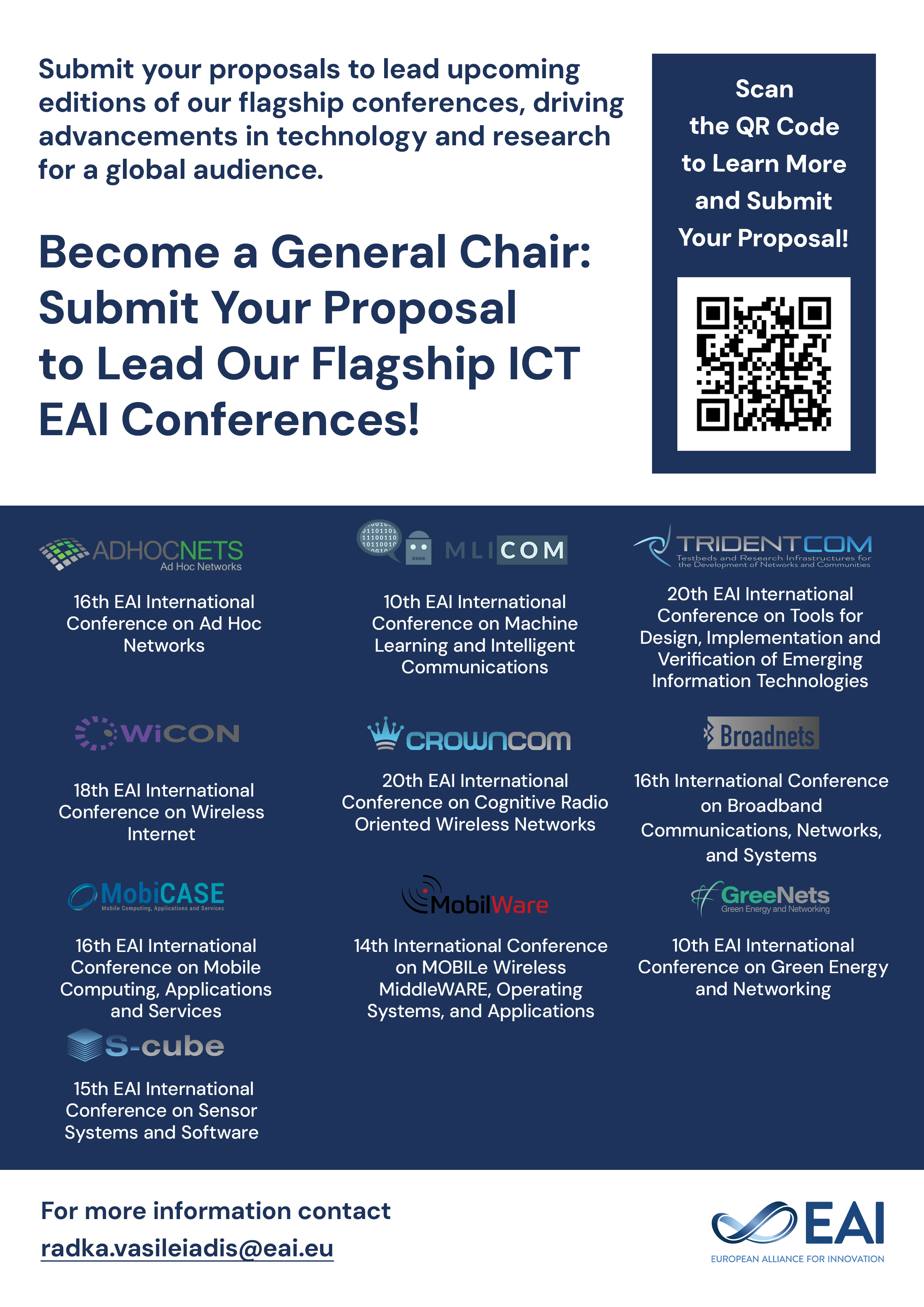
Research Article
Multi-domain Cooperative Service Fault Diagnosis Algorithm Under Network Slicing with Software Defined Networks
@INPROCEEDINGS{10.1007/978-3-030-73562-3_5, author={Wei Li and Yong Dai and Yong Xu and Xilao Wu and Wei Li and Peng Lin}, title={Multi-domain Cooperative Service Fault Diagnosis Algorithm Under Network Slicing with Software Defined Networks}, proceedings={Smart Grid and Innovative Frontiers in Telecommunications. 5th EAI International Conference, SmartGIFT 2020, Chicago, USA, December 12, 2020, Proceedings}, proceedings_a={SMARTGIFT}, year={2021}, month={7}, keywords={SDN network Network slicing Fault diagnosis Management domain}, doi={10.1007/978-3-030-73562-3_5} }
- Wei Li
Yong Dai
Yong Xu
Xilao Wu
Wei Li
Peng Lin
Year: 2021
Multi-domain Cooperative Service Fault Diagnosis Algorithm Under Network Slicing with Software Defined Networks
SMARTGIFT
Springer
DOI: 10.1007/978-3-030-73562-3_5
Abstract
In order to solve the problem of low accuracy of fault diagnosis algorithms in multiple management domain environments such as such as Software Defined Networks (SDN), this paper proposes a multi-domain cooperative service fault diagnosis algorithm under network slice based on the correlation between faults and symptoms. According to the relationship between the management domain and the symptoms, the network resources corresponding to the symptoms are divided into resources within the management domain and inter-domain resources. When constructing a suspected fault set, the suspected fault set is constructed according to the number of simultaneous faults, and the final suspected fault set is determined by calculating the interpretation capability of the suspected fault. Finally, according to Bayesian theory, the fault set with the highest probability is regarded as the most probable fault set. Compared with the existing classical algorithms in the experimental part, it is verified that the algorithm in this paper improves the accuracy of fault diagnosis and reduces the false alarm rate of fault diagnosis.