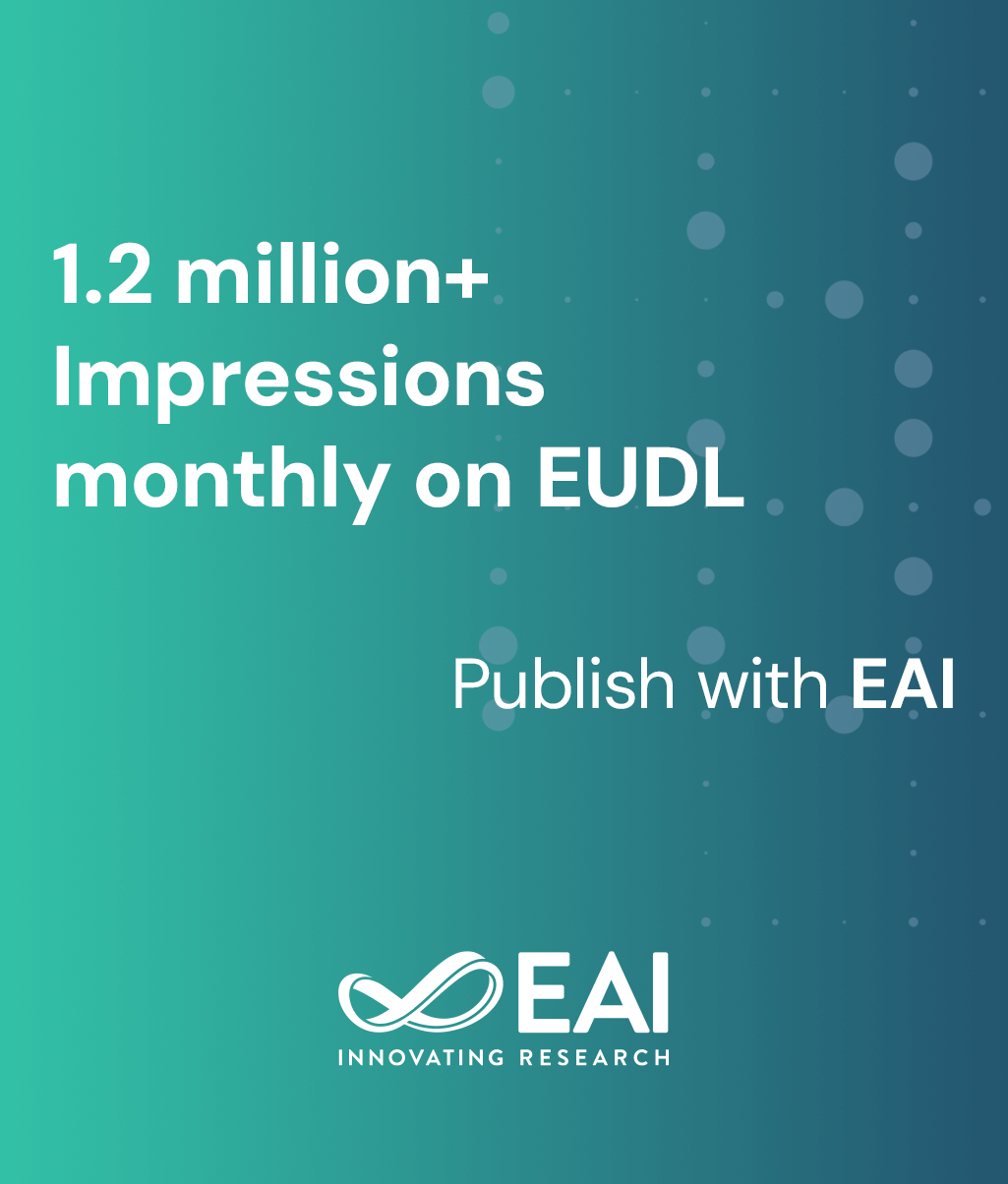
Research Article
Customized Attack Detection Under Power Industrial Control System
@INPROCEEDINGS{10.1007/978-3-030-49610-4_8, author={Bin Wang and Ling He and Huiting Yang and Feng Li and Jie Fan}, title={Customized Attack Detection Under Power Industrial Control System}, proceedings={Smart Grid and Internet of Things. Third EAI International Conference, SGIoT 2019, TaiChung, Taiwan, December 5-6, 2019, Proceedings}, proceedings_a={SGIOT}, year={2020}, month={6}, keywords={Power industrial control system Deep learning iForest}, doi={10.1007/978-3-030-49610-4_8} }
- Bin Wang
Ling He
Huiting Yang
Feng Li
Jie Fan
Year: 2020
Customized Attack Detection Under Power Industrial Control System
SGIOT
Springer
DOI: 10.1007/978-3-030-49610-4_8
Abstract
With the rapid development of information technology, the power system already has the typical characteristics of the information physical fusion system. The power industrial control system is widely used in the power industry. While improving the efficiency, the economic benefits have also been greatly improved. However, the dependence on information technology has also increased the vulnerability to malicious attacks. Power industry control system is facing a more serious threat. In this paper, we combine anomaly detection and data dimensionality reduction to propose a feature extraction method for iForest power measurement data, which not only ensures the targeting of attack detection in the data processing stage, but also takes into account the data quality of feature extraction. In addition, we use deep learning techniques to identify attack behavior characteristics and use captured features to detect attack behavior in real time. We prove the availability of the method through simulation of the IEEE 118-bus power systems.