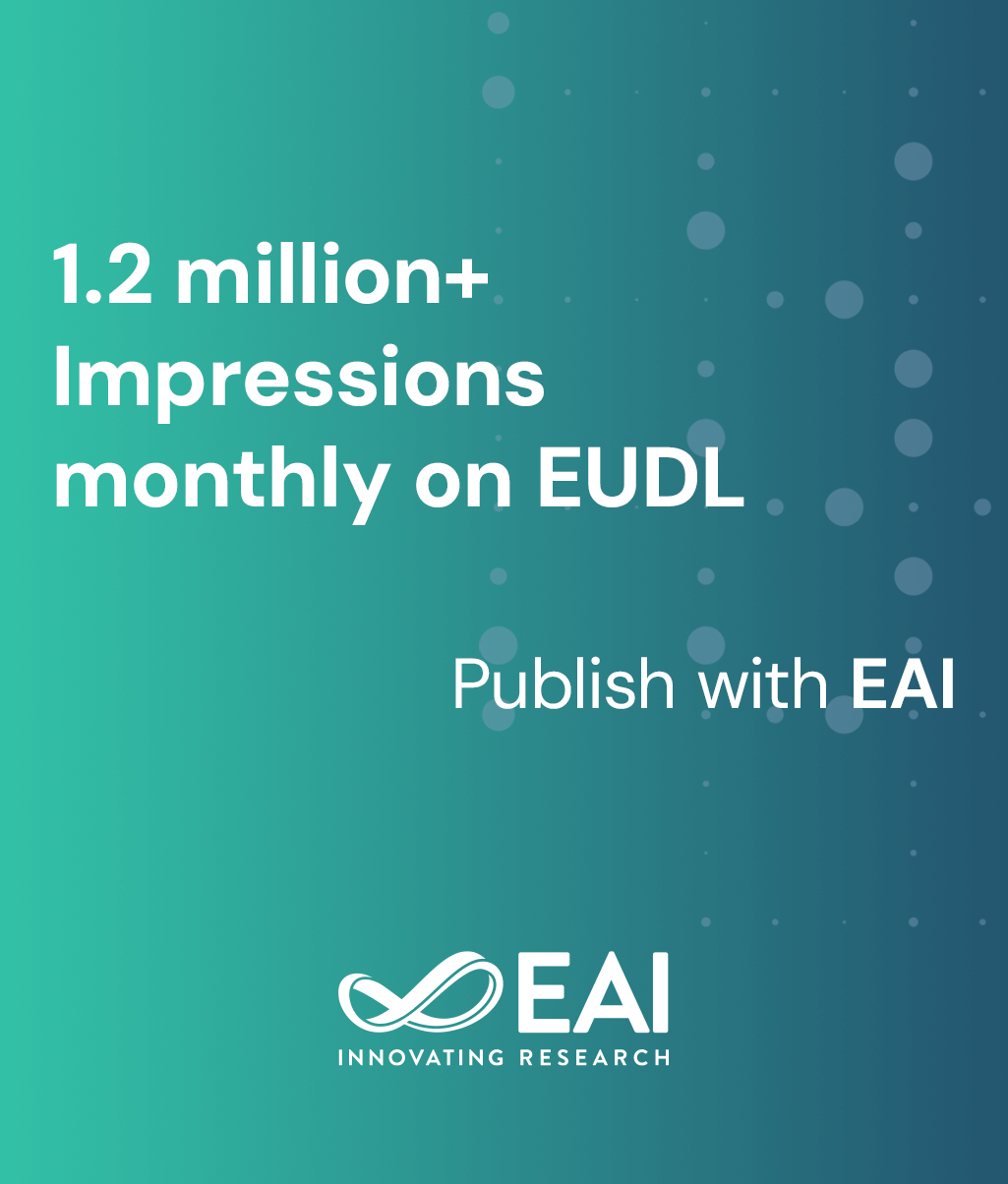
Research Article
A Proposal of Clinical Decision Support System Using Ensemble Learning for Coronary Artery Disease Diagnosis
@INPROCEEDINGS{10.1007/978-3-030-70569-5_19, author={Rawia Sammout and Kais Ben Salah and Khaled Ghedira and Rania Abdelhedi and Najla Kharrat}, title={A Proposal of Clinical Decision Support System Using Ensemble Learning for Coronary Artery Disease Diagnosis}, proceedings={Wireless Mobile Communication and Healthcare. 9th EAI International Conference, MobiHealth 2020, Virtual Event, November 19, 2020, Proceedings}, proceedings_a={MOBIHEALTH}, year={2021}, month={7}, keywords={Coronary artery heart disease Genetic factors Medications Ensemble learning}, doi={10.1007/978-3-030-70569-5_19} }
- Rawia Sammout
Kais Ben Salah
Khaled Ghedira
Rania Abdelhedi
Najla Kharrat
Year: 2021
A Proposal of Clinical Decision Support System Using Ensemble Learning for Coronary Artery Disease Diagnosis
MOBIHEALTH
Springer
DOI: 10.1007/978-3-030-70569-5_19
Abstract
Coronary Artery heart Disease (CAD) is the leading cause of mortality in the world. It is a complex and multifactorial disease resulting in several acute coronary syndromes and lead to death. In healthcare, an accurate clinical decision support system (CDSS) for CAD prediction has become increasingly important for making granted decisions at premature stage. Intensive research has been conducted on improving classification performance using machine learning techniques and metaheuristics algorithms. But most of these studies introduced the “classic risk factors” for CAD diagnosis i.e., demographic and clinical data. In this study, we present a novel CDSS based on ensemble learning for CAD prediction and we emphasize on adding other medical markers i.e., therapy data, some genetic polymorphisms along with classical factors. The new framework exploits the potential of three base classifiers including Support Vector Machines, Naïve Bayes and Decision Tree C4.5 to improve the prediction performance. Six experimental data used to build the proposed framework: the first one is collected from a Tunisian biotechnology center and the five other datasets from the University of California at Irvine repository. The analysis of the results shows that the proposed CDSS has the highest rate on classification accuracy, precision, recall and F1-measure when compared with CSGA Bagging and Adaptive boosting on the different datasets and proves that some medications and genetic polymorphisms such as Antivitamin K, Dose Beta Blocker, Proton pump inhibitor, CYP2C19*17, Clopidogrel active metabolite have an impact in CAD diagnosis.