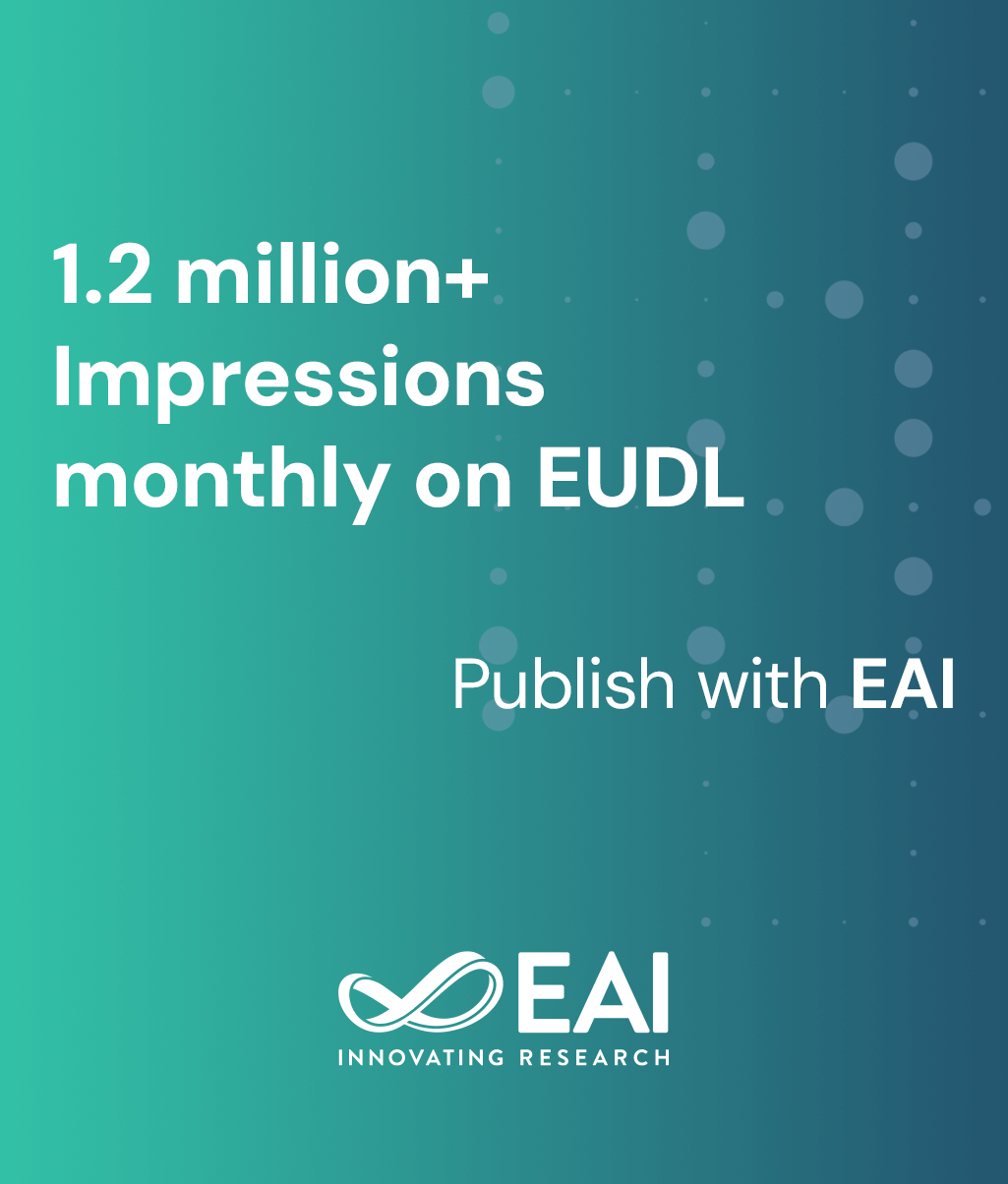
Research Article
Research of Offloading Decision and Resource Scheduling in Edge Computing Based on Deep Reinforcement Learning
@INPROCEEDINGS{10.1007/978-3-030-69514-9_1, author={Zhen-Jiang Zhang and Tong Wu and Zhiyuan Li and Bo Shen and Naiyue Chen and Jian Li}, title={Research of Offloading Decision and Resource Scheduling in Edge Computing Based on Deep Reinforcement Learning}, proceedings={Smart Grid and Internet of Things. 4th EAI International Conference, SGIoT 2020, TaiChung, Taiwan, December 5--6, 2020, Proceedings}, proceedings_a={SGIOT}, year={2021}, month={7}, keywords={Edge computing Offloading decision Resource scheduling Deep reinforcement learning}, doi={10.1007/978-3-030-69514-9_1} }
- Zhen-Jiang Zhang
Tong Wu
Zhiyuan Li
Bo Shen
Naiyue Chen
Jian Li
Year: 2021
Research of Offloading Decision and Resource Scheduling in Edge Computing Based on Deep Reinforcement Learning
SGIOT
Springer
DOI: 10.1007/978-3-030-69514-9_1
Abstract
The increasing scale of the IOT poses challenges to the energy consumption, transmission bandwidth and processing delay of centralized cloud computing data centers. The cloud computing data centers is moving from the center of the network to edge nodes with lower latency, namely, edge computing. Meanwhile, it can meet the needs of users for real-time services. In the field of edge computing, offloading decision and resource scheduling are the hot-spot issues. As for offloading decision and resource scheduling problems of single-cell multi-user partial offloading, the system model is also firstly established from four aspects: network architecture, application type, local computing and offloading computing. Based on the system model, the optimization problem of resource scheduling is modeled, where the solution is hard to be found. Thus, the deep reinforcement learning method based on policy gradient is selected to establish the SPBDDPG algorithm that can solve the problem. Then, in order to solve the practical problems, the SPBDDPG algorithm is set up with the state and action for iteration, as well as the environment for generating new state and feedback reward value. Finally, an appropriate iteration step is written for the edge computing resource scheduling problem by combining with the original deep reinforcement learning algorithm. We also evaluate the proposed approaches by relevant experiments. The complexity and effectiveness of the results are validated.