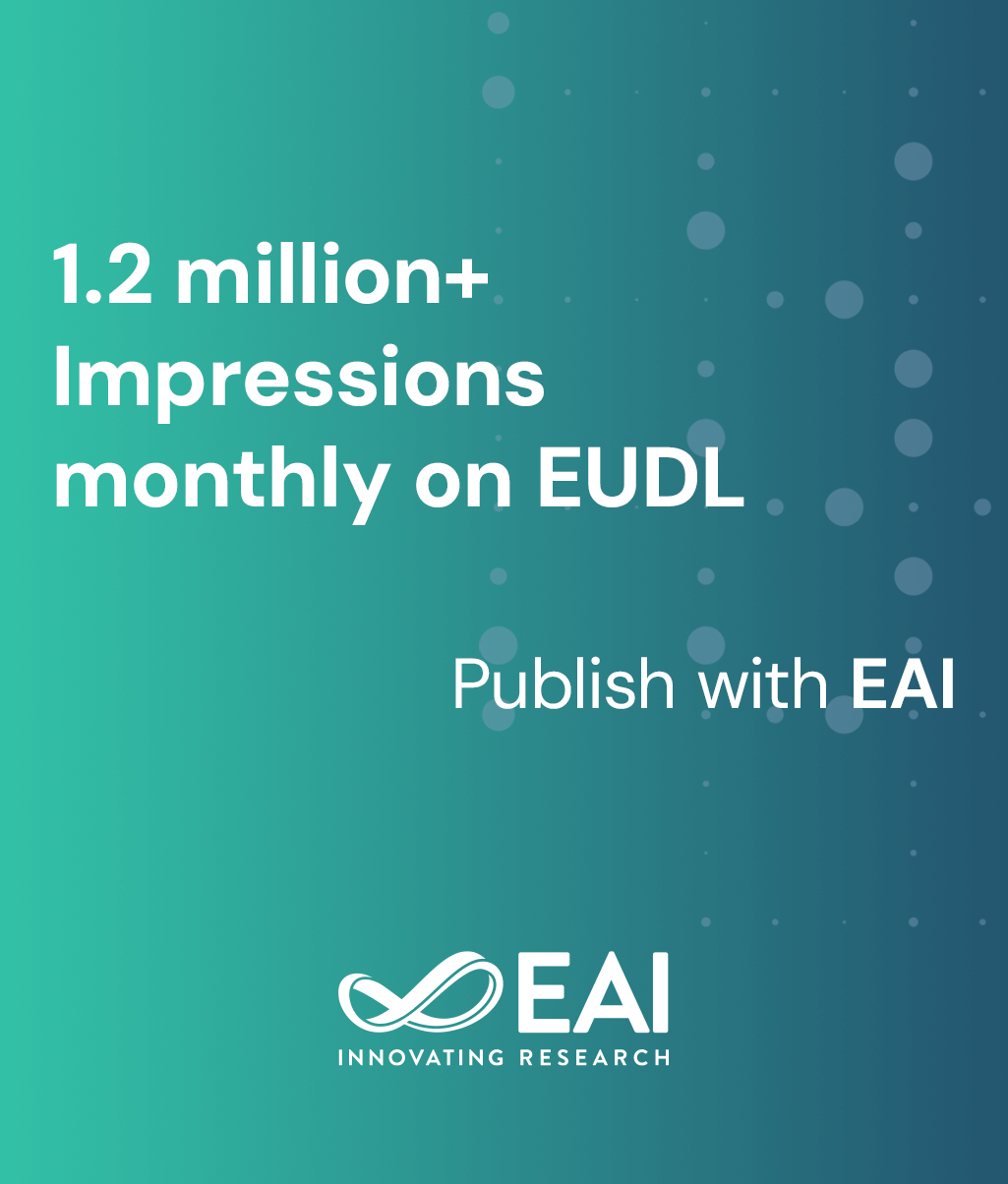
Research Article
Recognition of Periodic Behavioral Patterns from Streaming Mobility Data
@INPROCEEDINGS{10.1007/978-3-319-11569-6_9, author={Mitra Baratchi and Nirvana Meratnia and Paul Havinga}, title={Recognition of Periodic Behavioral Patterns from Streaming Mobility Data}, proceedings={Mobile and Ubiquitous Systems: Computing, Networking, and Services. 10th International Conference, MOBIQUITOUS 2013, Tokyo, Japan, December 2-4, 2013, Revised Selected Papers}, proceedings_a={MOBIQUITOUS}, year={2014}, month={12}, keywords={}, doi={10.1007/978-3-319-11569-6_9} }
- Mitra Baratchi
Nirvana Meratnia
Paul Havinga
Year: 2014
Recognition of Periodic Behavioral Patterns from Streaming Mobility Data
MOBIQUITOUS
Springer
DOI: 10.1007/978-3-319-11569-6_9
Abstract
Ubiquitous location-aware sensing devices have facilitated collection of large volumes of mobility data streams from moving entities such as people and animals, among others. Extraction of various types of periodic behavioral patterns hidden in such large volume of mobility data helps in understanding the dynamics of activities, interactions, and life style of these moving entities. The ever-increasing growth in the volume and dimensionality of such Big Data on the one hand, and the resource constraints of the sensing devices on the other hand, have made not only high pattern recognition accuracy but also low complexity, low resource consumption, and real-timeness important requirements for recognition of patterns from mobility data. In this paper, we propose a method for extracting periodic behavioral patterns from streaming mobility data which fulfills all these requirements. Our experimental results on both synthetic and real data sets confirm superiority of our method compared with existing techniques.