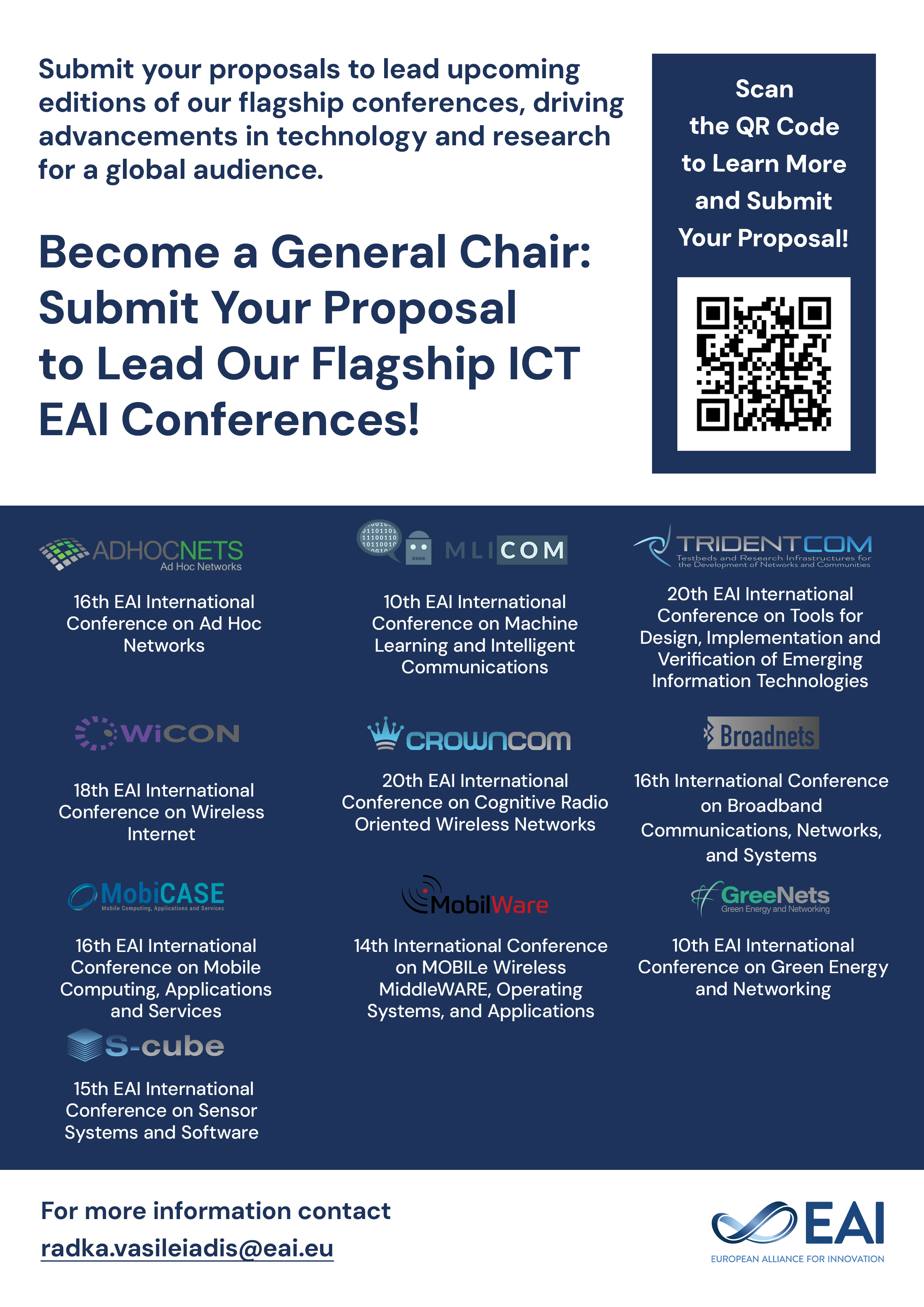
Research Article
Understanding for Differential Privacy in Differencing Attack Scenarios
@INPROCEEDINGS{10.1007/978-3-030-90019-9_10, author={Narges Ashena and Daniele Dell’Aglio and Abraham Bernstein}, title={Understanding for Differential Privacy in Differencing Attack Scenarios}, proceedings={Security and Privacy in Communication Networks. 17th EAI International Conference, SecureComm 2021, Virtual Event, September 6--9, 2021, Proceedings, Part I}, proceedings_a={SECURECOMM}, year={2021}, month={11}, keywords={Differential privacy Parameter tuning Differencing attack}, doi={10.1007/978-3-030-90019-9_10} }
- Narges Ashena
Daniele Dell’Aglio
Abraham Bernstein
Year: 2021
Understanding for Differential Privacy in Differencing Attack Scenarios
SECURECOMM
Springer
DOI: 10.1007/978-3-030-90019-9_10
Abstract
One of the recent notions of privacy protection is Differential Privacy (DP) with potential application in several personal data protection settings. DP acts as an intermediate layer between a private dataset and data analysts introducing privacy by injecting noise into the results of queries. Key to DP is the role of – a parameter that controls the magnitude of injected noise and, therefore, the trade-off between utility and privacy. Choosing proper value is a key challenge and a non-trivial task, as there is no straightforward way to assess the level of privacy loss associated with a given value. In this study, we measure the privacy loss imposed by a given through an adversarial model that exploits auxiliary information. We define the adversarial model and the privacy loss based on a differencing attack and the success probability of such an attack, respectively. Then, we restrict the probability of a successful differencing attack by tuning the . The result is an approach for setting based on the probability of a successful differencing attack and, hence, privacy leak. Our evaluation finds that setting based on some of the approaches presented in related work does not seem to offer adequate protection against the adversarial model introduced in this paper. Furthermore, our analysis shows that the selected by our proposed approach provides privacy protection for the adversary model in this paper and the adversary models in the related work.