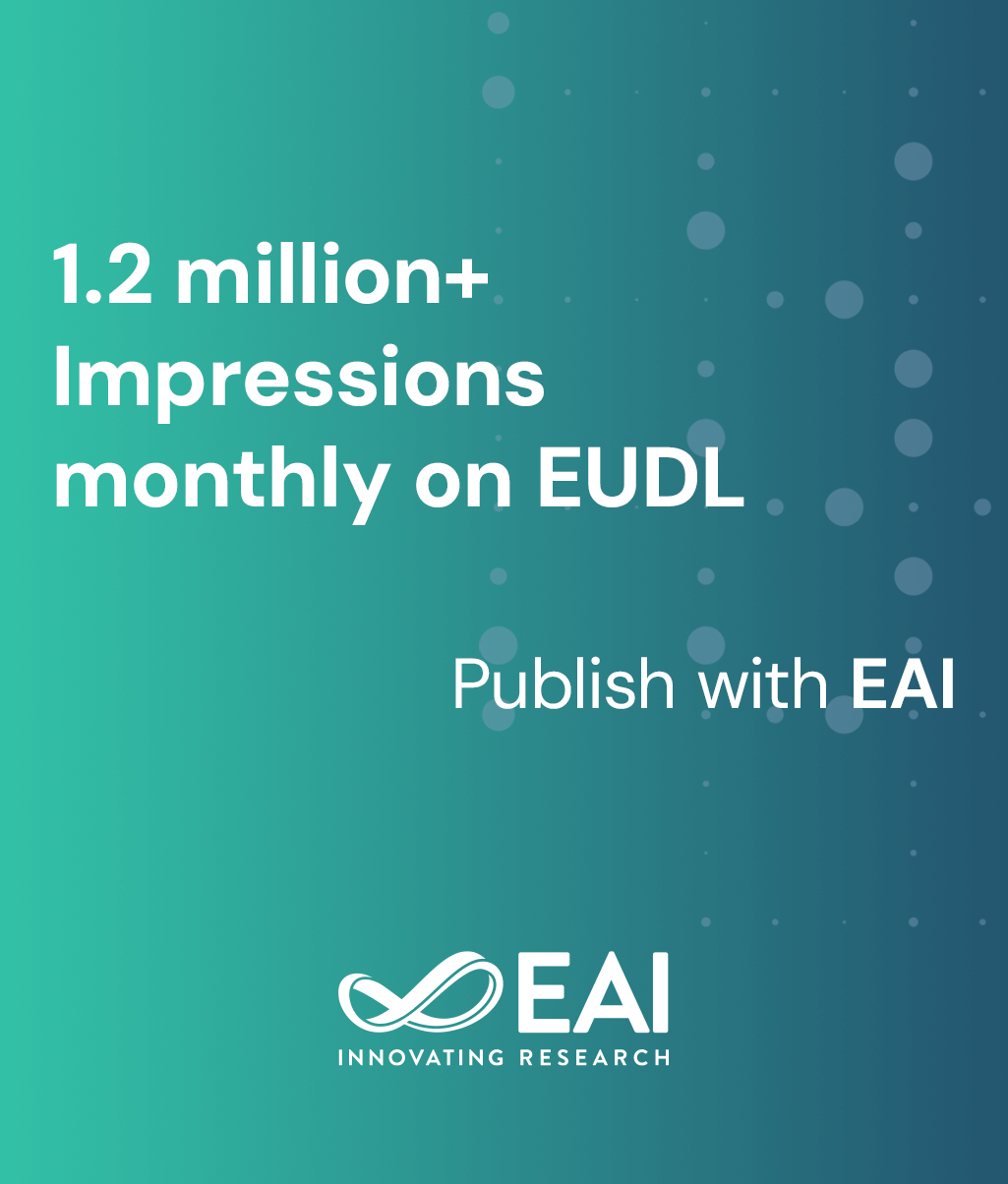
Research Article
Using Bayesian Optimization to Effectively Tune Random Forest and XGBoost Hyperparameters for Early Alzheimer’s Disease Diagnosis
@INPROCEEDINGS{10.1007/978-3-030-70569-5_18, author={Louise Bloch and Christoph M. Friedrich}, title={Using Bayesian Optimization to Effectively Tune Random Forest and XGBoost Hyperparameters for Early Alzheimer’s Disease Diagnosis}, proceedings={Wireless Mobile Communication and Healthcare. 9th EAI International Conference, MobiHealth 2020, Virtual Event, November 19, 2020, Proceedings}, proceedings_a={MOBIHEALTH}, year={2021}, month={7}, keywords={Bayesian optimization Computer-aided diagnosis Early Alzheimer’s Disease diagnosis eXtreme Gradient Boosting Random Forests}, doi={10.1007/978-3-030-70569-5_18} }
- Louise Bloch
Christoph M. Friedrich
Year: 2021
Using Bayesian Optimization to Effectively Tune Random Forest and XGBoost Hyperparameters for Early Alzheimer’s Disease Diagnosis
MOBIHEALTH
Springer
DOI: 10.1007/978-3-030-70569-5_18
Abstract
Many research articles used Machine Learning (ML) for early detection of Alzheimer’s Disease (AD) especially based on Magnetic Resonance Imaging (MRI). Most ML algorithms depend on a large number of hyperparameters. Those hyperparameters have a strong influence on the model performance and thus choosing good hyperparameters is important in ML. In this article, Bayesian Optimization (BO) was used to time-efficiently find good hyperparameters for Random Forest (RF) and eXtreme Gradient Boosting (XGBoost) models, which are based on four and seven hyperparameters and promise good classification results. Those models are applied to distinguish if mild cognitive impaired (MCI) subjects from the Alzheimer’s disease neuroimaging initiative (ADNI) dataset will prospectively convert to AD. The results showed comparable cross-validation (CV) classification accuracies for models trained using BO and grid-search, whereas BO has been less time-consuming. The initial combinations for BO were set using Latin Hypercube Design (LHD) and via Random Initialization (RI). Furthermore, many models trained using BO achieved better classification results for the independent test dataset than the model based on the grid-search. The best model achieved an accuracy of 73.43% for the independent test dataset. This model was an XGBoost model trained with BO and RI.