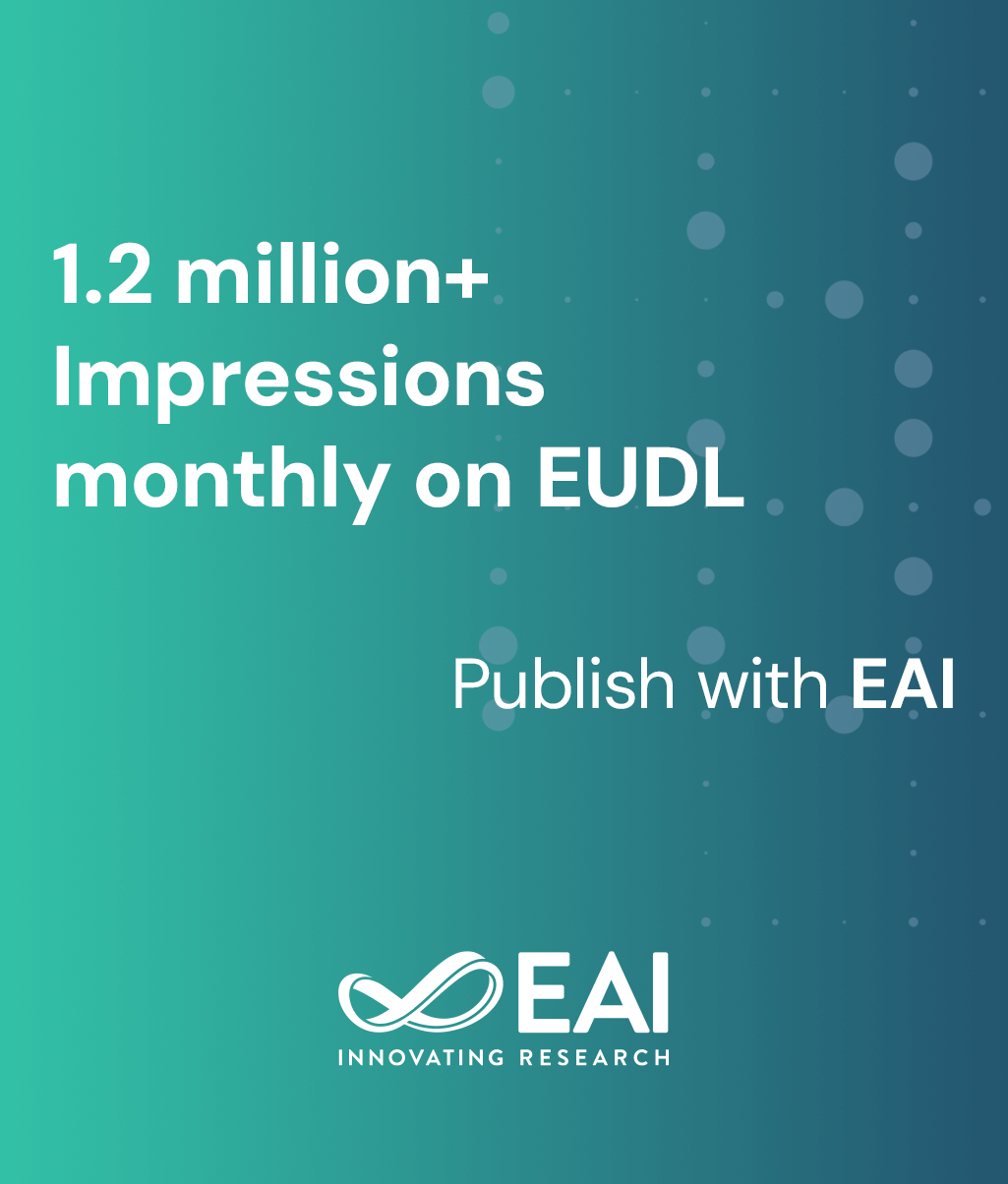
Research Article
Indoor Localization with Filtered and Corrected Calibration RSSI
@INPROCEEDINGS{10.1007/978-3-030-70572-5_4, author={Madikana S. Sediela and Moses L. Gadebe and Okuthe P. Kogeda}, title={Indoor Localization with Filtered and Corrected Calibration RSSI}, proceedings={Towards new e-Infrastructure and e-Services for Developing Countries. 12th EAI International Conference, AFRICOMM 2020, Eb\'{e}ne City, Mauritius, December 2-4, 2020, Proceedings}, proceedings_a={AFRICOMM}, year={2021}, month={7}, keywords={Fingerprinting Mean Standard deviation RSSI GPS Wi-Fi}, doi={10.1007/978-3-030-70572-5_4} }
- Madikana S. Sediela
Moses L. Gadebe
Okuthe P. Kogeda
Year: 2021
Indoor Localization with Filtered and Corrected Calibration RSSI
AFRICOMM
Springer
DOI: 10.1007/978-3-030-70572-5_4
Abstract
Nowadays Location-Based Services (LBS) applications are proposed and presented in literature because of high growth in wireless sensor networks. The LBS provide useful information about the person or object’s current position. Among these applications, Global Positioning System (GPS)-based positioning and navigation services have been deployed in an outdoor environment. However, GPS requires being in sight with satellites due to line of sight challenge. The GPS fails in an indoor environment because of multipath effects caused by walls or indoor setup in general. Meanwhile, Wi-Fi-based positioning system are being proposed in literature and most of them utilizes the fingerprinting algorithm. Whereby, measurements of Received Signal Strength Indicator (RSSI) are collected during the offline phase for radio map and positioning is performed during the online phase. Similarly, this approach is faced with signal attenuation challenge caused by walls, desks, moving people, or just the indoor setup in general. The collection of the signal strengths without correction can affect positioning accuracy. In this paper, we present a model that utilizes Mean and Standard Deviation to identify and correct unstable low RSSI outliers during offline phase of Fingerprinting algorithm. We conducted comparative performance of Machine learning classification algorithms based on corrected RSSI and measured RSSI. The simulation results performed in MATLAB, indicates that correcting the RSSI improves the accuracy, precision, and recall of Fine Tree, Coarse Decision Tree, and Kernel Naïve Bayes to 95.1%, 94.8% and 94.5% respectively.