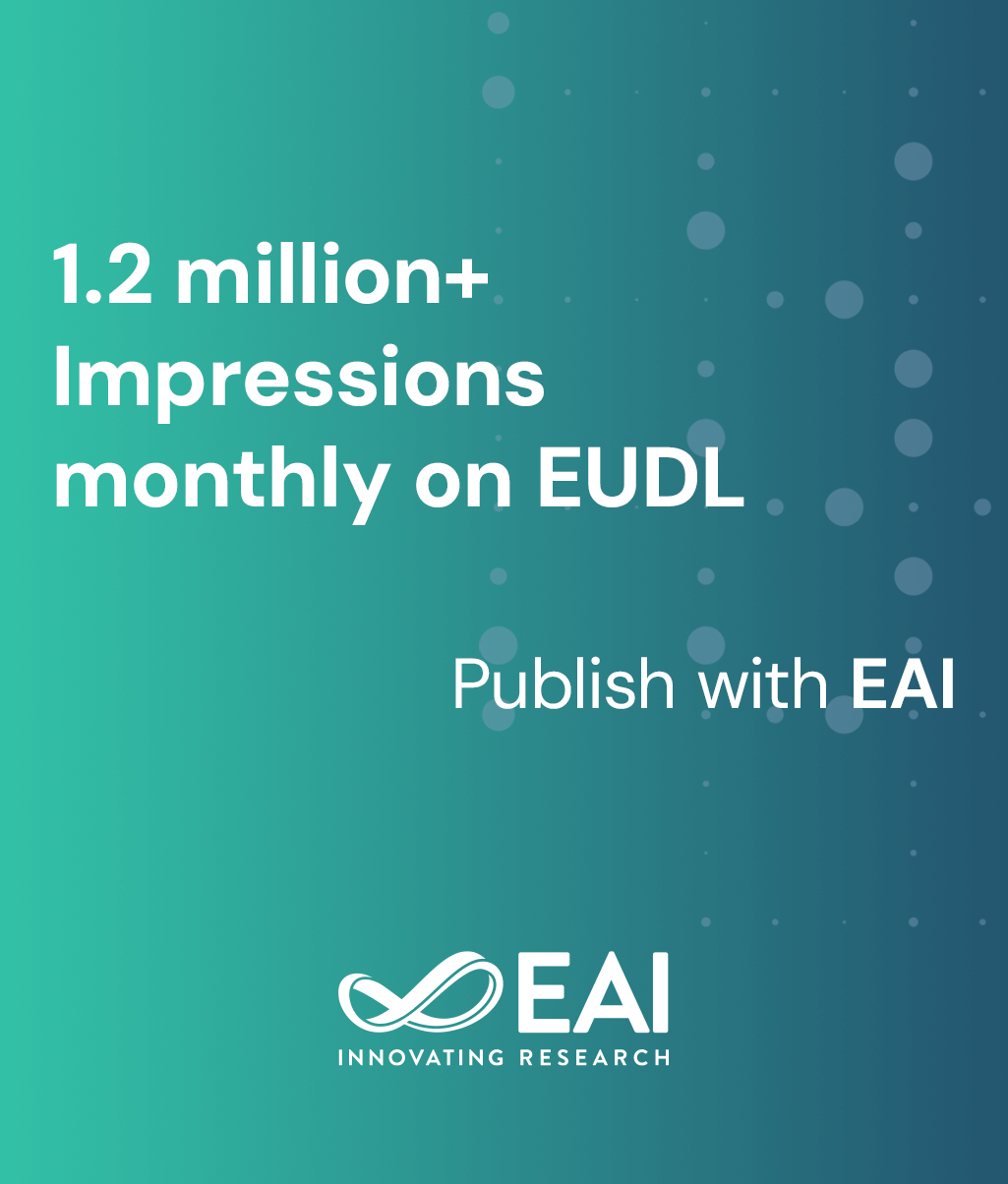
Research Article
Classification of Uncertain Data Based on Evidence Theory in Wireless Sensor Networks
@INPROCEEDINGS{10.1007/978-3-030-69514-9_13, author={Yang Zhang and Yun Liu and Zhenjiang Zhang}, title={Classification of Uncertain Data Based on Evidence Theory in Wireless Sensor Networks}, proceedings={Smart Grid and Internet of Things. 4th EAI International Conference, SGIoT 2020, TaiChung, Taiwan, December 5--6, 2020, Proceedings}, proceedings_a={SGIOT}, year={2021}, month={7}, keywords={Evidence theory Uncertain data Target classification Combination rule}, doi={10.1007/978-3-030-69514-9_13} }
- Yang Zhang
Yun Liu
Zhenjiang Zhang
Year: 2021
Classification of Uncertain Data Based on Evidence Theory in Wireless Sensor Networks
SGIOT
Springer
DOI: 10.1007/978-3-030-69514-9_13
Abstract
In wireless sensor networks, the classification of uncertain data reported by sensor nodes is an open issue because the given attribute information can be insufficient for making a correct specific classification of the objects. Although the traditional Evidential -Nearest Neighbor (ENN) algorithm can effectively model the uncertainty, it is easy to misjudge the target data to the incorrect class when the observed sample data is located in the feature overlapping region of training samples of different classes. In this paper, a novel Evidential -Nearest Neighbor (NENN) algorithm is proposed based on the evidential editing method. The main idea of NENN algorithm is to consider the expected value and standard deviation of various training sample data sets, and use normalized Euclidean distance to assign class labels with basic belief assignment (BBA) structure to each training sample, so that training samples in overlapping region can offer more abundant and diverse class information. Further, ENN classification of the observation sample data is carried out in the training sample sets of various classes, and mass functions of the target to be tested under this class are obtained, and Redistribute Conflicting Mass Proportionally Rule 5 (PCR5) combination rule is used to conduct global fusion, thus obtaining the global fusion results of the targets. The experimental results show that this algorithm has better performance than other classification methods based on -nearest neighbor.