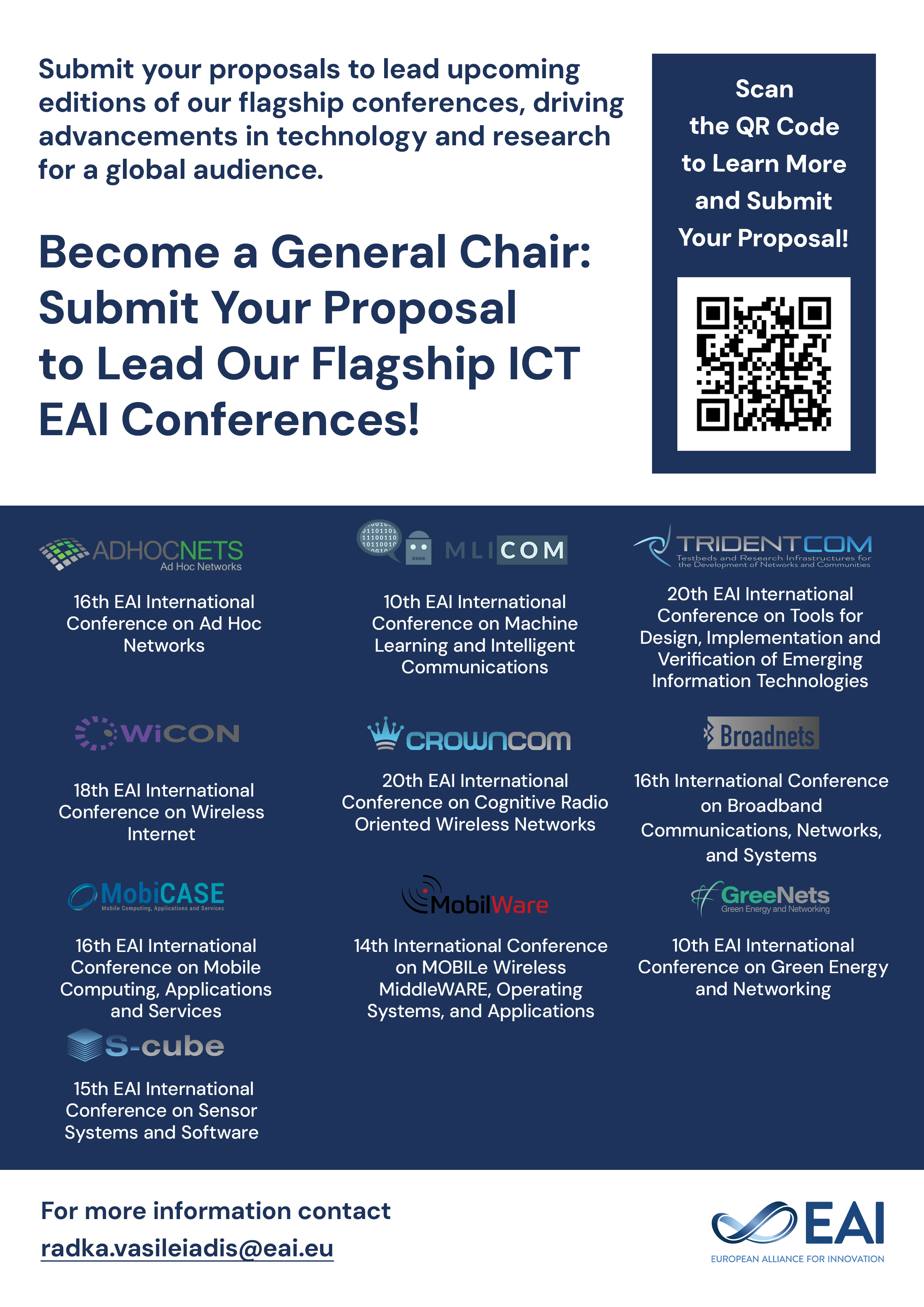
Research Article
Deep-Learning-Based Feature Encoding of Clinical Parameters for Patient Specific CTA Dose Optimization
@INPROCEEDINGS{10.1007/978-3-030-70569-5_20, author={Marja Fleitmann and Hristina Uzunova and Andreas Martin Stroth and Jan Gerlach and Alexander F\'{y}rschke and J\o{}rg Barkhausen and Arpad Bischof and Heinz Handels}, title={Deep-Learning-Based Feature Encoding of Clinical Parameters for Patient Specific CTA Dose Optimization}, proceedings={Wireless Mobile Communication and Healthcare. 9th EAI International Conference, MobiHealth 2020, Virtual Event, November 19, 2020, Proceedings}, proceedings_a={MOBIHEALTH}, year={2021}, month={7}, keywords={Feature encoding Deep Learning Case-based reasoning Contrast agent}, doi={10.1007/978-3-030-70569-5_20} }
- Marja Fleitmann
Hristina Uzunova
Andreas Martin Stroth
Jan Gerlach
Alexander Fürschke
Jörg Barkhausen
Arpad Bischof
Heinz Handels
Year: 2021
Deep-Learning-Based Feature Encoding of Clinical Parameters for Patient Specific CTA Dose Optimization
MOBIHEALTH
Springer
DOI: 10.1007/978-3-030-70569-5_20
Abstract
The use of contrast agents in CT angiography examinations holds a potential health risk for the patient. Despite this, often unintentionally an excessive contrast agent dose is administered. Our goal is to provide a support system for the medical practitioner that advises to adjust an individually adapted dose. We propose a comparison between different means of feature encoding techniques to gain a higher accuracy when recommending the dose adjustment. We apply advanced deep learning approaches and standard methods like principle component analysis to encode high dimensional parameter vectors in a low dimensional feature space. Our experiments showed that features encoded by a regression neural network provided the best results. Especially with a focus on the 90% precision for the “excessive dose” class meaning that if our system classified a case as “excessive dose” the ground truth is most likely accordingly. With that in mind a recommendation for a lower dose could be administered without the risk of insufficient contrast and therefore a repetition of the CT angiography examination. In conclusion we showed that Deep-Learning-based feature encoding on clinical parameters is advantageous for our aim to prevent excessive contrast agent doses.