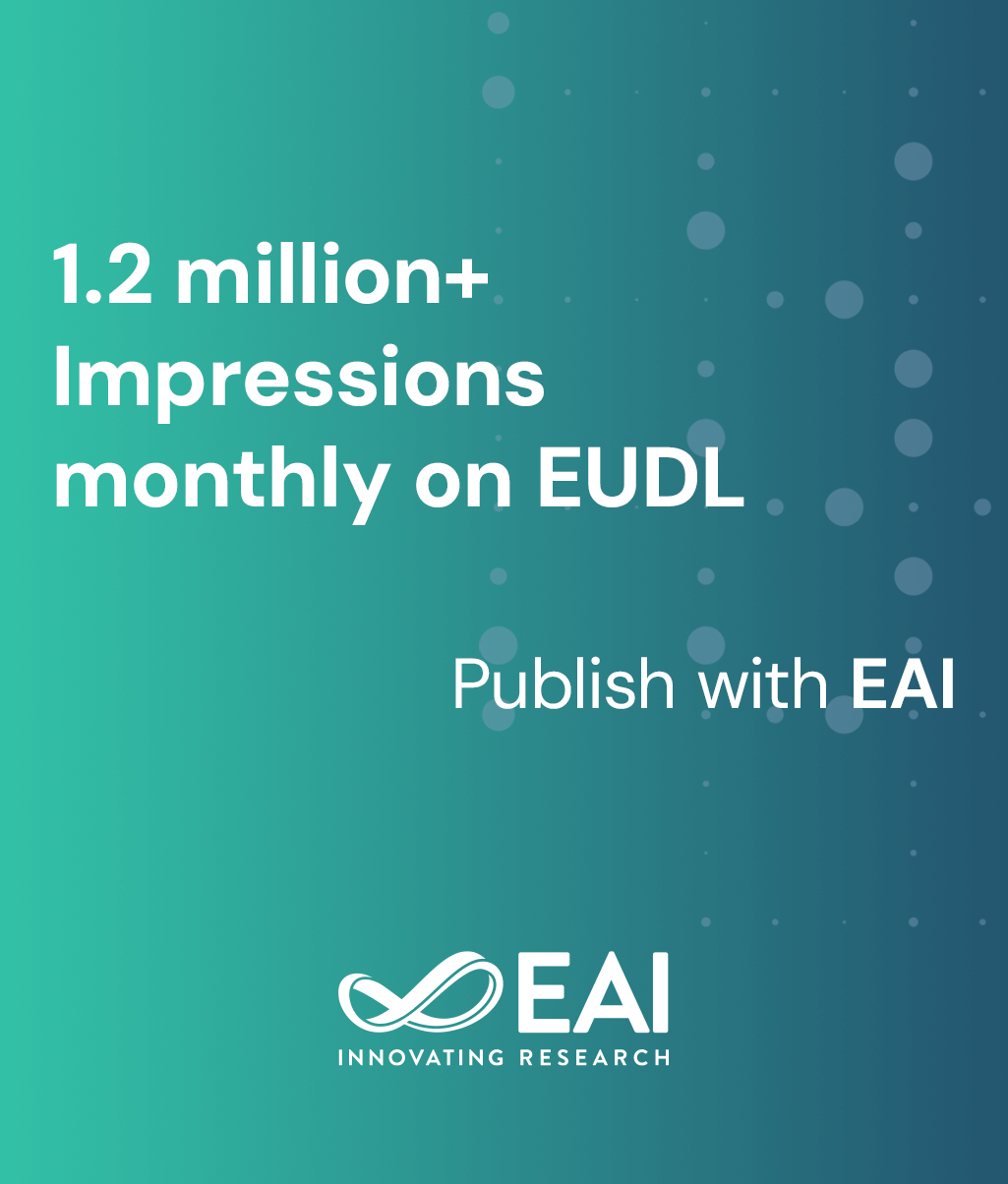
Research Article
Energy-Efficient Multi-UAV-Enabled Computation Offloading for Industrial Internet of Things via Deep Reinforcement Learning
@INPROCEEDINGS{10.1007/978-3-030-69066-3_26, author={Shuo Shi and Meng Wang and Xuemai Gu}, title={Energy-Efficient Multi-UAV-Enabled Computation Offloading for Industrial Internet of Things via Deep Reinforcement Learning}, proceedings={Artificial Intelligence for Communications and Networks. Second EAI International Conference, AICON 2020, Virtual Event, December 19-20, 2020, Proceedings}, proceedings_a={AICON}, year={2021}, month={7}, keywords={UAV-enabled IIoT Deep reinforcement learning Computation offloading}, doi={10.1007/978-3-030-69066-3_26} }
- Shuo Shi
Meng Wang
Xuemai Gu
Year: 2021
Energy-Efficient Multi-UAV-Enabled Computation Offloading for Industrial Internet of Things via Deep Reinforcement Learning
AICON
Springer
DOI: 10.1007/978-3-030-69066-3_26
Abstract
Industrial Internet of things (IIoT) has been envisioned as a key technology for Industry 4.0. However, the battery capcity and processing ability of IIoT devices are limited which imposes great challenges when handling tasks with high quality of service (QoS) requirements. Toward this end, in this paper we first use multiple unmanned aerial vehicles (UAVs) equipped with computation resources to offer computation offloading opportunities for IIoT devices due to their high flexibility. Then we formulate the multi-UAV-enabled computation offloading problem as a mixed integer non-linear programming (MINLP) problem and prove its NP-hardness. Furthermore, to obtain the energy-efficient solutions for IIoT devices, we propose an intelligent algorithm called multi-agent deep Q-learning with stochastic prioritized replay (MDSPR). Simulation results show that the proposed MDSPR converges fast and outperforms the normal deep Q-learning (DQN) method and other benchmark algorithms in terms of energy-efficiency and tasks’ successful rate.