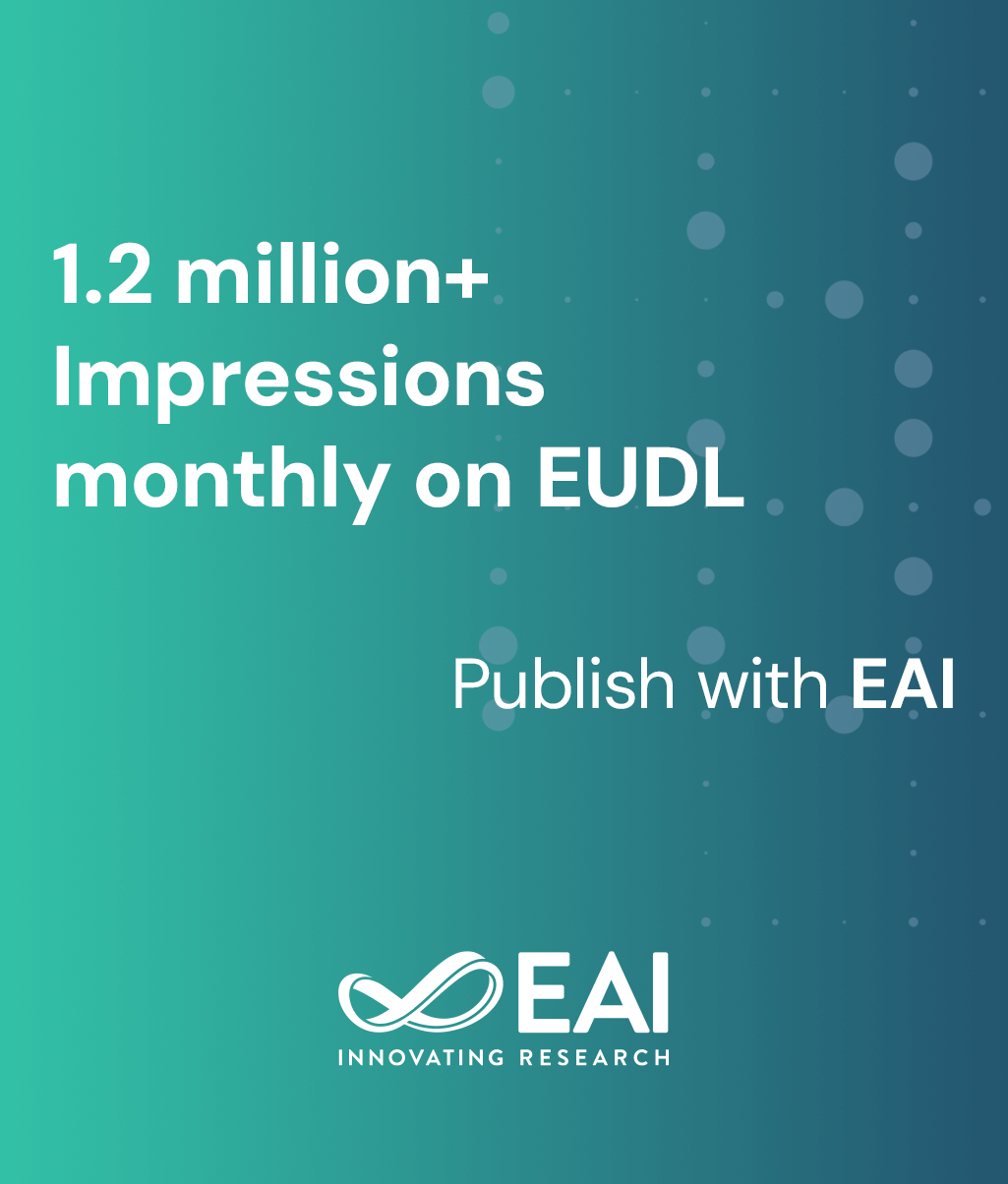
Research Article
A New Similarity Measurement Method for the Power Load Curves Analysis
@INPROCEEDINGS{10.1007/978-3-030-73562-3_1, author={Xin Ning and Ke Zhu and Yuanshi Deng and Rui Zhang and Qi Chen and Zhong Li}, title={A New Similarity Measurement Method for the Power Load Curves Analysis}, proceedings={Smart Grid and Innovative Frontiers in Telecommunications. 5th EAI International Conference, SmartGIFT 2020, Chicago, USA, December 12, 2020, Proceedings}, proceedings_a={SMARTGIFT}, year={2021}, month={7}, keywords={Power load curves Similarity measurement Euclidean distance Cluster analysis}, doi={10.1007/978-3-030-73562-3_1} }
- Xin Ning
Ke Zhu
Yuanshi Deng
Rui Zhang
Qi Chen
Zhong Li
Year: 2021
A New Similarity Measurement Method for the Power Load Curves Analysis
SMARTGIFT
Springer
DOI: 10.1007/978-3-030-73562-3_1
Abstract
In order to improve the quality of the power load curves similarity measurement, a new similarity measurement method based on Euclidean distance is proposed in this paper . Among the commonly used similarity measurement methods, Euclidean distance is not sensitive to the fluctuation of the load curves, which results in the lack of shape measurement capability. For the numerical distribution on the timeline is not concerned, the dynamic time warping (DTW) distance is not accord with the requirement of the power system load analysis. Focus on those issues, the proposed method introduced a correction factor that contains the dynamic characteristics of the numerical difference between two power load curves without compromising time warping. The advantages and performance of the proposed method are evaluated by similarity computing and clustering analysis. As shown in the experimental results of similarity computing, the proposed method performs as same as ED and DTW, but the calculating time is less than DTW. In the clustering analysis, it also decreases the calculating time from 3.9 s to 0.595 s compared with DTW and shows better clustering effect that make the Davies-Bouldin index from 0.438 for ED and 0.325 for DTW to 0.249.