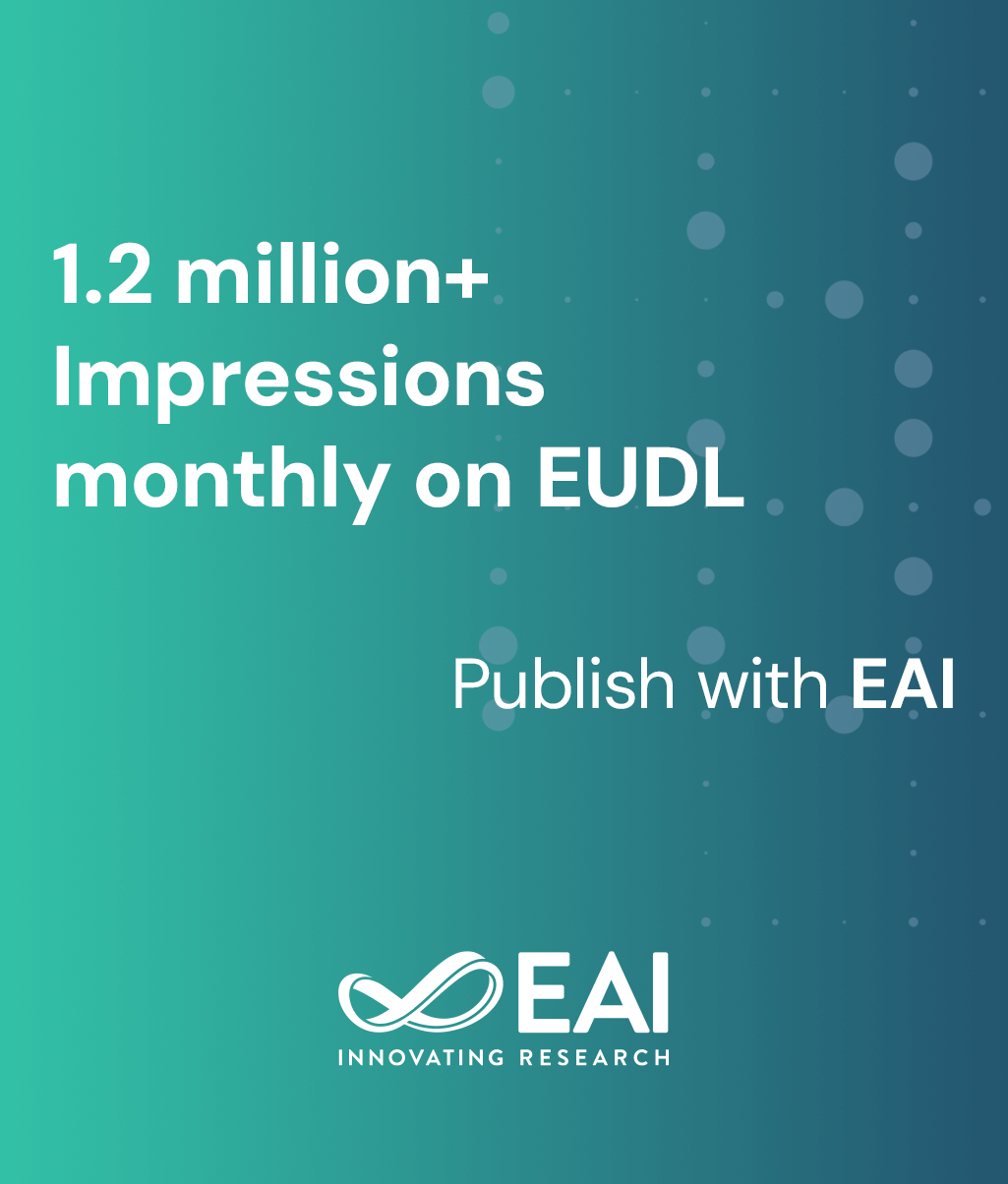
Research Article
Crowd Anomaly Detection Based on Elevator Internet of Things Technology
@INPROCEEDINGS{10.1007/978-3-030-71061-3_1, author={Chunhua Jia and Wenhai Yi and Yu Wu and Zhuang Li and Shuai Zhu and Leilei Wu}, title={Crowd Anomaly Detection Based on Elevator Internet of Things Technology}, proceedings={Industrial IoT Technologies and Applications. 4th EAI International Conference, Industrial IoT 2020, Virtual Event, December 11, 2020, Proceedings}, proceedings_a={INDUSTRIALIOT}, year={2021}, month={7}, keywords={IoT Anomaly Computer Vision Machine learning Big Data Cloud computing}, doi={10.1007/978-3-030-71061-3_1} }
- Chunhua Jia
Wenhai Yi
Yu Wu
Zhuang Li
Shuai Zhu
Leilei Wu
Year: 2021
Crowd Anomaly Detection Based on Elevator Internet of Things Technology
INDUSTRIALIOT
Springer
DOI: 10.1007/978-3-030-71061-3_1
Abstract
A work-flow which aims at capturing residents’ abnormal activities through the passenger flow of elevator in multi-storey residence buildings is presented in this paper. Firstly, sensors (hall sensor, photoelectric sensor, gyro, accelerometer, barometer, and thermometer) connected with internet are mounted in elevator to collect image and data. Then computer vision algorithms such as instance segmentation, multi-label recognition, embedding and clustering are applied to generalize passenger flow of elevator, i.e. how many people and what kinds of people get in and out of the elevator on each floor. More specifically so-called GraftNet is proposed for fine-grained multi-label recognition task to recognize human attributes (e.g. gender, age, appearance, and occupation). Thirdly, based on the passenger flow data, anomaly detection of unsupervised learning is hierarchically applied to detect abnormal or even illegal activities of the residents. Meanwhile, based on manual reviewed data, Catboost algorithm is implemented for multi-classification task. Experiment shows the work-flow proposed in this paper can detect the anomaly and classify different categories well.