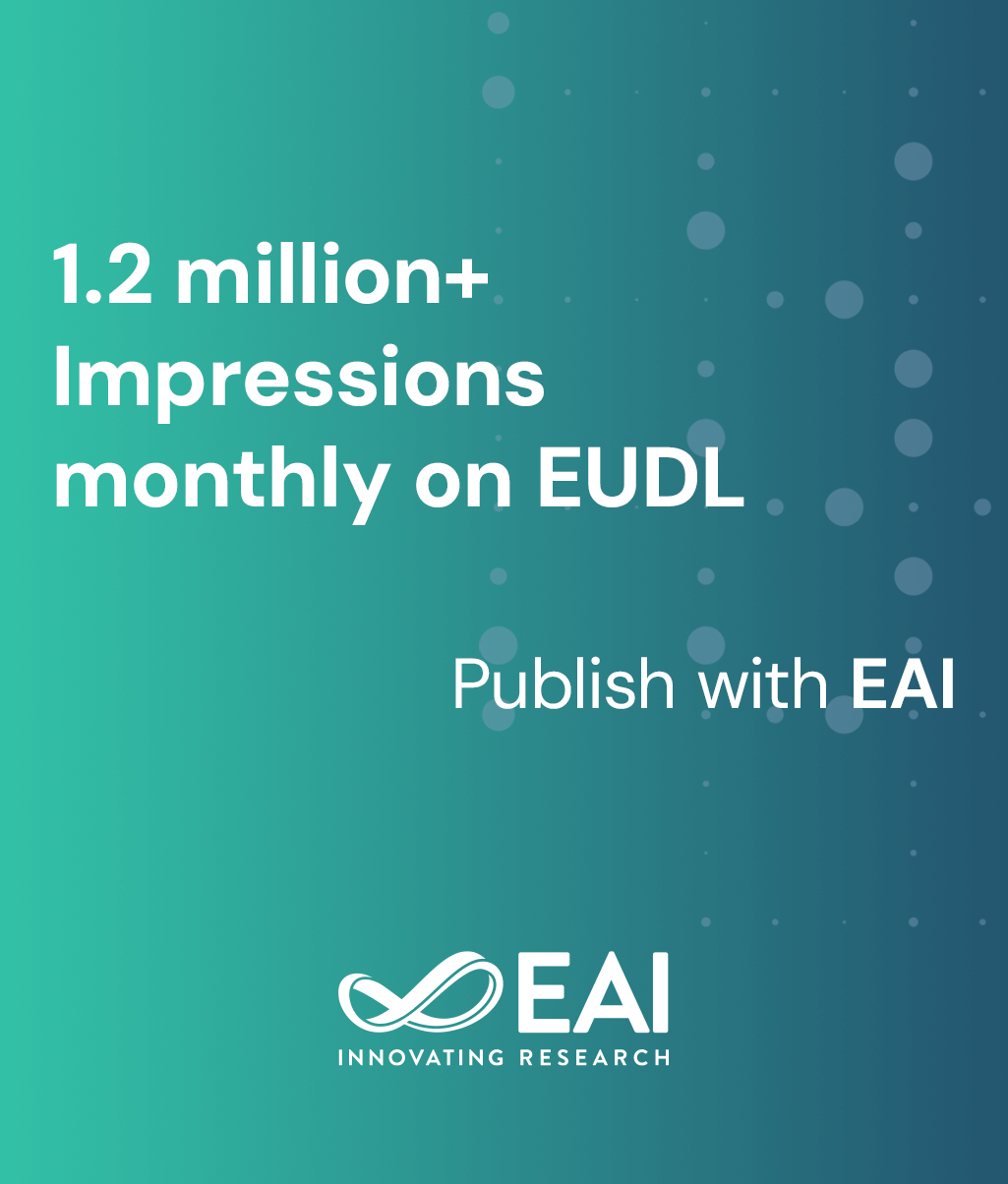
Research Article
Local Model Privacy-Preserving Study for Federated Learning
192 downloads
@INPROCEEDINGS{10.1007/978-3-030-90019-9_15, author={Kaiyun Pan and Daojing He and Chuan Xu}, title={Local Model Privacy-Preserving Study for Federated Learning}, proceedings={Security and Privacy in Communication Networks. 17th EAI International Conference, SecureComm 2021, Virtual Event, September 6--9, 2021, Proceedings, Part I}, proceedings_a={SECURECOMM}, year={2021}, month={11}, keywords={Federated learning Privacy-preserving Distributed optimization Differential privacy}, doi={10.1007/978-3-030-90019-9_15} }
- Kaiyun Pan
Daojing He
Chuan Xu
Year: 2021
Local Model Privacy-Preserving Study for Federated Learning
SECURECOMM
Springer
DOI: 10.1007/978-3-030-90019-9_15
Abstract
In federated learning framework, data are kept locally by clients, which provides naturally a certain level of privacy. However, we show in this paper that a curious onlooker can still infer some sensitive information of clients by looking at the exchanged messages. More precisely, for the linear regression task, the onlooker can decode the exact local model of each client in a constant number of rounds under both cross-device and cross-silo federated learning settings. We improve one of the learning algorithms and experimentally show that it makes the onlooker harder to decode the local model of clients.
Copyright © 2021–2025 ICST