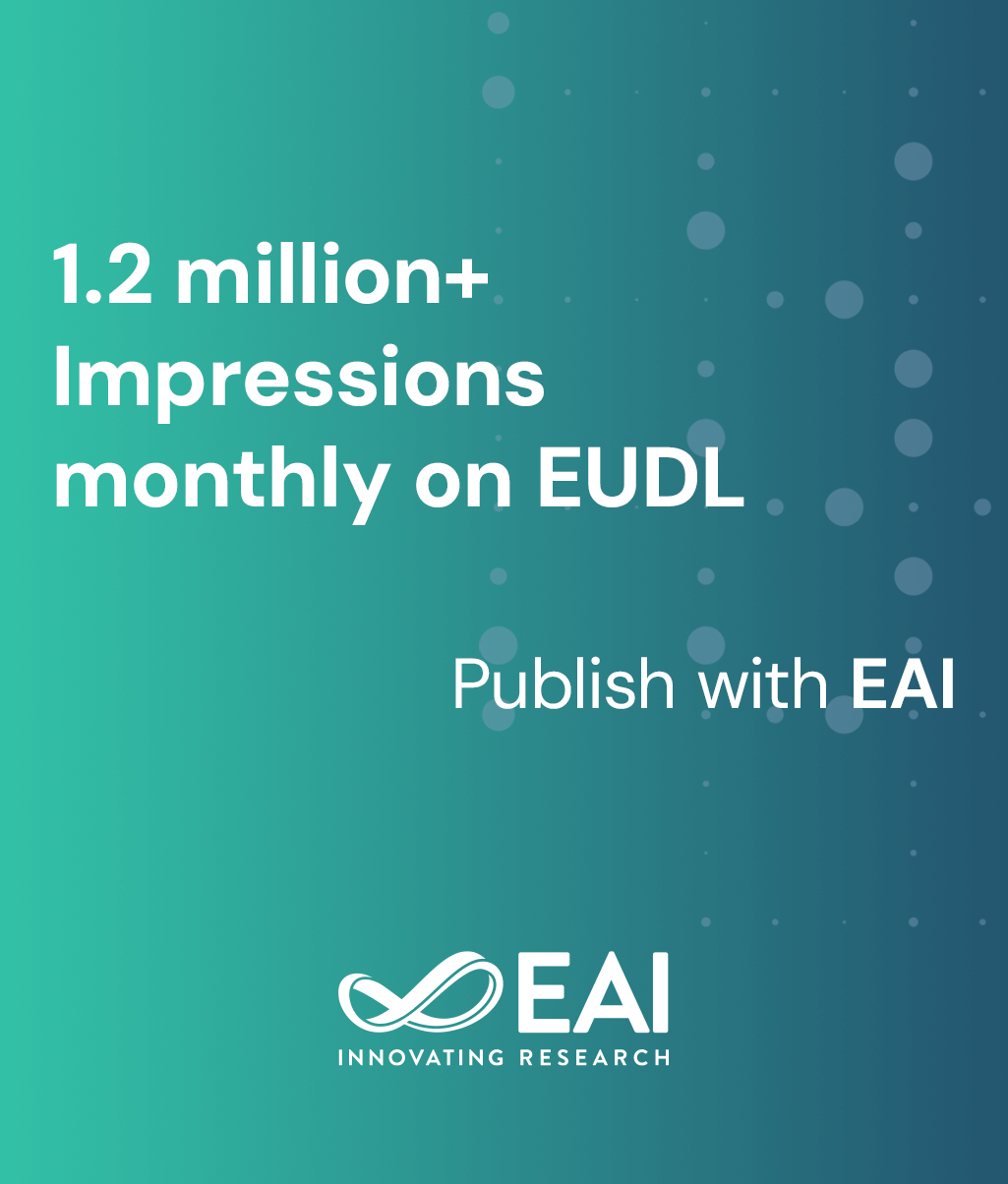
Research Article
Machine Learning-Based Security Authentication for IoT Networks
@INPROCEEDINGS{10.1007/978-3-030-69514-9_10, author={Xiaoying Qiu and Xuan Sun and Xiameng Si}, title={Machine Learning-Based Security Authentication for IoT Networks}, proceedings={Smart Grid and Internet of Things. 4th EAI International Conference, SGIoT 2020, TaiChung, Taiwan, December 5--6, 2020, Proceedings}, proceedings_a={SGIOT}, year={2021}, month={7}, keywords={Wireless communication Security authentication Physical layer security Machine learning}, doi={10.1007/978-3-030-69514-9_10} }
- Xiaoying Qiu
Xuan Sun
Xiameng Si
Year: 2021
Machine Learning-Based Security Authentication for IoT Networks
SGIOT
Springer
DOI: 10.1007/978-3-030-69514-9_10
Abstract
In this paper, we propose a security authentication scheme based on machine learning algorithms to detect spoofing attacks in the Internet of Things (IoT) network. This authentication method exploits the physical layer properties of the wireless channel to identify sensors and applies neural networks to learn channel fingerprints without being aware of the communication network model. We propose a channel differences-based security framework to provide lightweight authentication and a Long Short-Term Memory (LSTM) network-based detection approach to further enhance the authentication performance for sinks that support intelligent algorithms. Experiments and simulations were carried out in an indoor conference room. The results show that our strategy improves the authentication accuracy rate compared with the existing non-learning security authentication methods.