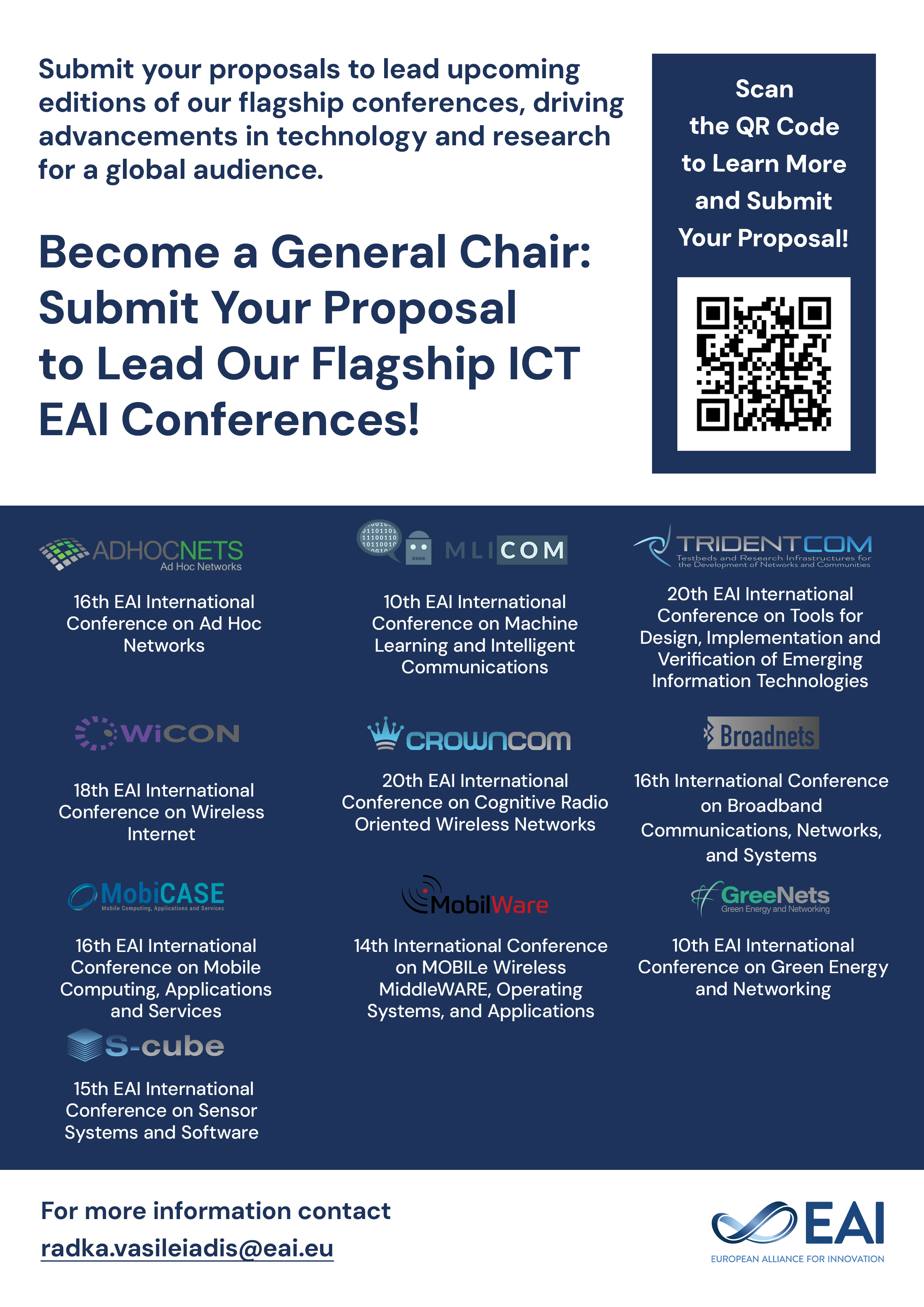
Research Article
TMT-RF: Tunnel Mixed Traffic Classification Based on Random Forest
@INPROCEEDINGS{10.1007/978-3-030-90019-9_21, author={Panpan Zhao and Gaopeng Gou and Chang Liu and Yangyang Guan and Mingxin Cui and Gang Xiong}, title={TMT-RF: Tunnel Mixed Traffic Classification Based on Random Forest}, proceedings={Security and Privacy in Communication Networks. 17th EAI International Conference, SecureComm 2021, Virtual Event, September 6--9, 2021, Proceedings, Part I}, proceedings_a={SECURECOMM}, year={2021}, month={11}, keywords={Traffic classification IPSec tunnel Machine learning}, doi={10.1007/978-3-030-90019-9_21} }
- Panpan Zhao
Gaopeng Gou
Chang Liu
Yangyang Guan
Mingxin Cui
Gang Xiong
Year: 2021
TMT-RF: Tunnel Mixed Traffic Classification Based on Random Forest
SECURECOMM
Springer
DOI: 10.1007/978-3-030-90019-9_21
Abstract
With the explosive growth of the use of tunnels, network anomaly detection and security management are facing huge challenges, of which the first and an important step is tunnel traffic classification. Previous research on the classification of encrypted traffic is mainly based on machine learning methods using statistical features and deep learning methods using packet arrival time and packet length sequence. However, these works mainly focus on the identification of single application traffic. In a real scenario where a single user uses a tunnel, the traffic within a time may contain multiple applications. Due to the tunnel traffic has the same five-tuple, we can’t get the start and end times of each application. Compared with encrypted application traffic classification, it is more difficult to identify applications in tunnels. In this paper, firstly we propose a TMT-RF framework to identify two mixed applications in IPSec tunnels. Then we introduce the first use of NoiseSplit module to split the traffic and then use a CombineBurst module for the second split. Finally, we collected four mixed traffic data sets of three types to evaluate our proposed method. Experimental results demonstrate that TMT-RF not only achieves a splitting accuracy of 93 in positive-time separation applications, but also outperforms other state-of-the-art methods on the data sets for zero-time separation applications and negative-time separation applications.