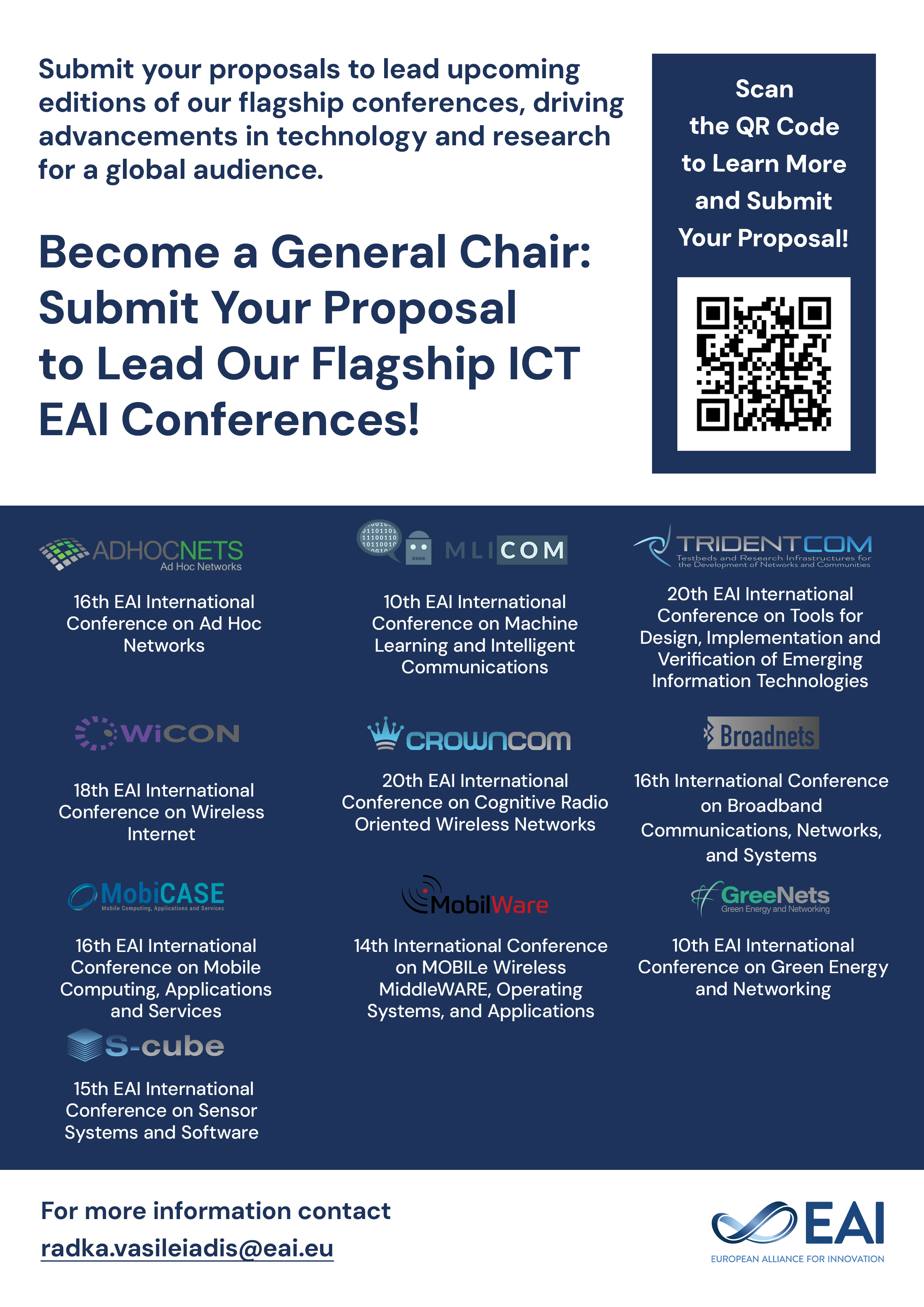
Research Article
Perceptual Quality Enhancement with Multi-scale Deep Learning for Video Transmission: A QoE Perspective
@INPROCEEDINGS{10.1007/978-3-030-69066-3_3, author={Chaoyi Han and Yiping Duan and Xiaoming Tao and Rundong Gao and Jianhua Lu}, title={Perceptual Quality Enhancement with Multi-scale Deep Learning for Video Transmission: A QoE Perspective}, proceedings={Artificial Intelligence for Communications and Networks. Second EAI International Conference, AICON 2020, Virtual Event, December 19-20, 2020, Proceedings}, proceedings_a={AICON}, year={2021}, month={7}, keywords={Quality of experience (QoE) Perceptual image enhancement Wireless communications}, doi={10.1007/978-3-030-69066-3_3} }
- Chaoyi Han
Yiping Duan
Xiaoming Tao
Rundong Gao
Jianhua Lu
Year: 2021
Perceptual Quality Enhancement with Multi-scale Deep Learning for Video Transmission: A QoE Perspective
AICON
Springer
DOI: 10.1007/978-3-030-69066-3_3
Abstract
With the development of mobile Internet technologies, wireless communication is facing huge challenges under the explosive growth of multimedia data, e.g. video conferences, online education. This makes it difficult to guarantee the communication quality where communication resources (bandwidth, channel, etc.) are limited. In this paper, we propose an image enhancement method to transform blurred images into images with high perceptual quality. The proposed method serves as a post-processing part for communication systems and is incorporated into the receiver. Specifically, we learn the prior of high quality images using a collected dataset. We train a neural network to accomplish this task and adopt a multi-scale perceptual loss as the objective, which is more consistent with the quality of experience (QoE). To validate the proposed method, we train our model on a large dataset with both blurred images and high quality images. Experimental results show that, using a pre-collected dataset with high quality images, the proposed approach can effectively restore the blurred images.