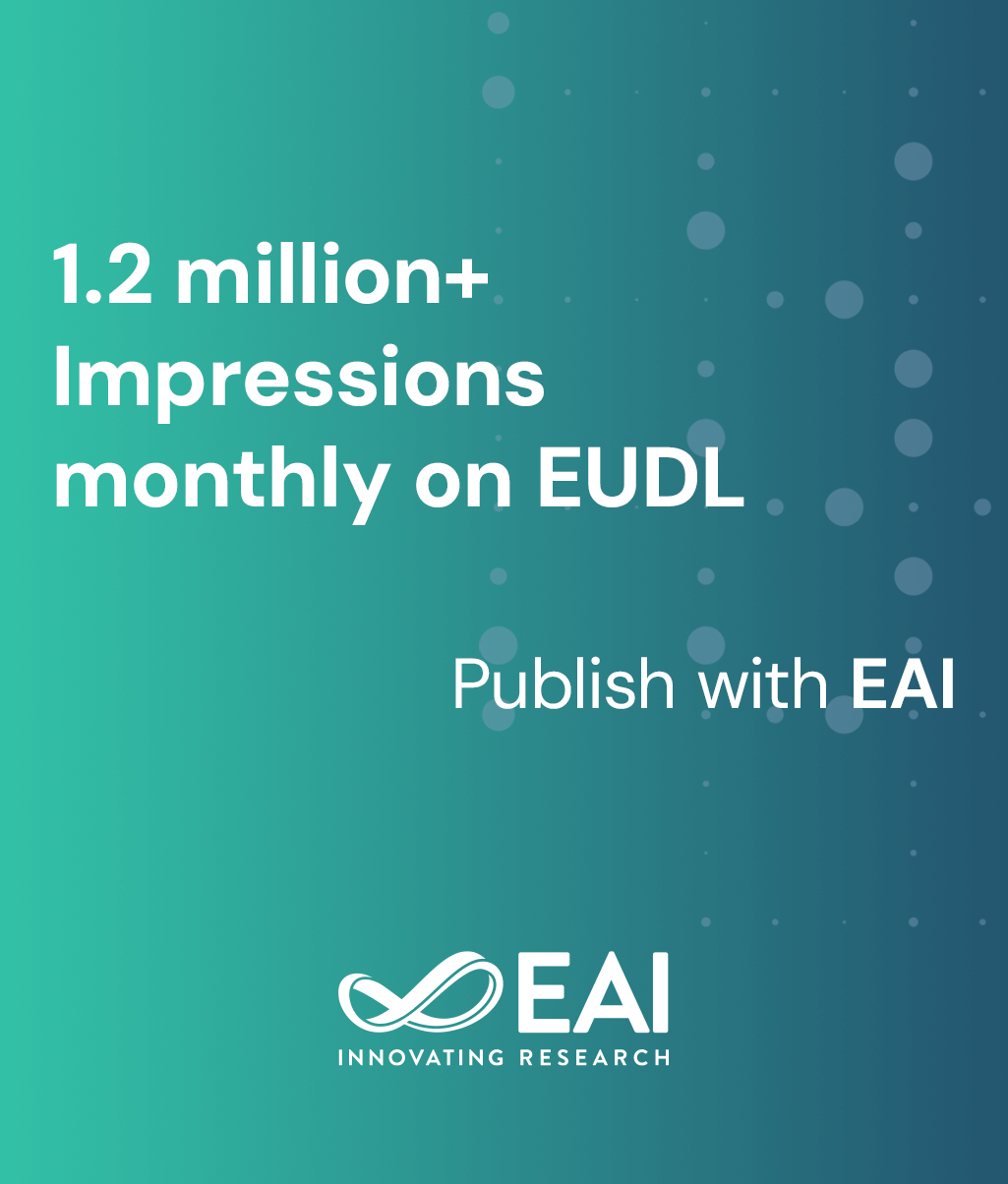
Research Article
Fair Resource Reusing for D2D Communication Based on Reinforcement Learning
@INPROCEEDINGS{10.1007/978-3-030-69514-9_5, author={Fang-Chang Kuo and Hwang-Cheng Wang and Jia-Hao Xu and Chih-Cheng Tseng}, title={Fair Resource Reusing for D2D Communication Based on Reinforcement Learning}, proceedings={Smart Grid and Internet of Things. 4th EAI International Conference, SGIoT 2020, TaiChung, Taiwan, December 5--6, 2020, Proceedings}, proceedings_a={SGIOT}, year={2021}, month={7}, keywords={Device-to-Device (D2D) Resource allocation Reinforcement learning Multi-Player Multi-Armed Bandit (MPMAB) Dynamic resource allocation}, doi={10.1007/978-3-030-69514-9_5} }
- Fang-Chang Kuo
Hwang-Cheng Wang
Jia-Hao Xu
Chih-Cheng Tseng
Year: 2021
Fair Resource Reusing for D2D Communication Based on Reinforcement Learning
SGIOT
Springer
DOI: 10.1007/978-3-030-69514-9_5
Abstract
Device-to-device (D2D) communications can improve the overall network performance, including low latency, high data rates, and system capability for the fifth generation (5G) wireless networks. The system capability can even be improved by reusing resource between D2D user equipment (DUE) and cellular user equipment (CUE) without bring harmful interference to the CUEs. A D2D resource allocation method is expected to have the characteristic that one CUE can be allocated with variable number of resource blocks (RBs), and the RBs can be reused by more than one CUE. In this study, Multi-Player Multi-Armed Bandit (MPMAB) reinforcement learning method is employed to model such problem by establishing preference matrix. A fair resource allocation method is then proposed to achieve fairness, prevent wasting resource, and alleviate starvation. This method even has better throughput if there are not too many D2D pairs.