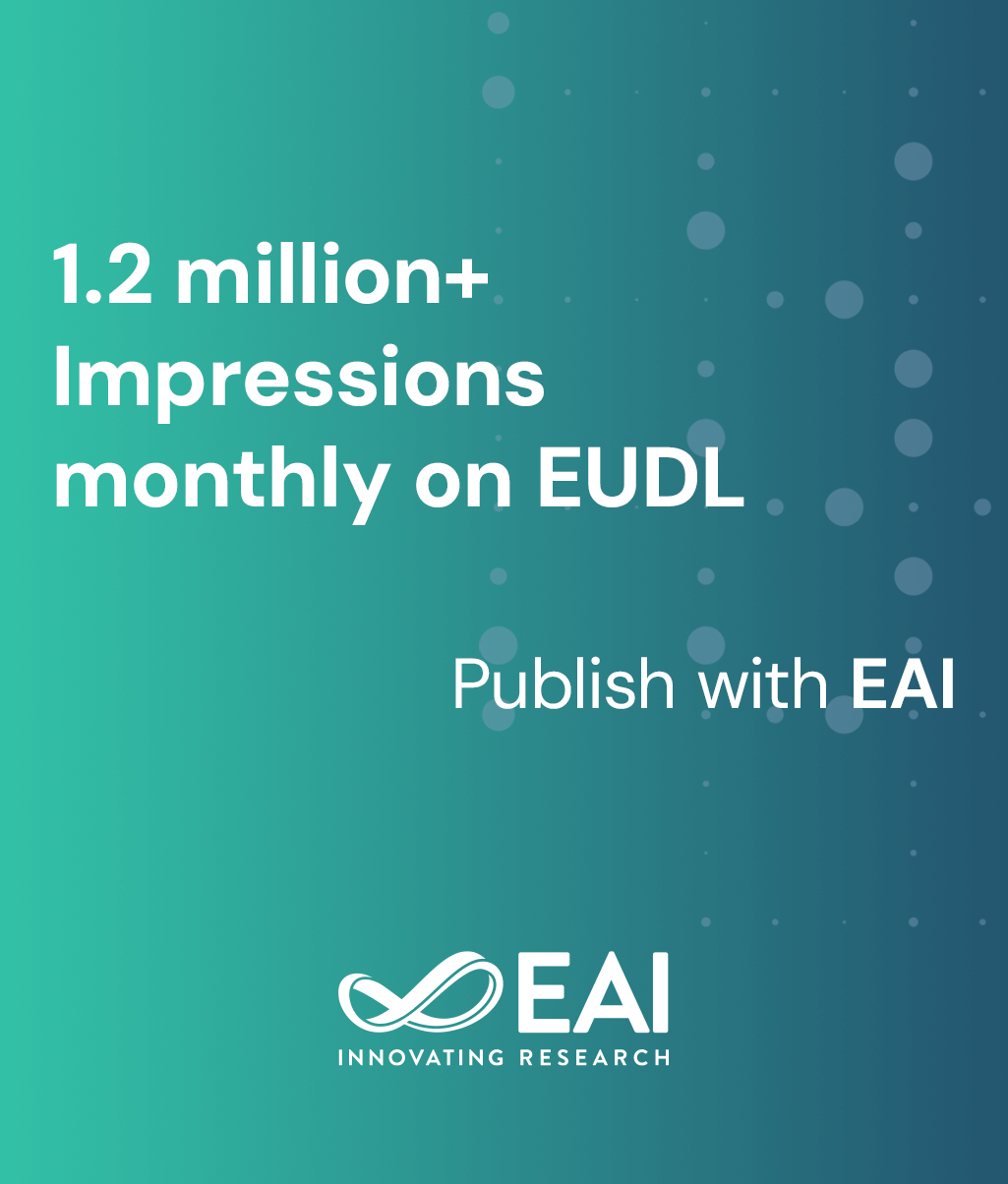
Research Article
Patient-Independent Schizophrenia Relapse Prediction Using Mobile Sensor Based Daily Behavioral Rhythm Changes
@INPROCEEDINGS{10.1007/978-3-030-70569-5_2, author={Bishal Lamichhane and Dror Ben-Zeev and Andrew Campbell and Tanzeem Choudhury and Marta Hauser and John Kane and Mikio Obuchi and Emily Scherer and Megan Walsh and Rui Wang and Weichen Wang and Akane Sano}, title={Patient-Independent Schizophrenia Relapse Prediction Using Mobile Sensor Based Daily Behavioral Rhythm Changes}, proceedings={Wireless Mobile Communication and Healthcare. 9th EAI International Conference, MobiHealth 2020, Virtual Event, November 19, 2020, Proceedings}, proceedings_a={MOBIHEALTH}, year={2021}, month={7}, keywords={Mobile sensing Ubiquitous computing Schizophrenia Relapse prediction}, doi={10.1007/978-3-030-70569-5_2} }
- Bishal Lamichhane
Dror Ben-Zeev
Andrew Campbell
Tanzeem Choudhury
Marta Hauser
John Kane
Mikio Obuchi
Emily Scherer
Megan Walsh
Rui Wang
Weichen Wang
Akane Sano
Year: 2021
Patient-Independent Schizophrenia Relapse Prediction Using Mobile Sensor Based Daily Behavioral Rhythm Changes
MOBIHEALTH
Springer
DOI: 10.1007/978-3-030-70569-5_2
Abstract
A schizophrenia relapse has severe consequences for a patient’s health, work, and sometimes even life safety. If an oncoming relapse can be predicted on time, for example by detecting early behavioral changes in patients, then interventions could be provided to prevent the relapse. In this work, we investigated a machine learning based schizophrenia relapse prediction model using mobile sensing data to characterize behavioral features. A patient-independent model providing sequential predictions, closely representing the clinical deployment scenario for relapse prediction, was evaluated. The model uses the mobile sensing data from the recent four weeks to predict an oncoming relapse in the next week. We used the behavioral rhythm features extracted from daily templates of mobile sensing data, self-reported symptoms collected via EMA (Ecological Momentary Assessment), and demographics to compare different classifiers for the relapse prediction. Naive Bayes based model gave the best results with an F2 score of 0.083 when evaluated in a dataset consisting of 63 schizophrenia patients, each monitored for up to a year. The obtained F2 score, though low, is better than the baseline performance of random classification (F2 score of 0.02 ± 0.024). Thus, mobile sensing has predictive value for detecting an oncoming relapse and needs further investigation to improve the current performance. Towards that end, further feature engineering and model personalization based on the behavioral idiosyncrasies of a patient could be helpful.