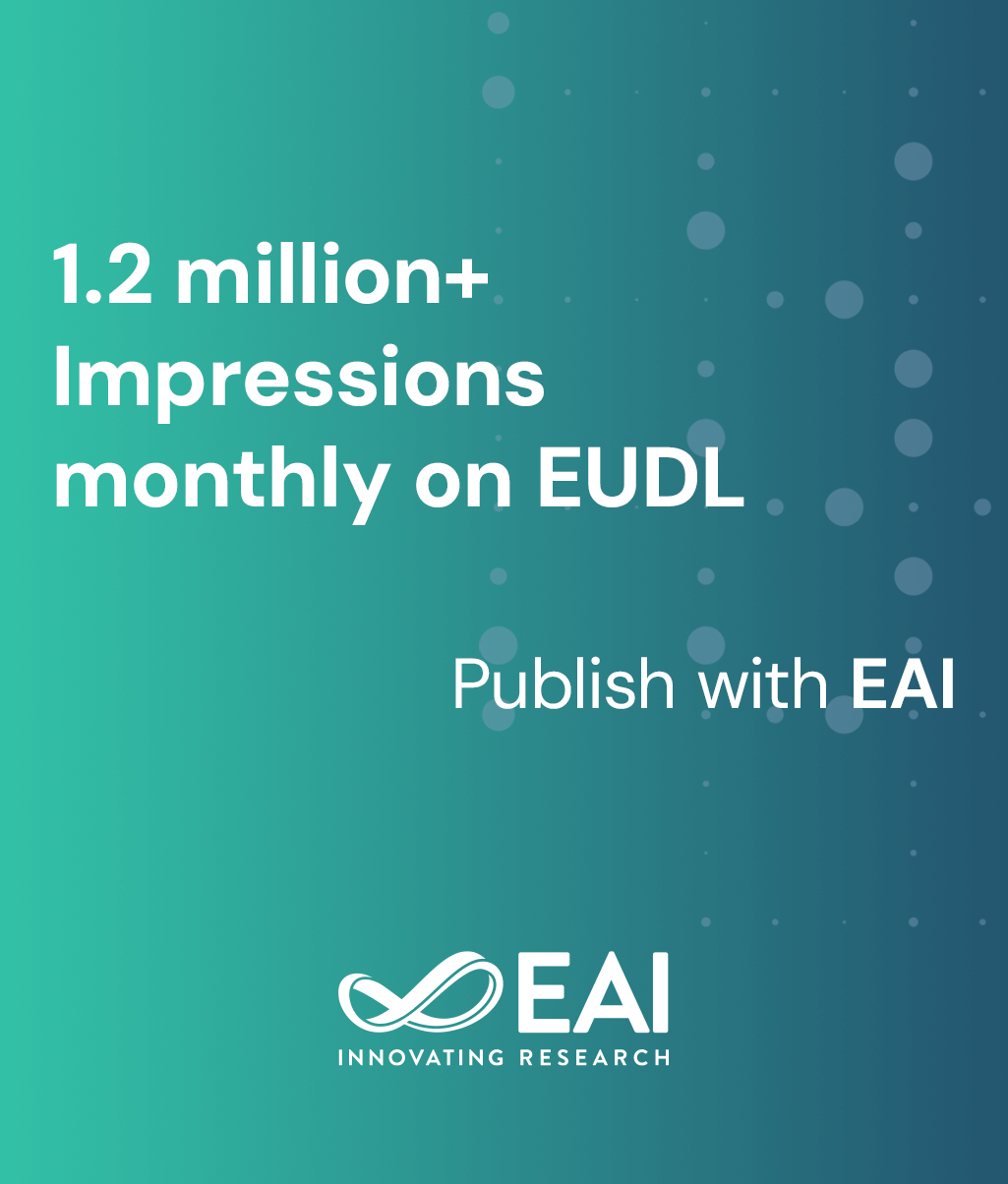
Research Article
A Semi-supervised Classification Method for Hyperspectral Images by Triple Classifiers with Data Editing and Deep Learning
@INPROCEEDINGS{10.1007/978-3-030-48513-9_14, author={Guoming Zhang and Junshu Wang and Ge Shi and Jie Zhang and Wanchun Dou}, title={A Semi-supervised Classification Method for Hyperspectral Images by Triple Classifiers with Data Editing and Deep Learning}, proceedings={Cloud Computing, Smart Grid and Innovative Frontiers in Telecommunications. 9th EAI International Conference, CloudComp 2019, and 4th EAI International Conference, SmartGIFT 2019, Beijing, China, December 4-5, 2019, and December 21-22, 2019}, proceedings_a={CLOUDCOMP}, year={2020}, month={6}, keywords={Deep learning Hyperspectral images (HSI) classification Tri-training Data editing}, doi={10.1007/978-3-030-48513-9_14} }
- Guoming Zhang
Junshu Wang
Ge Shi
Jie Zhang
Wanchun Dou
Year: 2020
A Semi-supervised Classification Method for Hyperspectral Images by Triple Classifiers with Data Editing and Deep Learning
CLOUDCOMP
Springer
DOI: 10.1007/978-3-030-48513-9_14
Abstract
A semi-supervised classification method for hyperspectral remote sensing images based on convolutional neural network (CNN) and modified tri-training is proposed. The abstract features are captured by training a CNN model with the pixels’ vectors as inputs. Based on the extracted high-level features, different classifiers will perform different outputs under the same training set, due to the different types of classifiers take on diverse characteristics. Thus, taking multiple classifiers’ results into consideration can integrate different prediction labels synthetically from a high level and can perform more credible results. At the meantime, the number of training samples of hyperspectral images is limited, which will hinder the classification effect. Illuminated by tri-training algorithm, we utilize triple different classifiers to classify the hyperspectral images based on the extracted high-level features in the semi-supervised mode. By utilizing triple classifiers jointly to train and update the training samples set when the number of labeled samples is limited. At the meantime, we pick the confident samples via randomize and majority vote into the training set for data editing during the iterative updating process. Experiments performed on two real hyperspectral images reveal that our method performs very well in terms of classification accuracy and effect.