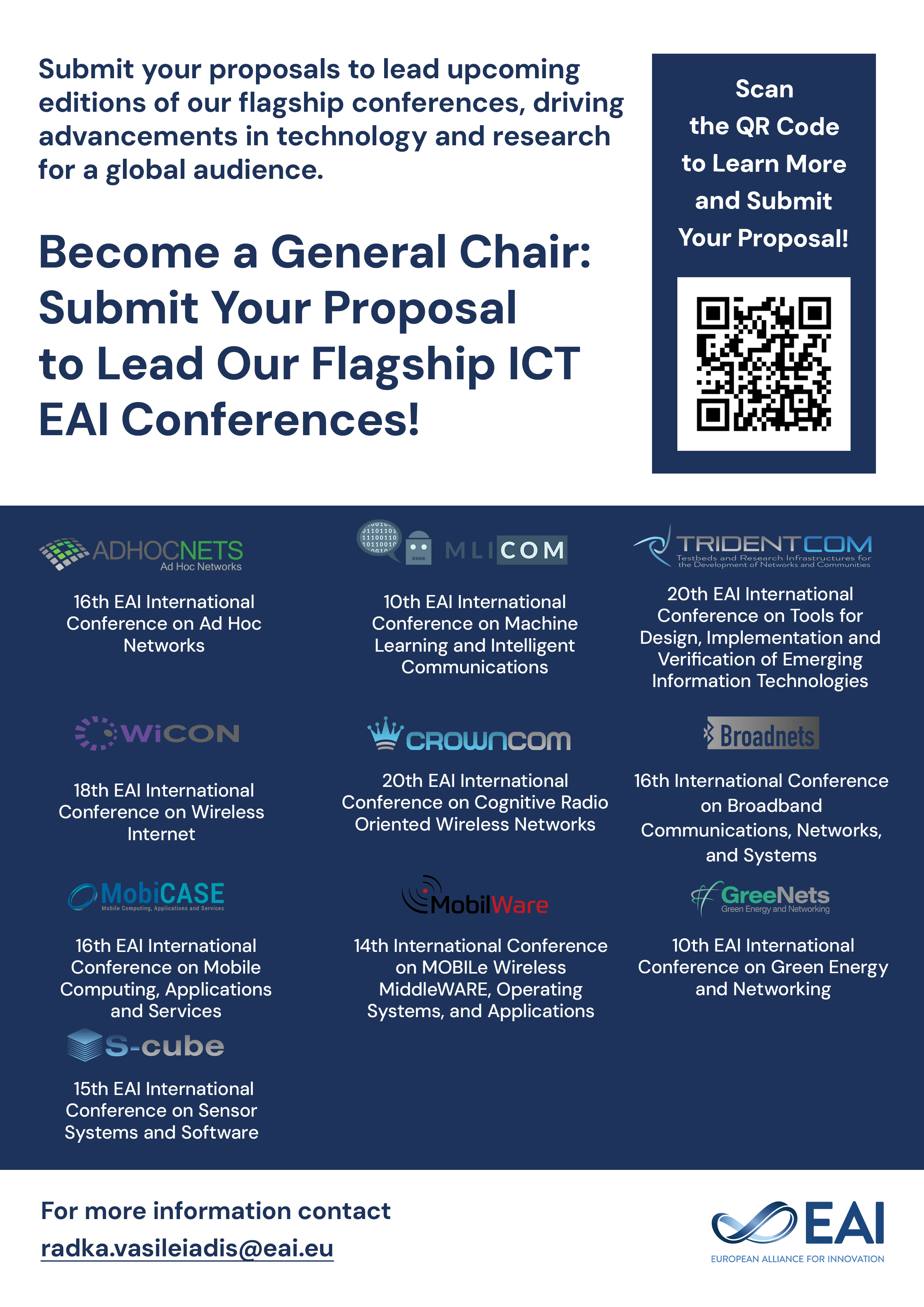
Research Article
Introducing and Benchmarking a One-Shot Learning Gesture Recognition Dataset
@INPROCEEDINGS{10.1007/978-3-030-72802-1_8, author={Panagiotis Kasnesis and Christos Chatzigeorgiou and Charalampos Z. Patrikakis and Maria Rangoussi}, title={Introducing and Benchmarking a One-Shot Learning Gesture Recognition Dataset}, proceedings={Big Data Technologies and Applications. 10th EAI International Conference, BDTA 2020, and 13th EAI International Conference on Wireless Internet, WiCON 2020, Virtual Event, December 11, 2020, Proceedings}, proceedings_a={BDTA \& WICON}, year={2021}, month={7}, keywords={Datasets Deep learning Wearable gesture recognition One-shot learning}, doi={10.1007/978-3-030-72802-1_8} }
- Panagiotis Kasnesis
Christos Chatzigeorgiou
Charalampos Z. Patrikakis
Maria Rangoussi
Year: 2021
Introducing and Benchmarking a One-Shot Learning Gesture Recognition Dataset
BDTA & WICON
Springer
DOI: 10.1007/978-3-030-72802-1_8
Abstract
Deep learning techniques have been widely and successfully applied, over the last five years, to recognize the gestures and activities performed by users wearing electronic devices. However, the collected datasets are built in an old fashioned way, mostly comprised of subjects that perform many times few different gestures/activities. This paper addresses the lack of a wearable gesture recognition dataset for exploring one-shot learning techniques. The current dataset consists of 46 gestures performed by 35 subjects, wearing a smartwatch equipped with 3 motion sensors and is publicly available. Moreover, 3 one-shot learning classification approaches are benchmarked on the dataset, exploiting two different deep learning classifiers. The results of the benchmark depict the difficulty of the one-shot learning task, exposing new challenges for wearable gesture/activity recognition.