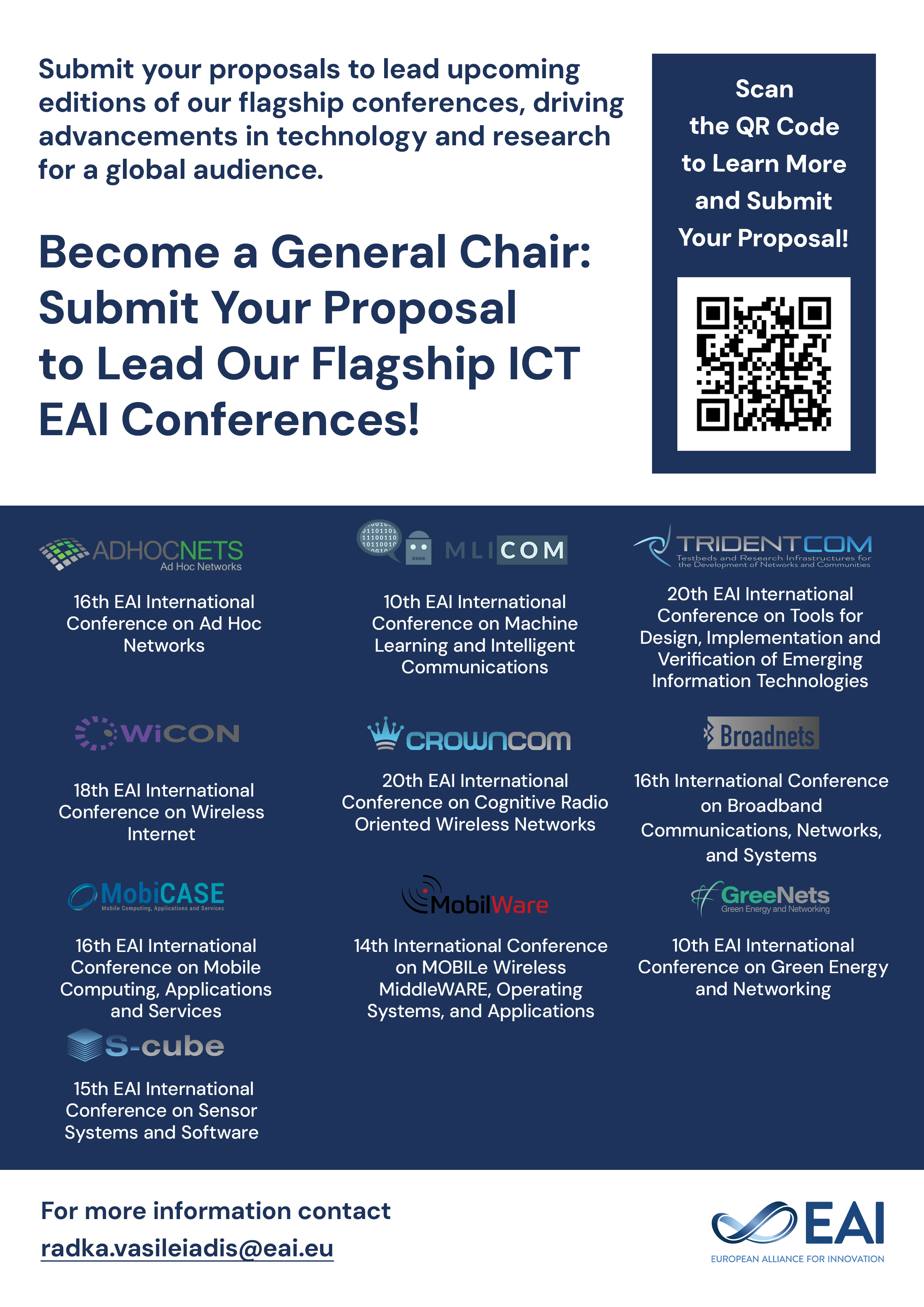
Research Article
Deep Learning at the Edge for Operation and Maintenance of Large-Scale Solar Farms
@INPROCEEDINGS{10.1007/978-3-030-69514-9_4, author={Salsabeel Shapsough and Imran Zualkernan and Rached Dhaouadi}, title={Deep Learning at the Edge for Operation and Maintenance of Large-Scale Solar Farms}, proceedings={Smart Grid and Internet of Things. 4th EAI International Conference, SGIoT 2020, TaiChung, Taiwan, December 5--6, 2020, Proceedings}, proceedings_a={SGIOT}, year={2021}, month={7}, keywords={Solar power Neural networks Edge analytics Fog computing}, doi={10.1007/978-3-030-69514-9_4} }
- Salsabeel Shapsough
Imran Zualkernan
Rached Dhaouadi
Year: 2021
Deep Learning at the Edge for Operation and Maintenance of Large-Scale Solar Farms
SGIOT
Springer
DOI: 10.1007/978-3-030-69514-9_4
Abstract
Real-time monitoring of large-scale solar farms is one important aspect of reliable and secure deployment of 100% renewable energy-based grids. The ability to observe sensors on solar panels using Internet of Things (IoT) technologies makes it possible to study the behavior of solar panels under various conditions and to detect anomalous behaviors in real-time. Such technologies make it possible for grid administrators to make informed decisions in reacting to anomalies such as panel damage, electrical errors, monitoring hardware decay, or malicious data injection attacks. Smart edge devices offer an opportunity to reduce the cost of continuously sending data for anomaly detection by performing analytics on local edge device within a given farm and sending only the result of the analysis back to datacenters. This paper presents the design and evaluation of a low-cost edge-based anomaly detection system for remote solar farms using Raspberry Pi and deep learning. The design was implemented and tested using real-life observations from a solar monitoring system under soiling conditions. The experiments showed that it is possible to run real-time anomaly detection algorithms on edge devices with little overhead in terms of power consumption and utilization of computational resources, making it an ideal system for large-scale implementation.