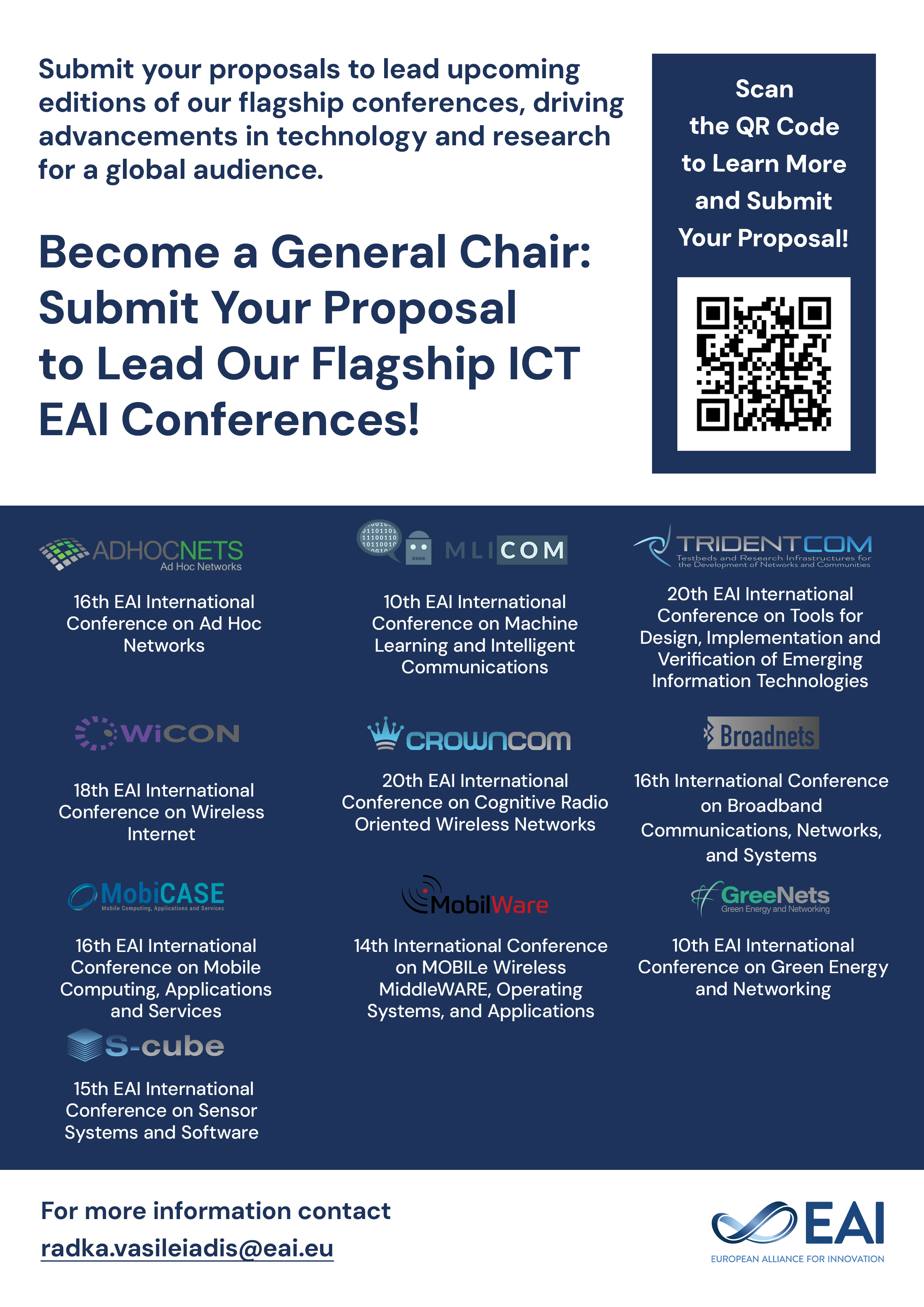
Research Article
A New Model of Cotton Yield Estimation Based on AWS
@INPROCEEDINGS{10.1007/978-3-030-48513-9_38, author={Quan Xu and Chuanjian Wang and Jianguo Dai and Peng Guo and Guoshun Zhang and Yan Jiang and Hongwei Shi}, title={A New Model of Cotton Yield Estimation Based on AWS}, proceedings={Cloud Computing, Smart Grid and Innovative Frontiers in Telecommunications. 9th EAI International Conference, CloudComp 2019, and 4th EAI International Conference, SmartGIFT 2019, Beijing, China, December 4-5, 2019, and December 21-22, 2019}, proceedings_a={CLOUDCOMP}, year={2020}, month={6}, keywords={Cotton Yield estimation Cloud computing AWS NDVI SENP}, doi={10.1007/978-3-030-48513-9_38} }
- Quan Xu
Chuanjian Wang
Jianguo Dai
Peng Guo
Guoshun Zhang
Yan Jiang
Hongwei Shi
Year: 2020
A New Model of Cotton Yield Estimation Based on AWS
CLOUDCOMP
Springer
DOI: 10.1007/978-3-030-48513-9_38
Abstract
Timely and precise yield estimation is of great significance to agricultural management and macro-policy formulation. In order to improve the accuracy and applicability of cotton yield estimation model, this paper proposes a new method called SENP (Seedling Emergence and Number of Peaches) based on Amazon Web Services (AWS). Firstly, using the high-resolution visible light data obtained by the Unmanned Aerial Vehicle (UAV), the spatial position of each cotton seedling in the region was extracted by U-Net model of deep learning. Secondly, Sentinel-2 data were used in analyzing the correlation between the multi-temporal Normalized Difference Vegetation Index (NDVI) and the actual yield, so as to determine the weighting factor of NDVI in each period in the model. Subsequently, to determine the number of bolls, the growth state of cotton was graded. Finally, combined with cotton boll weight, boll opening rate and other information, the cotton yield in the experimental area was estimated by SENP model, and the precision was verified according to the measured data of yield. The experimental results reveal that the U-Net model can effectively extract the information of cotton seedlings from the background with high accuracy. And the precision rate, recall rate and F value reached 93.88%, 97.87% and 95.83% respectively. NDVI based on time series can accurately reflect the growth state of cotton, so as to obtain the predicted boll number of each cotton, which greatly improves the accuracy and universality of the yield estimation model. The determination coefficient (R) of the yield estimation model reached 0.92, indicating that using SENP model for cotton yield estimation is an effective method. This study also proved that the potential and advantage of combining the AWS platform with SENP, due to its powerful cloud computing capacity, especially for deep learning, time-series crop monitoring and large scale yield estimation. This research can provide the reference information for cotton yield estimation and cloud computing platform application.