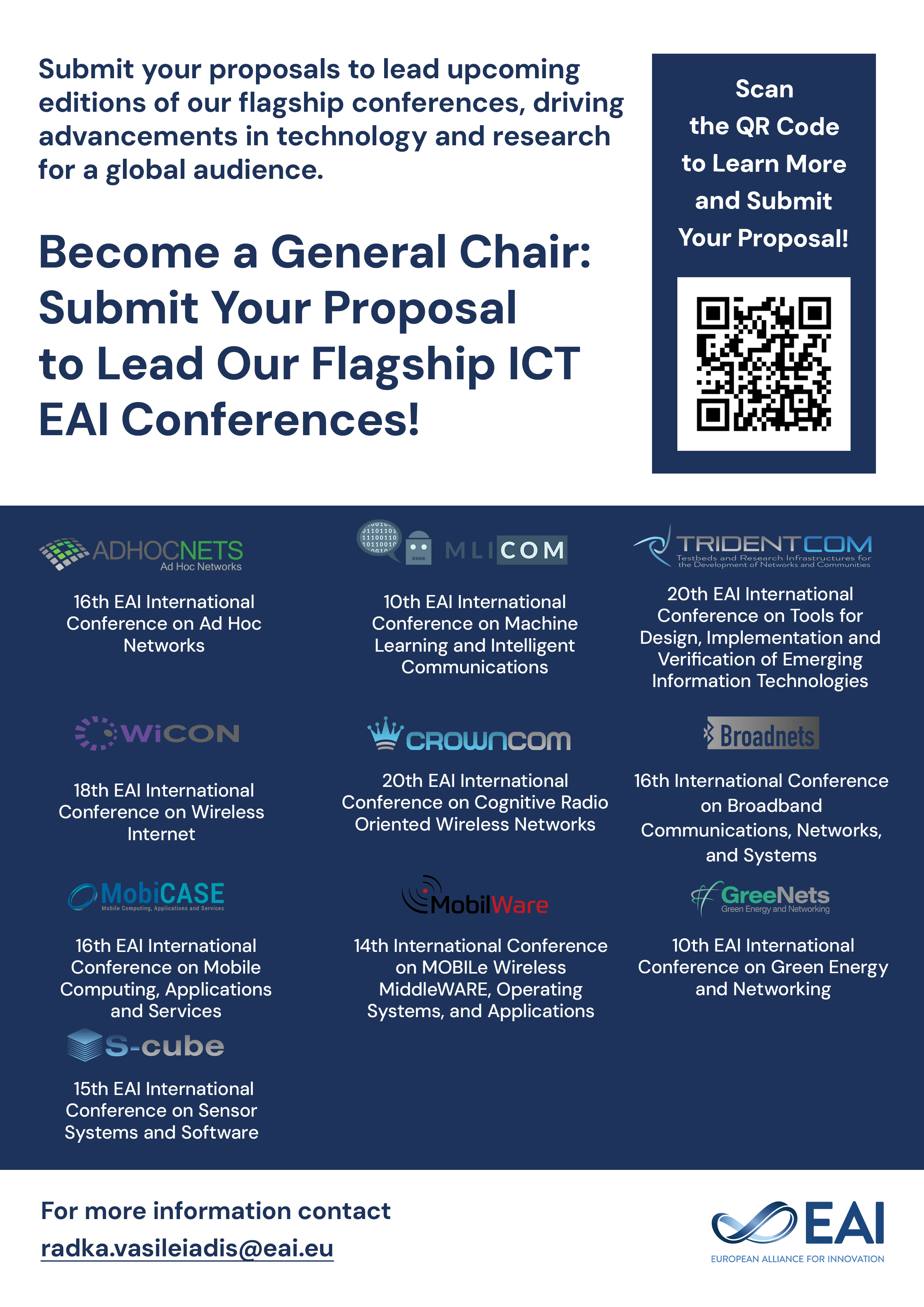
Research Article
A Real-Time Two-Stage Detector for Static Monitor Using GMM for Region Proposal
@INPROCEEDINGS{10.1007/978-3-030-69066-3_36, author={Yingping Liang and Yunfei Ma and Zhengliang Wu and Mingfeng Lu}, title={A Real-Time Two-Stage Detector for Static Monitor Using GMM for Region Proposal}, proceedings={Artificial Intelligence for Communications and Networks. Second EAI International Conference, AICON 2020, Virtual Event, December 19-20, 2020, Proceedings}, proceedings_a={AICON}, year={2021}, month={7}, keywords={Deep learning Computer vision Intelligent monitoring}, doi={10.1007/978-3-030-69066-3_36} }
- Yingping Liang
Yunfei Ma
Zhengliang Wu
Mingfeng Lu
Year: 2021
A Real-Time Two-Stage Detector for Static Monitor Using GMM for Region Proposal
AICON
Springer
DOI: 10.1007/978-3-030-69066-3_36
Abstract
CNN-based object detectors have been widely exploited for vision tasks. However, for specific real-time tasks (e.g. object detection on static monitor), the enormous computation cost makes it difficult to work. To reduce the computation cost for object detection on static monitor while inheriting high accuracy of CNN-based networks, this paper proposals a method with a two-stage detector using Gaussian mixture model for region proposal. We test our method on MOT16 datasets. Compared with original models, the two-stage detectors equipped with Gaussian region proposal achieve a better performance with the mAP increased by 0.20. We also design and train a light-weight detector based on our method, which is much faster and more suitable for mobile and embedded device with little drop in accuracy.