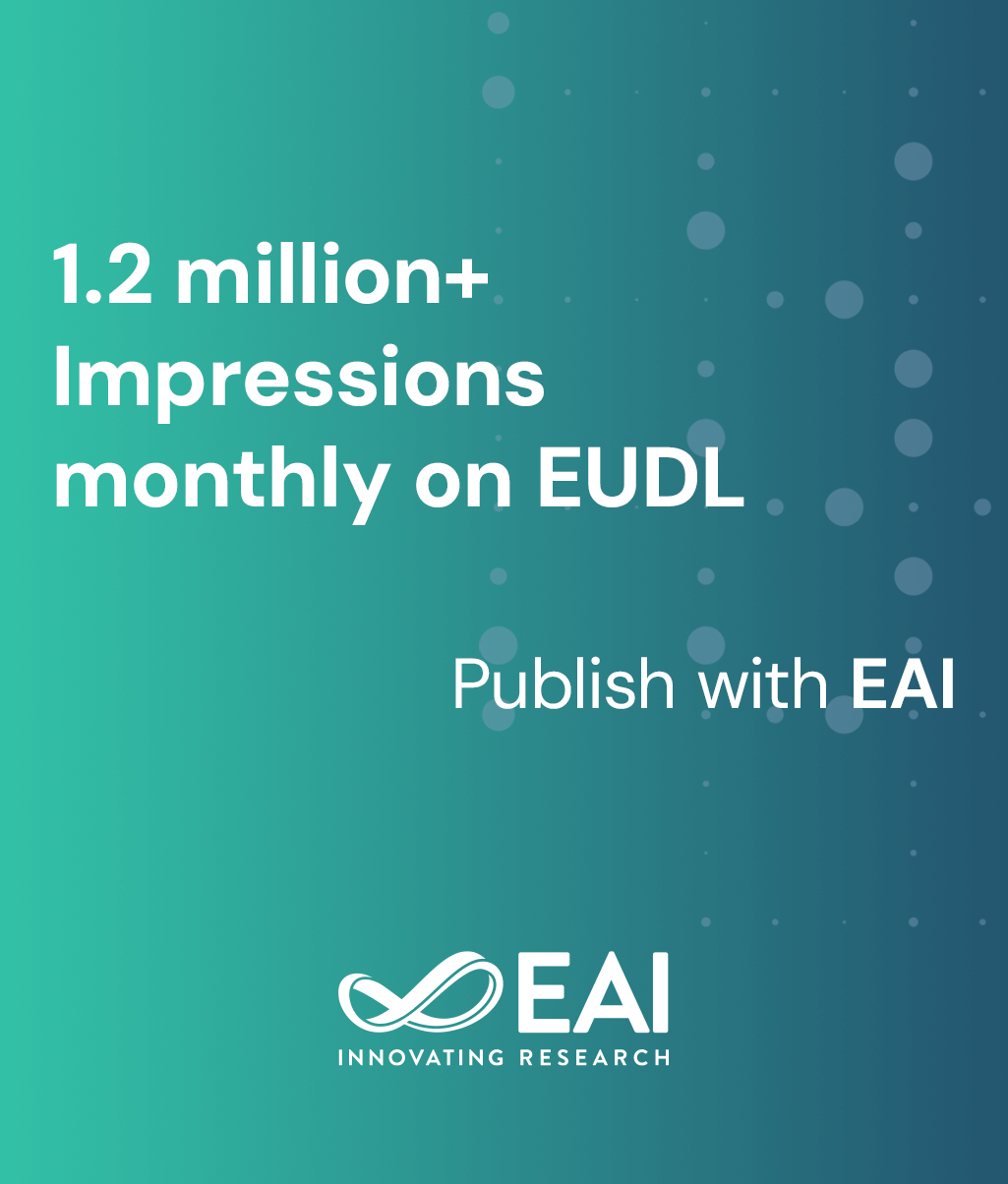
Research Article
Automatic Generation of Malware Threat Intelligence from Unstructured Malware Traces
@INPROCEEDINGS{10.1007/978-3-030-90019-9_3, author={Yuheng Wei and Futai Zou}, title={Automatic Generation of Malware Threat Intelligence from Unstructured Malware Traces}, proceedings={Security and Privacy in Communication Networks. 17th EAI International Conference, SecureComm 2021, Virtual Event, September 6--9, 2021, Proceedings, Part I}, proceedings_a={SECURECOMM}, year={2021}, month={11}, keywords={Malware Threat intelligence Indicators of compromise}, doi={10.1007/978-3-030-90019-9_3} }
- Yuheng Wei
Futai Zou
Year: 2021
Automatic Generation of Malware Threat Intelligence from Unstructured Malware Traces
SECURECOMM
Springer
DOI: 10.1007/978-3-030-90019-9_3
Abstract
Sharing plenty and accurate structured Cyber Threat Intelligence (CTI) will play a pivotal role in adapting to rapidly evolving cyber attacks and malware. However, the traditional CTI generation methods are extremely time and labor-consuming. The recent work focuses on extracting CTI from well structured Open Source Intelligence (OSINT). However, many challenges are still to generate CTI and Indicators of Compromise(IoC) from non-human-written malware traces. This work introduces a method to automatically generate concise, accurate and understandable CTI from unstructured malware traces. For a specific class of malware, we first construct the IoC expressions set from malware traces. Furthermore, we combine the generated IoC expressions and other meaningful information in malware traces to organize the threat intelligence which meets open standards such as Structured Threat Information Expression (STIX). We evaluate our algorithm on real-world dataset. The experimental results show that our method achieves a high average recall rate of 89.4% on the dataset and successfully generates STIX reports for every class of malware, which means our methodology is practical enough to automatically generate effective IoC and CTI.