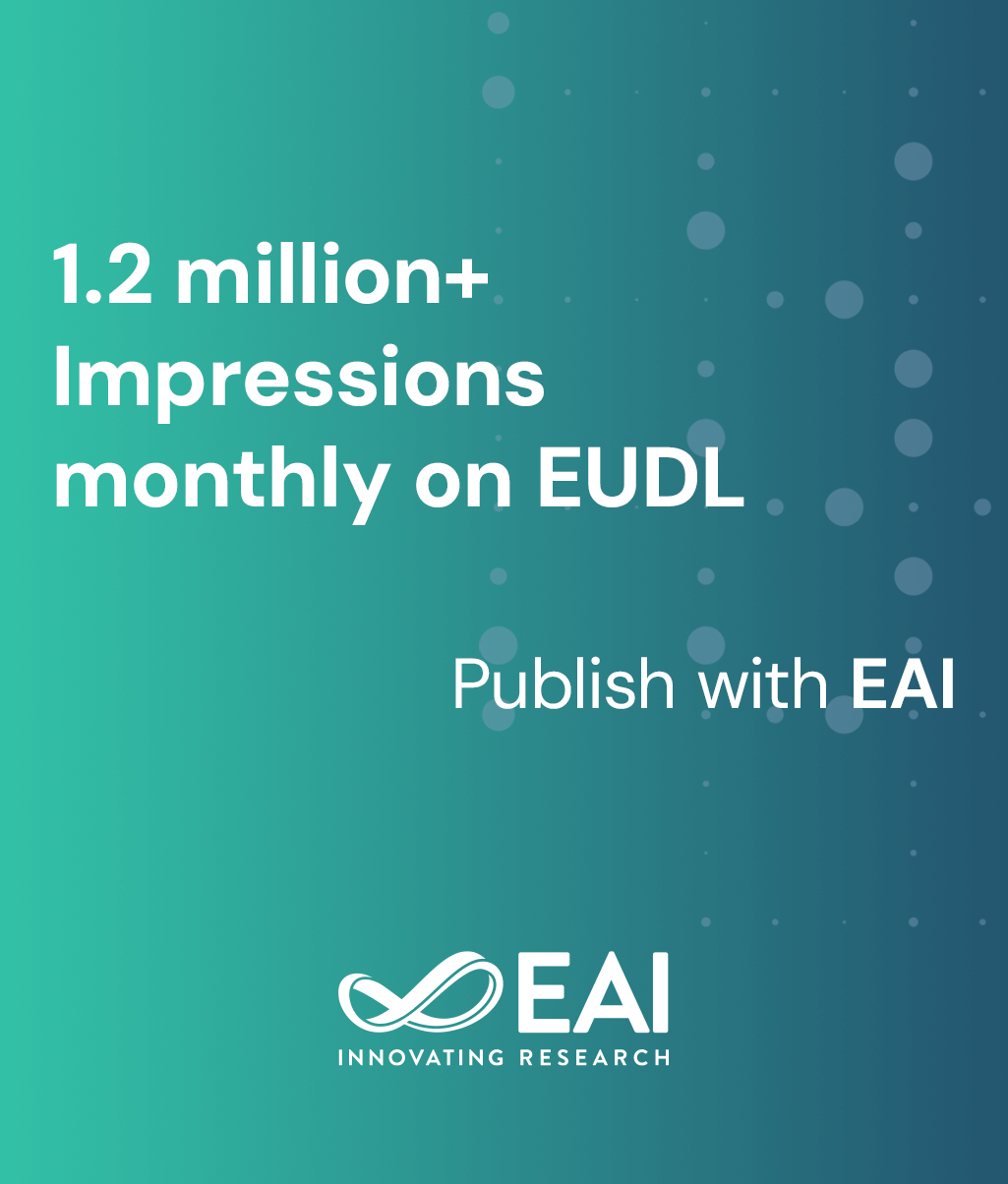
Research Article
Performance Evaluation of Energy Detection, Matched Filtering and KNN Under Different Noise Models
@INPROCEEDINGS{10.1007/978-3-030-72802-1_10, author={Xiaoyan Wang and Jingjing Yang and Tengye Yu and Rui Li and Ming Huang}, title={Performance Evaluation of Energy Detection, Matched Filtering and KNN Under Different Noise Models}, proceedings={Big Data Technologies and Applications. 10th EAI International Conference, BDTA 2020, and 13th EAI International Conference on Wireless Internet, WiCON 2020, Virtual Event, December 11, 2020, Proceedings}, proceedings_a={BDTA \& WICON}, year={2021}, month={4}, keywords={Spectrum security Radio monitoring Noise uncertainty}, doi={10.1007/978-3-030-72802-1_10} }
- Xiaoyan Wang
Jingjing Yang
Tengye Yu
Rui Li
Ming Huang
Year: 2021
Performance Evaluation of Energy Detection, Matched Filtering and KNN Under Different Noise Models
BDTA & WICON
Springer
DOI: 10.1007/978-3-030-72802-1_10
Abstract
Due to the broadcast nature of radio transmission, both authorized and unauthorized users can access the network, which leads to the increasingly prominent security problems of wireless network. At the same time, it is more difficult to detect and identify users in wireless network environment due to the influence of noise. In this paper, the performance of energy detection (ED), matched filtering (MF) and K-nearest neighbor algorithm (KNN) are analyzed under different noise and uncertain noise separately. The Gaussian noise, α-stable distribution noise and Laplace distribution noise models are simulated respectively under the different uncertainty of noise when the false alarm probability is 0.01. The results show that the performance of the detectors is significantly affected by different noise models. In any case, the detection probability of KNN algorithm is the highest; the performance of MF is much better than ED under different noise models; KNN is not sensitive to noise uncertainty; MF has better performance on noise uncertainty which makes ED performance decline fleetly.