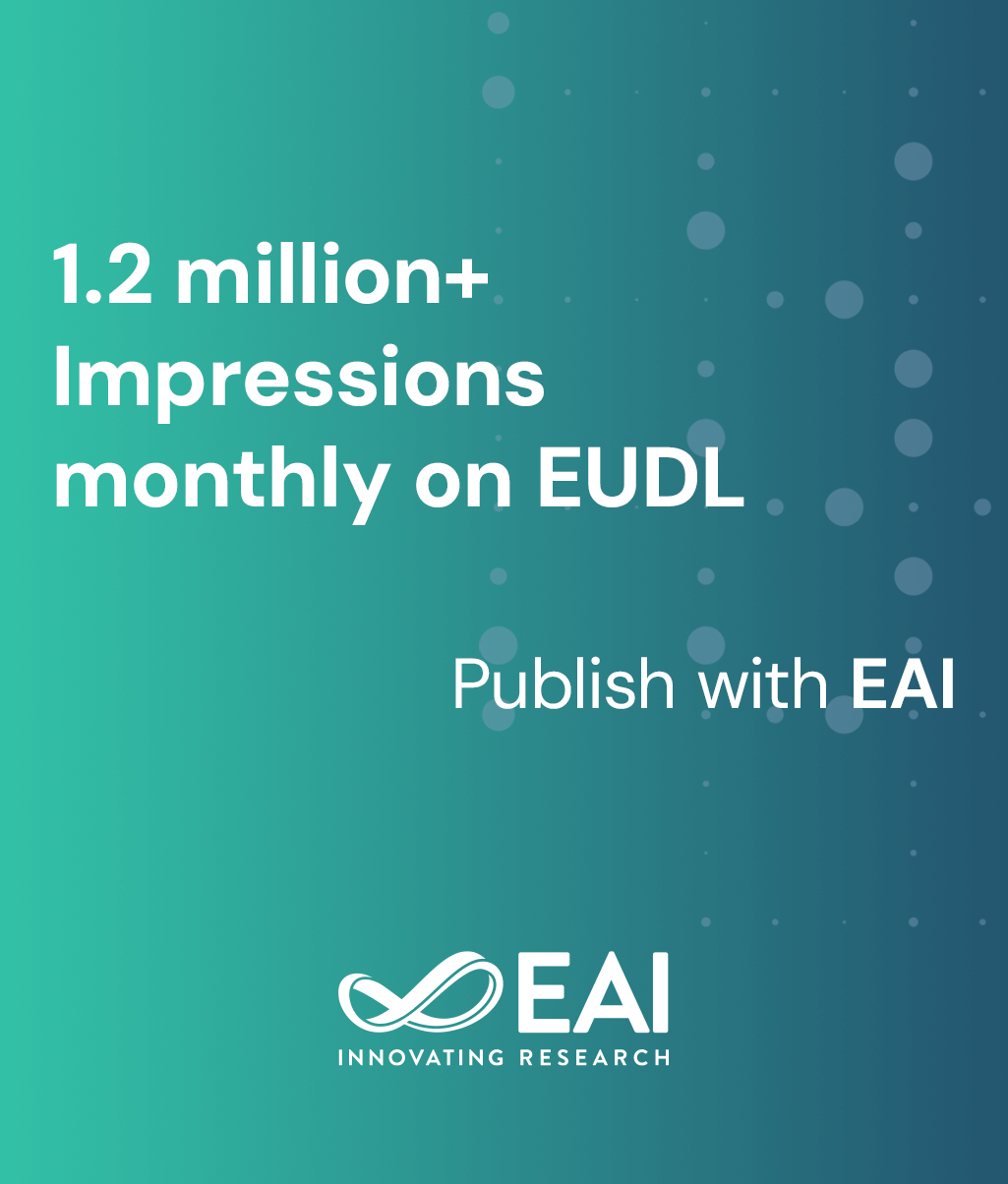
Research Article
Chronic Kidney Disease Early Diagnosis Enhancing by Using Data Mining Classification and Features Selection
@INPROCEEDINGS{10.1007/978-3-030-69963-5_5, author={Pedro A. Moreno-Sanchez}, title={Chronic Kidney Disease Early Diagnosis Enhancing by Using Data Mining Classification and Features Selection}, proceedings={IoT Technologies for HealthCare. 7th EAI International Conference, HealthyIoT 2020, Viana do Castelo, Portugal, December 3, 2020, Proceedings}, proceedings_a={HEALTHYIOT}, year={2021}, month={7}, keywords={Chronic kidney disease Early diagnosis Data mining Classification Feature selection}, doi={10.1007/978-3-030-69963-5_5} }
- Pedro A. Moreno-Sanchez
Year: 2021
Chronic Kidney Disease Early Diagnosis Enhancing by Using Data Mining Classification and Features Selection
HEALTHYIOT
Springer
DOI: 10.1007/978-3-030-69963-5_5
Abstract
Chronic Kidney Disease (CKD) is currently a worldwide chronic disease with an increasing incidence, prevalence and high cost to health systems. A delayed recognition and prevention often lead to a premature mortality due to progressive and incurable loss of kidney function. Data mining classifiers employment to discover patterns in CKD indicators would contribute to an early diagnosis that allow patients to prevent such kidney severe damage. Adopting the cross Industry Standard Process of Data Mining (CRISP-DM) methodology, this work develops a classifier model that would support healthcare professionals in early diagnosis of CKD patients. By building a data pipeline that manages the different phases of CRISP-DM, an automated data transformation, modelling and evaluation is applied to the CKD dataset extracted from the UCI ML repository. Moreover, the pipeline along with the Scikit-learn package’s GridSearchCV is used to carry out an exhaustive search of the best data mining classifier and the different parameters of the data preparation’s sub-stages like data missing and feature selection. Thus, AdaBoost is selected as the best classifier and it outperforms with a 100% in terms of accuracy, precision, sensivity, specificity, f1-score and roc auc, the classification results obtained by the related works reviewed. Moreover, the application of feature selection reduces up to 12 out of 24 features which are employed in the classifier model developed.