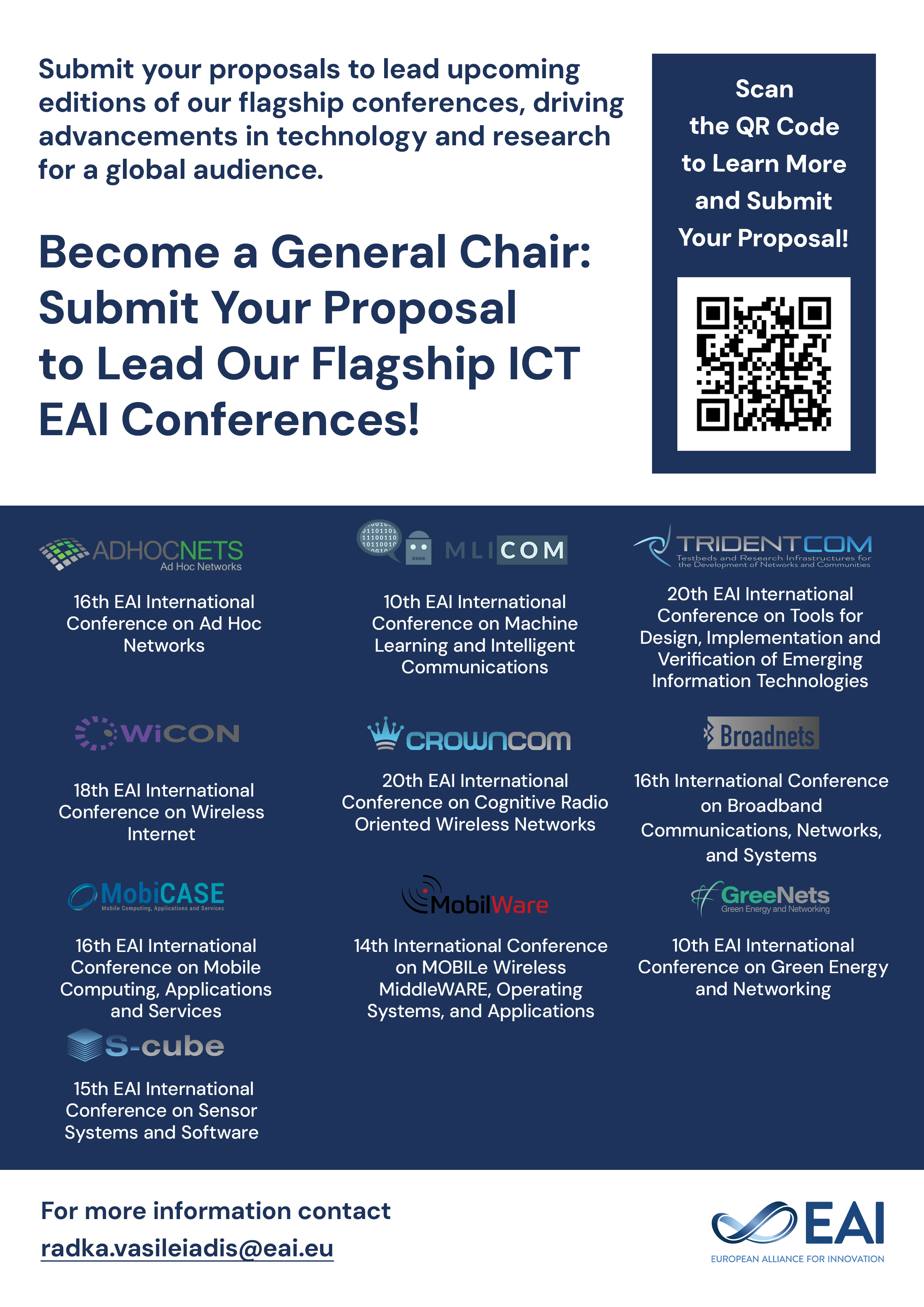
Research Article
Refactor Business Process Models for Efficiency Improvement
@INPROCEEDINGS{10.1007/978-3-030-48513-9_37, author={Fei Dai and Miao Liu and Qi Mo and Bi Huang and Tong Li}, title={Refactor Business Process Models for Efficiency Improvement}, proceedings={Cloud Computing, Smart Grid and Innovative Frontiers in Telecommunications. 9th EAI International Conference, CloudComp 2019, and 4th EAI International Conference, SmartGIFT 2019, Beijing, China, December 4-5, 2019, and December 21-22, 2019}, proceedings_a={CLOUDCOMP}, year={2020}, month={6}, keywords={Business process model Efficiency improvement Refactor Petri net}, doi={10.1007/978-3-030-48513-9_37} }
- Fei Dai
Miao Liu
Qi Mo
Bi Huang
Tong Li
Year: 2020
Refactor Business Process Models for Efficiency Improvement
CLOUDCOMP
Springer
DOI: 10.1007/978-3-030-48513-9_37
Abstract
Since business processes describe the core value chain of enterprises, thousands of business processes are modeled in business process models. A problem is how to improve the efficiency of these models. In this paper, we propose an approach to refactor these models for efficiency improvement. More specifically, we first identify false sequence relations that affect model efficiency based on the sequence relation matrix and the dependency relation matrix. Second, we refactor a business process model by constructing and transforming a dependency graph without altering its output result. After refactoring, the concurrent execution of business tasks in the original models can be maximized such that its efficiency can be improved. Experimental results show the effectiveness of our approach.