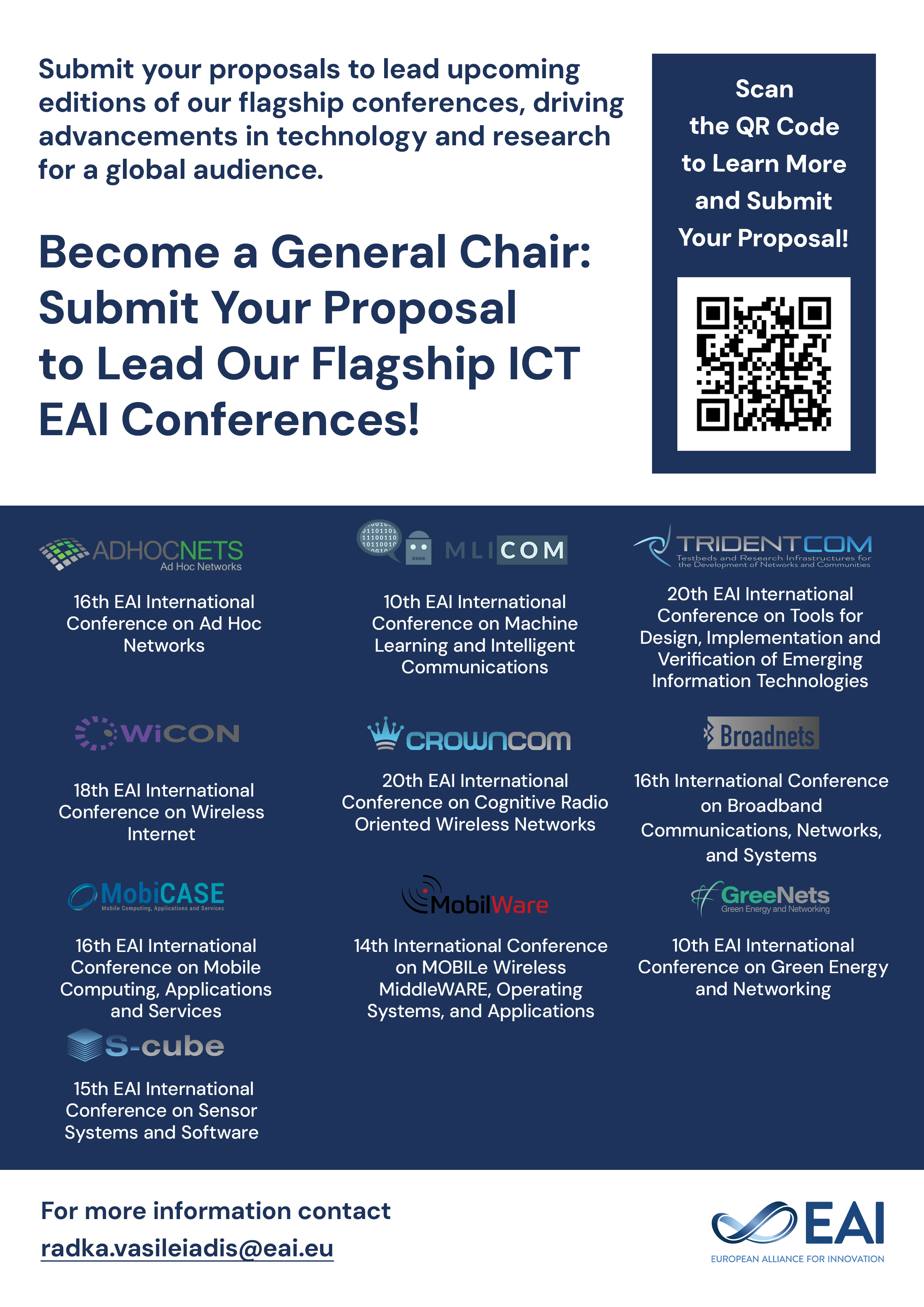
Research Article
An Intelligent Predictive Maintenance Approach Based on End-of-Line Test Logfiles in the Automotive Industry
@INPROCEEDINGS{10.1007/978-3-030-71061-3_8, author={David Vic\"{e}ncio and Hugo Silva and Salviano Soares and V\^{\i}tor Filipe and Ant\^{o}nio Valente}, title={An Intelligent Predictive Maintenance Approach Based on End-of-Line Test Logfiles in the Automotive Industry}, proceedings={Industrial IoT Technologies and Applications. 4th EAI International Conference, Industrial IoT 2020, Virtual Event, December 11, 2020, Proceedings}, proceedings_a={INDUSTRIALIOT}, year={2021}, month={7}, keywords={End-of-Line Testing Systems (EOL) Service monitoring and log operation data Industry 4.0 Predictive Maintenance (PdM) Machine learning (ML)}, doi={10.1007/978-3-030-71061-3_8} }
- David Vicêncio
Hugo Silva
Salviano Soares
Vítor Filipe
António Valente
Year: 2021
An Intelligent Predictive Maintenance Approach Based on End-of-Line Test Logfiles in the Automotive Industry
INDUSTRIALIOT
Springer
DOI: 10.1007/978-3-030-71061-3_8
Abstract
Through technological advents from Industry 4.0 and the Internet of Things, as well as new Big Data solutions, predictive maintenance begins to play a strategic role in the increasing operational performance of any industrial facility. Equipment failures can be very costly and have catastrophic consequences. In its basic concept, Predictive maintenance allows minimizing equipment faults or service disruptions, presenting promising cost savings. This paper presents a data-driven approach, based on multiple-instance learning, to predict malfunctions in End-of-Line Testing Systems through the extraction of operational logs, which, while not designed to predict failures, contains valid information regarding their operational mode over time. For the case study performed, a real-life dataset was used containing thousands of log messages, collected in a real automotive industry environment. The insights gained from mining this type of data will be shared in this paper, highlighting the main challenges and benefits, as well as good recommendations, and best practices for the appropriate usage of machine learning techniques and analytics tools that can be implemented in similar industrial environments.