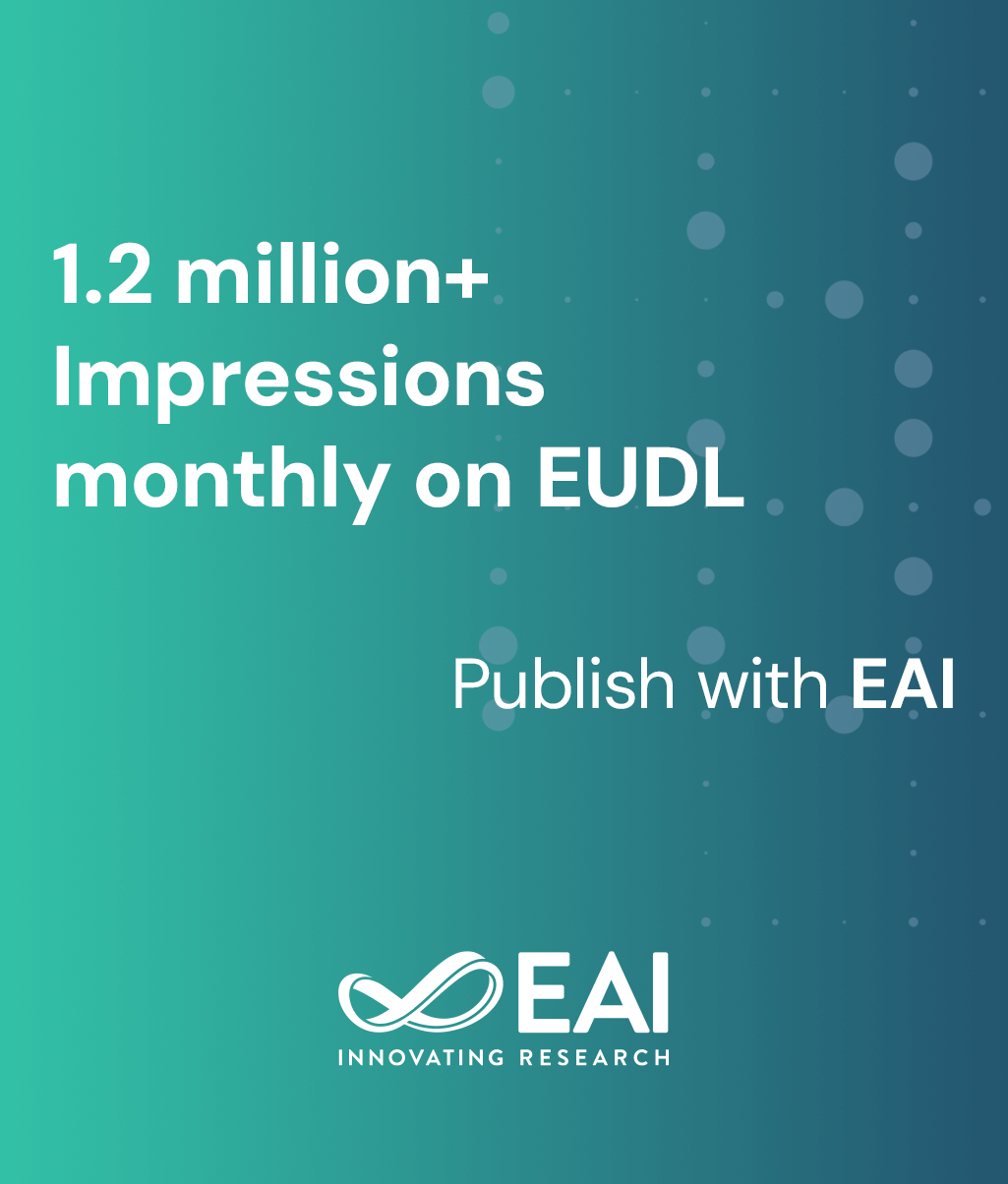
Research Article
Constructing Knowledge Graph for Prognostics and Health Management of On-board Train Control System Based on Big Data and XGBoost
@INPROCEEDINGS{10.1007/978-3-030-72802-1_1, author={Jiang Liu and Bai-gen Cai and Zhong-bin Guo and Xiao-lin Zhao}, title={Constructing Knowledge Graph for Prognostics and Health Management of On-board Train Control System Based on Big Data and XGBoost}, proceedings={Big Data Technologies and Applications. 10th EAI International Conference, BDTA 2020, and 13th EAI International Conference on Wireless Internet, WiCON 2020, Virtual Event, December 11, 2020, Proceedings}, proceedings_a={BDTA \& WICON}, year={2021}, month={7}, keywords={Knowledge graph Prognostics and health management Train control system Big data Machine learning Extreme gradient boosting}, doi={10.1007/978-3-030-72802-1_1} }
- Jiang Liu
Bai-gen Cai
Zhong-bin Guo
Xiao-lin Zhao
Year: 2021
Constructing Knowledge Graph for Prognostics and Health Management of On-board Train Control System Based on Big Data and XGBoost
BDTA & WICON
Springer
DOI: 10.1007/978-3-030-72802-1_1
Abstract
Train control system plays a significant role in safe and efficient operation of the railway transport system. In order to enhance the system capability and cost efficiency from a full life cycle perspective, the establishment of a Condition-based Maintenance (CBM) scheme will be beneficial to both the currently in use and next generation train control systems. Due to the complexity of the fault mechanism of on-board train control system, a data-driven method is of great necessity to enable the Prognostics and Health Management (PHM) for the equipments in field operation. In this paper, we propose a big data platform to realize the storage, management and processing of historical field data from on-board train control equipments. Specifically, we focus on constructing the Knowledge Graph (KG) of typical faults. The Extreme Gradient Boosting (XGBoost) method is adopted to build big-data-enabled training models, which reveal the distribution of the feature importance and quantitatively evaluate the fault correlation of all related features. The presented scheme is demonstrated by a big data platform with incremental field data sets from railway operation process. Case study results show that this scheme can derive knowledge graph of specific system fault and reveal the relevance of features effectively.