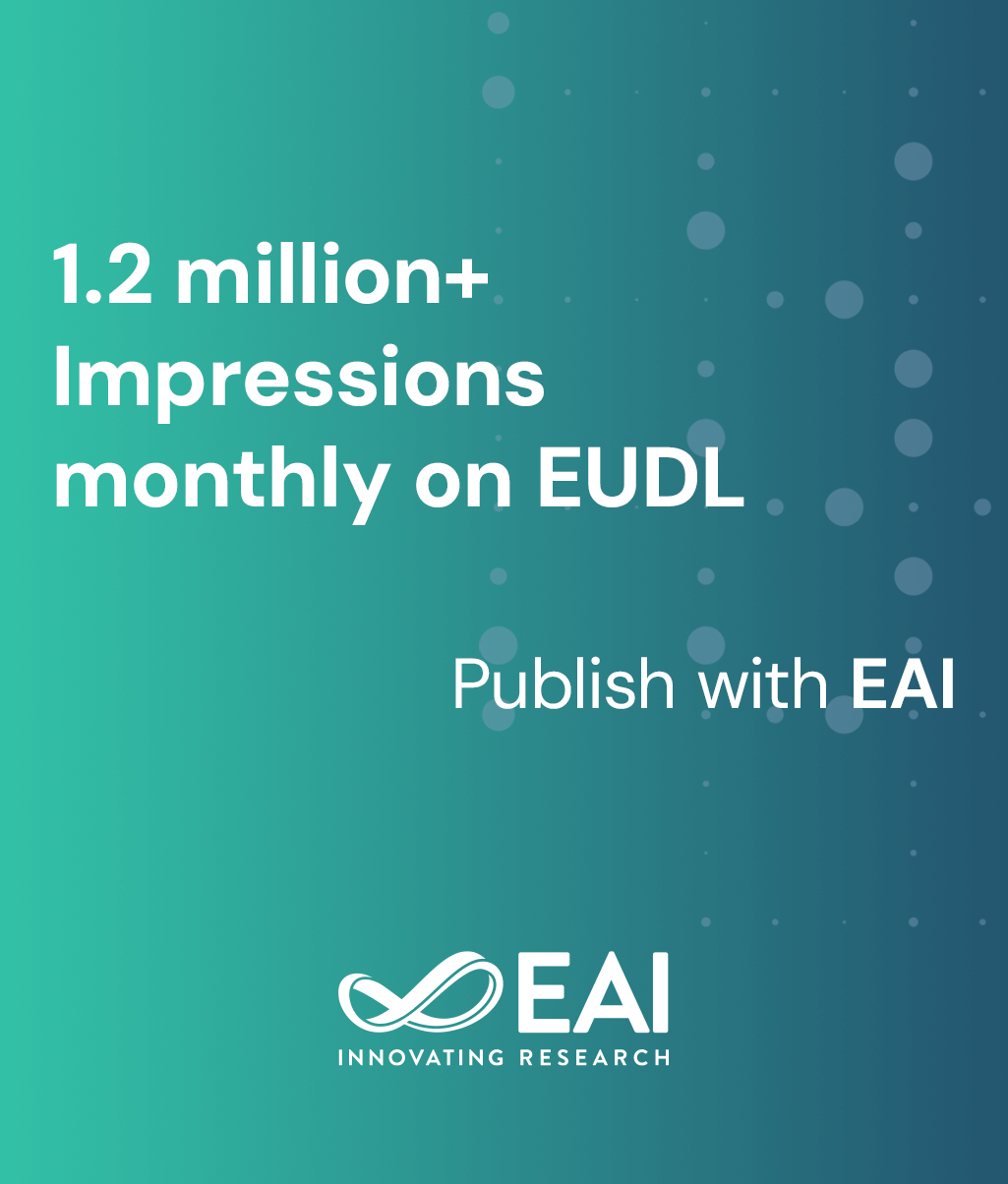
Research Article
Delay Minimization in Multi-UAV Assisted Wireless Networks: A Reinforcement Learning Approach
@INPROCEEDINGS{10.1007/978-3-030-69066-3_22, author={Chenyu Wu and Xuemai Gu and Shuo Shi}, title={Delay Minimization in Multi-UAV Assisted Wireless Networks: A Reinforcement Learning Approach}, proceedings={Artificial Intelligence for Communications and Networks. Second EAI International Conference, AICON 2020, Virtual Event, December 19-20, 2020, Proceedings}, proceedings_a={AICON}, year={2021}, month={7}, keywords={Unmanned Aerial Vehicles Delay minimization Deployment Reinforcement learning}, doi={10.1007/978-3-030-69066-3_22} }
- Chenyu Wu
Xuemai Gu
Shuo Shi
Year: 2021
Delay Minimization in Multi-UAV Assisted Wireless Networks: A Reinforcement Learning Approach
AICON
Springer
DOI: 10.1007/978-3-030-69066-3_22
Abstract
Unmanned Aerial Vehicles (UAVs) assisted communications are promising technology for meeting the demand of unprecedented demands for wireless services. In this paper, we propose a novel framework for delay minimization driven deployment of multiple UAVs. The problem of joint non-convex three dimensional (3D) deployment for minimizing average delay is formulated and solved by Deep Q network (DQN), which is a reinforcement learning based algorithm. Firstly, we obtain the cell partition by K-means algorithm. Then, we find the optimal 3D position for each UAV in each cluster to provide low delay service. Finally, when users are roaming, the UAVs are still able to track the real-time users. Numerical results show that the proposed DQN-based delay algorithm shows a fast convergence after a small number of iterations. Additionally, the proposed deployment algorithm outperforms several benchmarks in terms of average delay.